AI/ML
AI/ML
AI/ML
Introducing Video-To-Text and Pegasus-1 (80B)


Aiden Lee, Jae Lee
Aiden Lee, Jae Lee
Aiden Lee, Jae Lee
This article introduces the suite of video-to-text APIs powered by our latest video-language foundation model, Pegasus-1.
This article introduces the suite of video-to-text APIs powered by our latest video-language foundation model, Pegasus-1.


Join our newsletter
Receive the latest advancements, tutorials, and industry insights in video understanding
Oct 23, 2023
Oct 23, 2023
Oct 23, 2023
8min
8min
8min
Copy link to article
Copy link to article
Copy link to article
Bullet Point Summary
Product: Twelve Labs is announcing their latest video-language foundation model Pegasus-1 along with a new suite of Video-to-Text APIs (Gist API, Summary API, Generate API).
Product and Research Philosophy: Unlike many that reframe video understanding as an image or speech understanding problem, Twelve Labs adopts a “Video First” strategy with four core principles: Efficient Long-form Video Processing, Multimodal Understanding, Video-native Embeddings, Deep Alignment between Video and Language Embeddings
The New Model: Pegasus-1 has approximately 80B parameters with three model components jointly trained together: video encoder, video-language alignment model, language decoder.
Dataset: Twelve Labs has collected over 300 million diverse, carefully-curated video-text pairs, making it one of the largest video-text corpora there is for video-language foundation model training. This technical report is based on the initial training run conducted on a 10% subset consisting of 35M video-text pairs and over 1B image-text pairs.
Performance against SOTA video-language model: Compared to the previous state-of-the-art (SOTA) video-language model, Pegasus-1 shows a 61% relative improvement on the MSR-VTT Dataset (Xu et al., 2016) and 47% enhancement on the Video Descriptions Dataset (Maaz et al., 2023) as measured by the QEFVC Quality Score (Maaz et al., 2023). Evaluating on VidFactScore (our proposed evaluation metric), it shows 20% absolute F1-score increase on the MSR-VTT Dataset and 14% enhancement on the Video Description dataset.
Performance against ASR+LLM models: ASR+LLM is a widely-adopted approach for tackling video-to-text tasks. Compared to Whisper-ChatGPT (OpenAI) and a leading commercial ASR+LLM product, Pegasus-1 outperforms by 79% on MSR-VTT and 188% on the Video Descriptions dataset. Evaluating on VidFactScore-F1, it shows 25% absolute gains on the MSR-VTT Dataset and 33% on the Video Description dataset.
API access to Pegasus-1: Here is the link for the waitlist for Pegasus-powered Video-to-Text APIs
Background
Expanding Our Research Horizon: Beyond Video Embedding to Generative Models

Twelve Labs, a San Francisco Bay Area-based AI research and product company, is at the forefront of multimodal video understanding. Today, we are thrilled to unveil the state-of-the-art video-to-text generation capabilities of Pegasus-1, our latest video-language foundation model. This represents our commitment to offer a comprehensive suite of APIs tailored for various downstream video understanding tasks. Our suite spans from natural language-based video moment retrieval to classification, and now, with the latest release, prompt-based video-to-text generation.
Our Video-First Ethos
Video data is intriguing as it contains multiple modalities within a single format. We believe that video understanding requires a novel take on marrying the intricacies of visual perception and the sequential and contextual nuances of audio and text. With the rise of capable image and language models, the dominant approach for video understanding has been to reframe it as an image or speech understanding problem. A typical framework would involve sampling frames from the video and inputting them into a vision-language model.
While this approach may be viable for short videos (which is why most vision-language models focus on < 1min video clips), most real-world videos exceed 1 minute and can easily extend to hours. Using a vanilla "image-first" approach on such videos would mean processing tens of thousands of images for each video, which result in having to manipulate on a vast number of image-text embeddings that loosely capture the semantics in spatiotemporal information at best. This is impractical in many applications in terms of performance, latency, and cost. Furthermore, the dominant methodology overlooks the multimodal nature of videos, wherein the joint analysis of both visual and auditory elements, including speech, is crucial for a comprehensive understanding of their content.
With the fundamental properties of video data in mind, Twelve Labs has adopted a “Video First” strategy, focusing our model, data, and ML systems solely to processing and understanding video data. This stands in contrast to the prevalent “Language/Image First” approach observed among many Generative AI players. Four central principles underscore our “Video First” ethos, guiding both the design of our video-language foundation models and the architecture of our ML system:
Efficient Long-form Video Processing: Our model and system must be optimized to manage videos of diverse lengths, from brief 10-second clips to extensive multi-hour content.
Multimodal Understanding: Our model must be able to synthesize visual, audio, and speech information.
Video-native Embeddings: Instead of relying on image-native embeddings (e.g. CLIP) that focus on spatial relationships, we believe in the need for video-native embeddings that can incorporate spatiotemporal information of a video in a holistic manner.
Deep Alignment Between Video-native Embeddings and Language Model: Beyond image-text alignment, our model must undergo deep video-language alignment through extensive training on large video-text corpora and video-text instruction datasets.
New Video-to-Text Capabilities and Interfaces
With a single API call, developers can prompt Pegasus-1 model to produce specific text outputs from their video data. Contrary to existing solutions that either utilizes speech-to-text conversions or rely solely on visual frame data, Pegasus-1 integrates visual, audio, and speech information to generate more holistic text from videos, achieving the new state-of-the-art performance in video summarization benchmarks. (See Evaluation and Results section below.)

The Gist and Summary APIs are pre-loaded with relevant prompts to work out of the box without needing user prompts. The Gist API can produce concise text outputs like titles, topics, and lists of relevant hashtags. The Summary API is designed to generate video summaries, chapters, and highlights. For customized outputs, the experimental Generate API allows users to prompt specific formats and styles, from bullet points to reports and even creative lyrics based on the content of the video.
Example 1: Generating a small report from a video through the Gist and Summary APIs.

Example 2: Generating a video summary by passing in a styling prompt to the Summary API.

Example 3: Generating a customized text output by prompting through the experimental Generate API.

Example 4: Demonstrating multimodal understanding that incorporates visual, speech, and auditory cues inside a video. (Highlighted in Green: Visual Information)

Pegasus-1 (80B) Model Overview

The Functions of the Constituent Models and the Overall Architecture
Pegasus-1 model is structured around three primary components, each tasked with generating video-native embeddings, video-language-aligned embeddings, and text outputs respectively.
1. Video Encoder Model - Derived from our existing Marengo embedding model
Input: Video
Output: Video Embeddings (incorporating visual, audio, and speech information)
Function: The purpose of the video encoder is to glean intricate details from videos. It assesses frames and their temporal relationships to obtain relevant visual information while concurrently processing audio signals and speech information.
2. Video-language Alignment Model
Input: Video Embeddings
Output: Video-language-aligned Embeddings
Function: The alignment model’s primary task is to bridge the video embeddings with the language model’s domain. This ensures that the language model interprets the video embeddings similarly how it comprehends text tokens.
3. Large Language Model - Decoder model
Input: Video-language-aligned Embedding, User prompt
Output: Text
Function: Leveraging its extensive knowledge base, the language model interprets the aligned embeddings based on the input user prompts. It then decodes this information into coherent, human-readable text.
Model Parameters and Scale
The Pegasus-1 model has an aggregate of approximately 80B parameters. Detailed parameter distributions for individual components, including the size of the Marengo embedding model, are not disclosed at this time.
Training and Fine-tuning Datasets
Training Data for Video-Language Foundation Model: From a collection of over 300M video-text pairs, we have processed and selected a 10% subset, consisting of 35M videos (termed TL-35M) and over 1B images. We believe this is sufficiently large as a first training run and will run the subsequent training on TL-100M. To the best of our knowledge, this is the largest video-text corpus there is that is carefully curated for training video-language foundation models. To support broader research efforts, we contemplate open-sourcing a smaller dataset. For expressions of interest, please contact research@twelvelabs.io.
Fine-tuning Dataset: A high-quality video-to-text fine-tuning dataset is crucial for enhancing the instruction-following capability of the aforementioned video-language foundation model. Our selection criteria revolve around three primary aspects: diversity of domains, comprehensiveness and precision in text annotation. On average, the text annotation associated with each video in our dataset is twice as long as those in existing open datasets for videos of similar length. Furthermore, annotations undergo several rounds of verification and correction to ensure accuracy. While this approach elevates the unit annotation cost, we prioritized on maintaining high standards in the quality of the fine-tuning dataset over merely increasing its size, as the importance observed in the previous work (Zhou et al., 2023).
Factors Influencing Performance
As one would expect, the overall model performance is strongly correlated with that of each component. The degree of how much each constituent model influences the overall quality remains an open question. We will be conducting an extensive ablation study to have a better understanding and share our findings in the future.
Video Encoder Model: Derived from our Marengo 2.5 model (March 2023, 100M+ Videos / 1B+ Images) which powers our current Search and Classify APIs, video encoder model achieves state-of-the-art results in embedding-based tasks such as video classification and retrieval. The depth of the information extractable from a video is inherently upper-bounded by the video encoder model. Further details on the Marengo model will be featured in an upcoming report with the next release of Marengo 2.6.
Video-language Alignment Model: This model acquires its video-language alignment proficiency during foundation model training and instruction fine-tuning. The extent to which our language model can interface with video embeddings is demarcated by this alignment mechanism.
Large Language Model (Decoder Model): Our language model’s capabilities are framed by its knowledge acquired during its training phase. The caliber of the resulting text output is governed by the model’s ability to synthesize its knowledge, user prompts, and the video-language-aligned embeddings.
Evaluation and Results
Twelve Labs acknowledges the importance of ensuring the responsible deployment of advanced technologies, including our Pegasus-1 model. We are dedicated to developing a comprehensive and transparent dataset and evaluation framework that benchmarks all of our models across fine-grained categories including correctness, detail orientation, contextual understanding, safety, and helpfulness. We are in the process of developing metrics specifically for safety and helpfulness in video-language models, with results to be shared imminently. We are excited to present the preliminary findings in this blog with a more detailed report to be released in the future. The evaluation is based on the preview version of Pegasus-1.
Our evaluation codebase can be found here.
Comparison Models
We compare our model against three distinct model (or product) categories:
Video-ChatGPT (Maaz et al., 2023): This open-source model is the current state-of-the-art video language model with a chat interface. The model processes video frames to capture visual events within a video. However, it does not utilize the conversational information within the video.
Whisper + ChatGPT-3.5 (OpenAI): This combination is one of the few widely adopted approaches to video summarization. By leveraging the state-of-the-art speech-to-text and large language models, summaries are derived primarily from the video’s auditory content. A significant drawback is the oversight of valuable visual information within the video.
Vendor A’s Summary API: Widely adopted commercial product for audio and video summary generation. Vendor A’s Summary API seems to be based only on transcription data and language models (akin to ASR+ChatGPT3.5) to output a video summary.
Datasets
MSR-VTT Dataset (Xu et al., 2016): MSR-VTT is a widely used video-caption dataset that assesses a model's ability to generate a description or caption for short video clips ranging from 10 to 40 seconds. Each video is annotated with 20 sentences by human annotators. To capture as much detail as possible, we use an LLM (ChatGPT) to merge the 20 individual captions into a single, dense description. Our evaluation is conducted on the JSFusion Test Split, which consists of 1,000 video clips.
Video-ChatGPT Video Descriptions Dataset (Maaz et al., 2023): Video-to-text evaluations predominantly use video captioning datasets datasets, such as MSR-VTT dataset (above). While these short video descriptions offer a point of reference, they fall short in evaluating text generation for long-form videos prevalent in real-world contexts. Given this, we conduct an additional evaluation on our model on Video-ChatGPT Video Description Dataset. This dataset includes 500 videos from ActivityNet, all accompanied by comprehensive human-annotated summaries. Unlike traditional captioning datasets, the videos in this dataset range from 30 seconds to several minutes, and each comes with a dense, 5-8 sentence summary encapsulating both visual and auditory elements.
Metrics
Following the Quantitative Evaluation Framework for Video-based Conversation Models (QEFVC)(Maaz et al., 2023), we evaluate the models on three areas: Correctness of Information, Detail Orientation, and Contextual Understanding. To do this, we ask an instruction-tuned language model (e.g. GPT-4) about each criterion with respect to the reference summary. To quantify overall performance, we average the three scores, defining it as QEFVC quality score.
While this evaluation metric offers a convenient means for comparison with existing models, they are not without challenges. Past works on language model evaluation have highlighted that solely relying on GPT-4 for model prediction scores is prone to inaccurate evaluation. It is also observed that making the evaluation as fine-grained as possible improves the consistency as well as the precision of the evaluation (Ye et al., 2023). With this in mind, and drawing inspiration from FActScore (Min et al., 2023), we introduce a refined evaluation method, VidFactScore (Video Factual Score) to evaluate the quality of video summaries in a much more fine-grained manner:
For each video and reference summary pair, we break down the reference summary into discrete facts. As an example, “A man and a woman are running.” is segmented into “A man is running.”, and “A woman is running.”. This segmentation is done by an instruction-tuned language model, like GPT-4, with appropriate prompting.
The model-generated summary undergoes a similar division.
An ideal predicted summary should (1) encompass the majority of facts from the reference and (2) minimize the inclusion of facts absent in the reference. Determining the presence or absence of facts is achieved through an instruction-tuned language model with appropriate prompting.
From a quantitative perspective, (1) corresponds to Recall Rate, which calculates the proportion of shared facts between prediction and reference to the total facts in the reference. (2) corresponds to Precision, which calculates the proportion of shared facts to the total facts in the prediction. The harmonic mean of the two numbers, F1, offers a straightforward metric for model comparison.
Results


In a comparison with the current state-of-the-art model (VideoChatGPT), Pegasus-1 exhibits a significant 61% relative improvement on the MSR-VTT dataset and 47% enhancement on the Video Description dataset as measured by the QEFVC Quality Score. Against the ASR+LLM model cohort (including models such as Whisper+ChatGPT and Vendor A), the performance gap widens with Pegasus-1 outperforming by 79% on MSR-VTT and 188% on the Video Description dataset.


Evaluating on our newly proposed VidFactScore-F1 metric, Pegasus-1 shows a 20% absolute increase on the MSR-VTT Dataset and 14% enhancement on the Video Descriptions dataset compared to VideoChatGPT. When benchmarked against the ASR+LLM model cohort, the gains are 25% on the MSR-VTT Dataset and 33% on the Video Descriptions dataset. These findings consistently suggest that VidFactScore aligns well and correlates strongly with evaluations based on the QEFVC framework.
An intriguing observation was made regarding videos predominantly reliant on speech, such as standup routines or lectures. Our model outperforms the ASR+LLM models even in these scenarios. Contrary to initial perceptions that ASR might suffice for such videos, our findings suggest otherwise. We postulate that even minimal visual cues (for instance, "a man performing standup comedy" or “a reaction video”) can enrich speech data, yielding more precise and encompassing summaries. Such outcomes underscore the notion that video comprehension surpasses mere speech understanding. It is clear that incorporating both visual and speech modalities is necessary to achieve a comprehensive understanding. See the “Reaction Video” in the “In-the-wild Examples” section below.
In-the-wild examples
These are sample examples that are randomly selected from diverse domains to illustrate the capabilities of Pegasus-1 compared to the existing approaches.
Note that the generated output may contain
Hallucinations (creating a coherent story even though it is not factually depicted in the video)
Irrelevant answers due to not being able to comprehend the prompt or question
Biases
We welcome any feedback and will do our best to address them in the near future.
Bullet Point Summary
Product: Twelve Labs is announcing their latest video-language foundation model Pegasus-1 along with a new suite of Video-to-Text APIs (Gist API, Summary API, Generate API).
Product and Research Philosophy: Unlike many that reframe video understanding as an image or speech understanding problem, Twelve Labs adopts a “Video First” strategy with four core principles: Efficient Long-form Video Processing, Multimodal Understanding, Video-native Embeddings, Deep Alignment between Video and Language Embeddings
The New Model: Pegasus-1 has approximately 80B parameters with three model components jointly trained together: video encoder, video-language alignment model, language decoder.
Dataset: Twelve Labs has collected over 300 million diverse, carefully-curated video-text pairs, making it one of the largest video-text corpora there is for video-language foundation model training. This technical report is based on the initial training run conducted on a 10% subset consisting of 35M video-text pairs and over 1B image-text pairs.
Performance against SOTA video-language model: Compared to the previous state-of-the-art (SOTA) video-language model, Pegasus-1 shows a 61% relative improvement on the MSR-VTT Dataset (Xu et al., 2016) and 47% enhancement on the Video Descriptions Dataset (Maaz et al., 2023) as measured by the QEFVC Quality Score (Maaz et al., 2023). Evaluating on VidFactScore (our proposed evaluation metric), it shows 20% absolute F1-score increase on the MSR-VTT Dataset and 14% enhancement on the Video Description dataset.
Performance against ASR+LLM models: ASR+LLM is a widely-adopted approach for tackling video-to-text tasks. Compared to Whisper-ChatGPT (OpenAI) and a leading commercial ASR+LLM product, Pegasus-1 outperforms by 79% on MSR-VTT and 188% on the Video Descriptions dataset. Evaluating on VidFactScore-F1, it shows 25% absolute gains on the MSR-VTT Dataset and 33% on the Video Description dataset.
API access to Pegasus-1: Here is the link for the waitlist for Pegasus-powered Video-to-Text APIs
Background
Expanding Our Research Horizon: Beyond Video Embedding to Generative Models

Twelve Labs, a San Francisco Bay Area-based AI research and product company, is at the forefront of multimodal video understanding. Today, we are thrilled to unveil the state-of-the-art video-to-text generation capabilities of Pegasus-1, our latest video-language foundation model. This represents our commitment to offer a comprehensive suite of APIs tailored for various downstream video understanding tasks. Our suite spans from natural language-based video moment retrieval to classification, and now, with the latest release, prompt-based video-to-text generation.
Our Video-First Ethos
Video data is intriguing as it contains multiple modalities within a single format. We believe that video understanding requires a novel take on marrying the intricacies of visual perception and the sequential and contextual nuances of audio and text. With the rise of capable image and language models, the dominant approach for video understanding has been to reframe it as an image or speech understanding problem. A typical framework would involve sampling frames from the video and inputting them into a vision-language model.
While this approach may be viable for short videos (which is why most vision-language models focus on < 1min video clips), most real-world videos exceed 1 minute and can easily extend to hours. Using a vanilla "image-first" approach on such videos would mean processing tens of thousands of images for each video, which result in having to manipulate on a vast number of image-text embeddings that loosely capture the semantics in spatiotemporal information at best. This is impractical in many applications in terms of performance, latency, and cost. Furthermore, the dominant methodology overlooks the multimodal nature of videos, wherein the joint analysis of both visual and auditory elements, including speech, is crucial for a comprehensive understanding of their content.
With the fundamental properties of video data in mind, Twelve Labs has adopted a “Video First” strategy, focusing our model, data, and ML systems solely to processing and understanding video data. This stands in contrast to the prevalent “Language/Image First” approach observed among many Generative AI players. Four central principles underscore our “Video First” ethos, guiding both the design of our video-language foundation models and the architecture of our ML system:
Efficient Long-form Video Processing: Our model and system must be optimized to manage videos of diverse lengths, from brief 10-second clips to extensive multi-hour content.
Multimodal Understanding: Our model must be able to synthesize visual, audio, and speech information.
Video-native Embeddings: Instead of relying on image-native embeddings (e.g. CLIP) that focus on spatial relationships, we believe in the need for video-native embeddings that can incorporate spatiotemporal information of a video in a holistic manner.
Deep Alignment Between Video-native Embeddings and Language Model: Beyond image-text alignment, our model must undergo deep video-language alignment through extensive training on large video-text corpora and video-text instruction datasets.
New Video-to-Text Capabilities and Interfaces
With a single API call, developers can prompt Pegasus-1 model to produce specific text outputs from their video data. Contrary to existing solutions that either utilizes speech-to-text conversions or rely solely on visual frame data, Pegasus-1 integrates visual, audio, and speech information to generate more holistic text from videos, achieving the new state-of-the-art performance in video summarization benchmarks. (See Evaluation and Results section below.)

The Gist and Summary APIs are pre-loaded with relevant prompts to work out of the box without needing user prompts. The Gist API can produce concise text outputs like titles, topics, and lists of relevant hashtags. The Summary API is designed to generate video summaries, chapters, and highlights. For customized outputs, the experimental Generate API allows users to prompt specific formats and styles, from bullet points to reports and even creative lyrics based on the content of the video.
Example 1: Generating a small report from a video through the Gist and Summary APIs.

Example 2: Generating a video summary by passing in a styling prompt to the Summary API.

Example 3: Generating a customized text output by prompting through the experimental Generate API.

Example 4: Demonstrating multimodal understanding that incorporates visual, speech, and auditory cues inside a video. (Highlighted in Green: Visual Information)

Pegasus-1 (80B) Model Overview

The Functions of the Constituent Models and the Overall Architecture
Pegasus-1 model is structured around three primary components, each tasked with generating video-native embeddings, video-language-aligned embeddings, and text outputs respectively.
1. Video Encoder Model - Derived from our existing Marengo embedding model
Input: Video
Output: Video Embeddings (incorporating visual, audio, and speech information)
Function: The purpose of the video encoder is to glean intricate details from videos. It assesses frames and their temporal relationships to obtain relevant visual information while concurrently processing audio signals and speech information.
2. Video-language Alignment Model
Input: Video Embeddings
Output: Video-language-aligned Embeddings
Function: The alignment model’s primary task is to bridge the video embeddings with the language model’s domain. This ensures that the language model interprets the video embeddings similarly how it comprehends text tokens.
3. Large Language Model - Decoder model
Input: Video-language-aligned Embedding, User prompt
Output: Text
Function: Leveraging its extensive knowledge base, the language model interprets the aligned embeddings based on the input user prompts. It then decodes this information into coherent, human-readable text.
Model Parameters and Scale
The Pegasus-1 model has an aggregate of approximately 80B parameters. Detailed parameter distributions for individual components, including the size of the Marengo embedding model, are not disclosed at this time.
Training and Fine-tuning Datasets
Training Data for Video-Language Foundation Model: From a collection of over 300M video-text pairs, we have processed and selected a 10% subset, consisting of 35M videos (termed TL-35M) and over 1B images. We believe this is sufficiently large as a first training run and will run the subsequent training on TL-100M. To the best of our knowledge, this is the largest video-text corpus there is that is carefully curated for training video-language foundation models. To support broader research efforts, we contemplate open-sourcing a smaller dataset. For expressions of interest, please contact research@twelvelabs.io.
Fine-tuning Dataset: A high-quality video-to-text fine-tuning dataset is crucial for enhancing the instruction-following capability of the aforementioned video-language foundation model. Our selection criteria revolve around three primary aspects: diversity of domains, comprehensiveness and precision in text annotation. On average, the text annotation associated with each video in our dataset is twice as long as those in existing open datasets for videos of similar length. Furthermore, annotations undergo several rounds of verification and correction to ensure accuracy. While this approach elevates the unit annotation cost, we prioritized on maintaining high standards in the quality of the fine-tuning dataset over merely increasing its size, as the importance observed in the previous work (Zhou et al., 2023).
Factors Influencing Performance
As one would expect, the overall model performance is strongly correlated with that of each component. The degree of how much each constituent model influences the overall quality remains an open question. We will be conducting an extensive ablation study to have a better understanding and share our findings in the future.
Video Encoder Model: Derived from our Marengo 2.5 model (March 2023, 100M+ Videos / 1B+ Images) which powers our current Search and Classify APIs, video encoder model achieves state-of-the-art results in embedding-based tasks such as video classification and retrieval. The depth of the information extractable from a video is inherently upper-bounded by the video encoder model. Further details on the Marengo model will be featured in an upcoming report with the next release of Marengo 2.6.
Video-language Alignment Model: This model acquires its video-language alignment proficiency during foundation model training and instruction fine-tuning. The extent to which our language model can interface with video embeddings is demarcated by this alignment mechanism.
Large Language Model (Decoder Model): Our language model’s capabilities are framed by its knowledge acquired during its training phase. The caliber of the resulting text output is governed by the model’s ability to synthesize its knowledge, user prompts, and the video-language-aligned embeddings.
Evaluation and Results
Twelve Labs acknowledges the importance of ensuring the responsible deployment of advanced technologies, including our Pegasus-1 model. We are dedicated to developing a comprehensive and transparent dataset and evaluation framework that benchmarks all of our models across fine-grained categories including correctness, detail orientation, contextual understanding, safety, and helpfulness. We are in the process of developing metrics specifically for safety and helpfulness in video-language models, with results to be shared imminently. We are excited to present the preliminary findings in this blog with a more detailed report to be released in the future. The evaluation is based on the preview version of Pegasus-1.
Our evaluation codebase can be found here.
Comparison Models
We compare our model against three distinct model (or product) categories:
Video-ChatGPT (Maaz et al., 2023): This open-source model is the current state-of-the-art video language model with a chat interface. The model processes video frames to capture visual events within a video. However, it does not utilize the conversational information within the video.
Whisper + ChatGPT-3.5 (OpenAI): This combination is one of the few widely adopted approaches to video summarization. By leveraging the state-of-the-art speech-to-text and large language models, summaries are derived primarily from the video’s auditory content. A significant drawback is the oversight of valuable visual information within the video.
Vendor A’s Summary API: Widely adopted commercial product for audio and video summary generation. Vendor A’s Summary API seems to be based only on transcription data and language models (akin to ASR+ChatGPT3.5) to output a video summary.
Datasets
MSR-VTT Dataset (Xu et al., 2016): MSR-VTT is a widely used video-caption dataset that assesses a model's ability to generate a description or caption for short video clips ranging from 10 to 40 seconds. Each video is annotated with 20 sentences by human annotators. To capture as much detail as possible, we use an LLM (ChatGPT) to merge the 20 individual captions into a single, dense description. Our evaluation is conducted on the JSFusion Test Split, which consists of 1,000 video clips.
Video-ChatGPT Video Descriptions Dataset (Maaz et al., 2023): Video-to-text evaluations predominantly use video captioning datasets datasets, such as MSR-VTT dataset (above). While these short video descriptions offer a point of reference, they fall short in evaluating text generation for long-form videos prevalent in real-world contexts. Given this, we conduct an additional evaluation on our model on Video-ChatGPT Video Description Dataset. This dataset includes 500 videos from ActivityNet, all accompanied by comprehensive human-annotated summaries. Unlike traditional captioning datasets, the videos in this dataset range from 30 seconds to several minutes, and each comes with a dense, 5-8 sentence summary encapsulating both visual and auditory elements.
Metrics
Following the Quantitative Evaluation Framework for Video-based Conversation Models (QEFVC)(Maaz et al., 2023), we evaluate the models on three areas: Correctness of Information, Detail Orientation, and Contextual Understanding. To do this, we ask an instruction-tuned language model (e.g. GPT-4) about each criterion with respect to the reference summary. To quantify overall performance, we average the three scores, defining it as QEFVC quality score.
While this evaluation metric offers a convenient means for comparison with existing models, they are not without challenges. Past works on language model evaluation have highlighted that solely relying on GPT-4 for model prediction scores is prone to inaccurate evaluation. It is also observed that making the evaluation as fine-grained as possible improves the consistency as well as the precision of the evaluation (Ye et al., 2023). With this in mind, and drawing inspiration from FActScore (Min et al., 2023), we introduce a refined evaluation method, VidFactScore (Video Factual Score) to evaluate the quality of video summaries in a much more fine-grained manner:
For each video and reference summary pair, we break down the reference summary into discrete facts. As an example, “A man and a woman are running.” is segmented into “A man is running.”, and “A woman is running.”. This segmentation is done by an instruction-tuned language model, like GPT-4, with appropriate prompting.
The model-generated summary undergoes a similar division.
An ideal predicted summary should (1) encompass the majority of facts from the reference and (2) minimize the inclusion of facts absent in the reference. Determining the presence or absence of facts is achieved through an instruction-tuned language model with appropriate prompting.
From a quantitative perspective, (1) corresponds to Recall Rate, which calculates the proportion of shared facts between prediction and reference to the total facts in the reference. (2) corresponds to Precision, which calculates the proportion of shared facts to the total facts in the prediction. The harmonic mean of the two numbers, F1, offers a straightforward metric for model comparison.
Results


In a comparison with the current state-of-the-art model (VideoChatGPT), Pegasus-1 exhibits a significant 61% relative improvement on the MSR-VTT dataset and 47% enhancement on the Video Description dataset as measured by the QEFVC Quality Score. Against the ASR+LLM model cohort (including models such as Whisper+ChatGPT and Vendor A), the performance gap widens with Pegasus-1 outperforming by 79% on MSR-VTT and 188% on the Video Description dataset.


Evaluating on our newly proposed VidFactScore-F1 metric, Pegasus-1 shows a 20% absolute increase on the MSR-VTT Dataset and 14% enhancement on the Video Descriptions dataset compared to VideoChatGPT. When benchmarked against the ASR+LLM model cohort, the gains are 25% on the MSR-VTT Dataset and 33% on the Video Descriptions dataset. These findings consistently suggest that VidFactScore aligns well and correlates strongly with evaluations based on the QEFVC framework.
An intriguing observation was made regarding videos predominantly reliant on speech, such as standup routines or lectures. Our model outperforms the ASR+LLM models even in these scenarios. Contrary to initial perceptions that ASR might suffice for such videos, our findings suggest otherwise. We postulate that even minimal visual cues (for instance, "a man performing standup comedy" or “a reaction video”) can enrich speech data, yielding more precise and encompassing summaries. Such outcomes underscore the notion that video comprehension surpasses mere speech understanding. It is clear that incorporating both visual and speech modalities is necessary to achieve a comprehensive understanding. See the “Reaction Video” in the “In-the-wild Examples” section below.
In-the-wild examples
These are sample examples that are randomly selected from diverse domains to illustrate the capabilities of Pegasus-1 compared to the existing approaches.
Note that the generated output may contain
Hallucinations (creating a coherent story even though it is not factually depicted in the video)
Irrelevant answers due to not being able to comprehend the prompt or question
Biases
We welcome any feedback and will do our best to address them in the near future.
Bullet Point Summary
Product: Twelve Labs is announcing their latest video-language foundation model Pegasus-1 along with a new suite of Video-to-Text APIs (Gist API, Summary API, Generate API).
Product and Research Philosophy: Unlike many that reframe video understanding as an image or speech understanding problem, Twelve Labs adopts a “Video First” strategy with four core principles: Efficient Long-form Video Processing, Multimodal Understanding, Video-native Embeddings, Deep Alignment between Video and Language Embeddings
The New Model: Pegasus-1 has approximately 80B parameters with three model components jointly trained together: video encoder, video-language alignment model, language decoder.
Dataset: Twelve Labs has collected over 300 million diverse, carefully-curated video-text pairs, making it one of the largest video-text corpora there is for video-language foundation model training. This technical report is based on the initial training run conducted on a 10% subset consisting of 35M video-text pairs and over 1B image-text pairs.
Performance against SOTA video-language model: Compared to the previous state-of-the-art (SOTA) video-language model, Pegasus-1 shows a 61% relative improvement on the MSR-VTT Dataset (Xu et al., 2016) and 47% enhancement on the Video Descriptions Dataset (Maaz et al., 2023) as measured by the QEFVC Quality Score (Maaz et al., 2023). Evaluating on VidFactScore (our proposed evaluation metric), it shows 20% absolute F1-score increase on the MSR-VTT Dataset and 14% enhancement on the Video Description dataset.
Performance against ASR+LLM models: ASR+LLM is a widely-adopted approach for tackling video-to-text tasks. Compared to Whisper-ChatGPT (OpenAI) and a leading commercial ASR+LLM product, Pegasus-1 outperforms by 79% on MSR-VTT and 188% on the Video Descriptions dataset. Evaluating on VidFactScore-F1, it shows 25% absolute gains on the MSR-VTT Dataset and 33% on the Video Description dataset.
API access to Pegasus-1: Here is the link for the waitlist for Pegasus-powered Video-to-Text APIs
Background
Expanding Our Research Horizon: Beyond Video Embedding to Generative Models

Twelve Labs, a San Francisco Bay Area-based AI research and product company, is at the forefront of multimodal video understanding. Today, we are thrilled to unveil the state-of-the-art video-to-text generation capabilities of Pegasus-1, our latest video-language foundation model. This represents our commitment to offer a comprehensive suite of APIs tailored for various downstream video understanding tasks. Our suite spans from natural language-based video moment retrieval to classification, and now, with the latest release, prompt-based video-to-text generation.
Our Video-First Ethos
Video data is intriguing as it contains multiple modalities within a single format. We believe that video understanding requires a novel take on marrying the intricacies of visual perception and the sequential and contextual nuances of audio and text. With the rise of capable image and language models, the dominant approach for video understanding has been to reframe it as an image or speech understanding problem. A typical framework would involve sampling frames from the video and inputting them into a vision-language model.
While this approach may be viable for short videos (which is why most vision-language models focus on < 1min video clips), most real-world videos exceed 1 minute and can easily extend to hours. Using a vanilla "image-first" approach on such videos would mean processing tens of thousands of images for each video, which result in having to manipulate on a vast number of image-text embeddings that loosely capture the semantics in spatiotemporal information at best. This is impractical in many applications in terms of performance, latency, and cost. Furthermore, the dominant methodology overlooks the multimodal nature of videos, wherein the joint analysis of both visual and auditory elements, including speech, is crucial for a comprehensive understanding of their content.
With the fundamental properties of video data in mind, Twelve Labs has adopted a “Video First” strategy, focusing our model, data, and ML systems solely to processing and understanding video data. This stands in contrast to the prevalent “Language/Image First” approach observed among many Generative AI players. Four central principles underscore our “Video First” ethos, guiding both the design of our video-language foundation models and the architecture of our ML system:
Efficient Long-form Video Processing: Our model and system must be optimized to manage videos of diverse lengths, from brief 10-second clips to extensive multi-hour content.
Multimodal Understanding: Our model must be able to synthesize visual, audio, and speech information.
Video-native Embeddings: Instead of relying on image-native embeddings (e.g. CLIP) that focus on spatial relationships, we believe in the need for video-native embeddings that can incorporate spatiotemporal information of a video in a holistic manner.
Deep Alignment Between Video-native Embeddings and Language Model: Beyond image-text alignment, our model must undergo deep video-language alignment through extensive training on large video-text corpora and video-text instruction datasets.
New Video-to-Text Capabilities and Interfaces
With a single API call, developers can prompt Pegasus-1 model to produce specific text outputs from their video data. Contrary to existing solutions that either utilizes speech-to-text conversions or rely solely on visual frame data, Pegasus-1 integrates visual, audio, and speech information to generate more holistic text from videos, achieving the new state-of-the-art performance in video summarization benchmarks. (See Evaluation and Results section below.)

The Gist and Summary APIs are pre-loaded with relevant prompts to work out of the box without needing user prompts. The Gist API can produce concise text outputs like titles, topics, and lists of relevant hashtags. The Summary API is designed to generate video summaries, chapters, and highlights. For customized outputs, the experimental Generate API allows users to prompt specific formats and styles, from bullet points to reports and even creative lyrics based on the content of the video.
Example 1: Generating a small report from a video through the Gist and Summary APIs.

Example 2: Generating a video summary by passing in a styling prompt to the Summary API.

Example 3: Generating a customized text output by prompting through the experimental Generate API.

Example 4: Demonstrating multimodal understanding that incorporates visual, speech, and auditory cues inside a video. (Highlighted in Green: Visual Information)

Pegasus-1 (80B) Model Overview

The Functions of the Constituent Models and the Overall Architecture
Pegasus-1 model is structured around three primary components, each tasked with generating video-native embeddings, video-language-aligned embeddings, and text outputs respectively.
1. Video Encoder Model - Derived from our existing Marengo embedding model
Input: Video
Output: Video Embeddings (incorporating visual, audio, and speech information)
Function: The purpose of the video encoder is to glean intricate details from videos. It assesses frames and their temporal relationships to obtain relevant visual information while concurrently processing audio signals and speech information.
2. Video-language Alignment Model
Input: Video Embeddings
Output: Video-language-aligned Embeddings
Function: The alignment model’s primary task is to bridge the video embeddings with the language model’s domain. This ensures that the language model interprets the video embeddings similarly how it comprehends text tokens.
3. Large Language Model - Decoder model
Input: Video-language-aligned Embedding, User prompt
Output: Text
Function: Leveraging its extensive knowledge base, the language model interprets the aligned embeddings based on the input user prompts. It then decodes this information into coherent, human-readable text.
Model Parameters and Scale
The Pegasus-1 model has an aggregate of approximately 80B parameters. Detailed parameter distributions for individual components, including the size of the Marengo embedding model, are not disclosed at this time.
Training and Fine-tuning Datasets
Training Data for Video-Language Foundation Model: From a collection of over 300M video-text pairs, we have processed and selected a 10% subset, consisting of 35M videos (termed TL-35M) and over 1B images. We believe this is sufficiently large as a first training run and will run the subsequent training on TL-100M. To the best of our knowledge, this is the largest video-text corpus there is that is carefully curated for training video-language foundation models. To support broader research efforts, we contemplate open-sourcing a smaller dataset. For expressions of interest, please contact research@twelvelabs.io.
Fine-tuning Dataset: A high-quality video-to-text fine-tuning dataset is crucial for enhancing the instruction-following capability of the aforementioned video-language foundation model. Our selection criteria revolve around three primary aspects: diversity of domains, comprehensiveness and precision in text annotation. On average, the text annotation associated with each video in our dataset is twice as long as those in existing open datasets for videos of similar length. Furthermore, annotations undergo several rounds of verification and correction to ensure accuracy. While this approach elevates the unit annotation cost, we prioritized on maintaining high standards in the quality of the fine-tuning dataset over merely increasing its size, as the importance observed in the previous work (Zhou et al., 2023).
Factors Influencing Performance
As one would expect, the overall model performance is strongly correlated with that of each component. The degree of how much each constituent model influences the overall quality remains an open question. We will be conducting an extensive ablation study to have a better understanding and share our findings in the future.
Video Encoder Model: Derived from our Marengo 2.5 model (March 2023, 100M+ Videos / 1B+ Images) which powers our current Search and Classify APIs, video encoder model achieves state-of-the-art results in embedding-based tasks such as video classification and retrieval. The depth of the information extractable from a video is inherently upper-bounded by the video encoder model. Further details on the Marengo model will be featured in an upcoming report with the next release of Marengo 2.6.
Video-language Alignment Model: This model acquires its video-language alignment proficiency during foundation model training and instruction fine-tuning. The extent to which our language model can interface with video embeddings is demarcated by this alignment mechanism.
Large Language Model (Decoder Model): Our language model’s capabilities are framed by its knowledge acquired during its training phase. The caliber of the resulting text output is governed by the model’s ability to synthesize its knowledge, user prompts, and the video-language-aligned embeddings.
Evaluation and Results
Twelve Labs acknowledges the importance of ensuring the responsible deployment of advanced technologies, including our Pegasus-1 model. We are dedicated to developing a comprehensive and transparent dataset and evaluation framework that benchmarks all of our models across fine-grained categories including correctness, detail orientation, contextual understanding, safety, and helpfulness. We are in the process of developing metrics specifically for safety and helpfulness in video-language models, with results to be shared imminently. We are excited to present the preliminary findings in this blog with a more detailed report to be released in the future. The evaluation is based on the preview version of Pegasus-1.
Our evaluation codebase can be found here.
Comparison Models
We compare our model against three distinct model (or product) categories:
Video-ChatGPT (Maaz et al., 2023): This open-source model is the current state-of-the-art video language model with a chat interface. The model processes video frames to capture visual events within a video. However, it does not utilize the conversational information within the video.
Whisper + ChatGPT-3.5 (OpenAI): This combination is one of the few widely adopted approaches to video summarization. By leveraging the state-of-the-art speech-to-text and large language models, summaries are derived primarily from the video’s auditory content. A significant drawback is the oversight of valuable visual information within the video.
Vendor A’s Summary API: Widely adopted commercial product for audio and video summary generation. Vendor A’s Summary API seems to be based only on transcription data and language models (akin to ASR+ChatGPT3.5) to output a video summary.
Datasets
MSR-VTT Dataset (Xu et al., 2016): MSR-VTT is a widely used video-caption dataset that assesses a model's ability to generate a description or caption for short video clips ranging from 10 to 40 seconds. Each video is annotated with 20 sentences by human annotators. To capture as much detail as possible, we use an LLM (ChatGPT) to merge the 20 individual captions into a single, dense description. Our evaluation is conducted on the JSFusion Test Split, which consists of 1,000 video clips.
Video-ChatGPT Video Descriptions Dataset (Maaz et al., 2023): Video-to-text evaluations predominantly use video captioning datasets datasets, such as MSR-VTT dataset (above). While these short video descriptions offer a point of reference, they fall short in evaluating text generation for long-form videos prevalent in real-world contexts. Given this, we conduct an additional evaluation on our model on Video-ChatGPT Video Description Dataset. This dataset includes 500 videos from ActivityNet, all accompanied by comprehensive human-annotated summaries. Unlike traditional captioning datasets, the videos in this dataset range from 30 seconds to several minutes, and each comes with a dense, 5-8 sentence summary encapsulating both visual and auditory elements.
Metrics
Following the Quantitative Evaluation Framework for Video-based Conversation Models (QEFVC)(Maaz et al., 2023), we evaluate the models on three areas: Correctness of Information, Detail Orientation, and Contextual Understanding. To do this, we ask an instruction-tuned language model (e.g. GPT-4) about each criterion with respect to the reference summary. To quantify overall performance, we average the three scores, defining it as QEFVC quality score.
While this evaluation metric offers a convenient means for comparison with existing models, they are not without challenges. Past works on language model evaluation have highlighted that solely relying on GPT-4 for model prediction scores is prone to inaccurate evaluation. It is also observed that making the evaluation as fine-grained as possible improves the consistency as well as the precision of the evaluation (Ye et al., 2023). With this in mind, and drawing inspiration from FActScore (Min et al., 2023), we introduce a refined evaluation method, VidFactScore (Video Factual Score) to evaluate the quality of video summaries in a much more fine-grained manner:
For each video and reference summary pair, we break down the reference summary into discrete facts. As an example, “A man and a woman are running.” is segmented into “A man is running.”, and “A woman is running.”. This segmentation is done by an instruction-tuned language model, like GPT-4, with appropriate prompting.
The model-generated summary undergoes a similar division.
An ideal predicted summary should (1) encompass the majority of facts from the reference and (2) minimize the inclusion of facts absent in the reference. Determining the presence or absence of facts is achieved through an instruction-tuned language model with appropriate prompting.
From a quantitative perspective, (1) corresponds to Recall Rate, which calculates the proportion of shared facts between prediction and reference to the total facts in the reference. (2) corresponds to Precision, which calculates the proportion of shared facts to the total facts in the prediction. The harmonic mean of the two numbers, F1, offers a straightforward metric for model comparison.
Results


In a comparison with the current state-of-the-art model (VideoChatGPT), Pegasus-1 exhibits a significant 61% relative improvement on the MSR-VTT dataset and 47% enhancement on the Video Description dataset as measured by the QEFVC Quality Score. Against the ASR+LLM model cohort (including models such as Whisper+ChatGPT and Vendor A), the performance gap widens with Pegasus-1 outperforming by 79% on MSR-VTT and 188% on the Video Description dataset.


Evaluating on our newly proposed VidFactScore-F1 metric, Pegasus-1 shows a 20% absolute increase on the MSR-VTT Dataset and 14% enhancement on the Video Descriptions dataset compared to VideoChatGPT. When benchmarked against the ASR+LLM model cohort, the gains are 25% on the MSR-VTT Dataset and 33% on the Video Descriptions dataset. These findings consistently suggest that VidFactScore aligns well and correlates strongly with evaluations based on the QEFVC framework.
An intriguing observation was made regarding videos predominantly reliant on speech, such as standup routines or lectures. Our model outperforms the ASR+LLM models even in these scenarios. Contrary to initial perceptions that ASR might suffice for such videos, our findings suggest otherwise. We postulate that even minimal visual cues (for instance, "a man performing standup comedy" or “a reaction video”) can enrich speech data, yielding more precise and encompassing summaries. Such outcomes underscore the notion that video comprehension surpasses mere speech understanding. It is clear that incorporating both visual and speech modalities is necessary to achieve a comprehensive understanding. See the “Reaction Video” in the “In-the-wild Examples” section below.
In-the-wild examples
These are sample examples that are randomly selected from diverse domains to illustrate the capabilities of Pegasus-1 compared to the existing approaches.
Note that the generated output may contain
Hallucinations (creating a coherent story even though it is not factually depicted in the video)
Irrelevant answers due to not being able to comprehend the prompt or question
Biases
We welcome any feedback and will do our best to address them in the near future.
Related articles
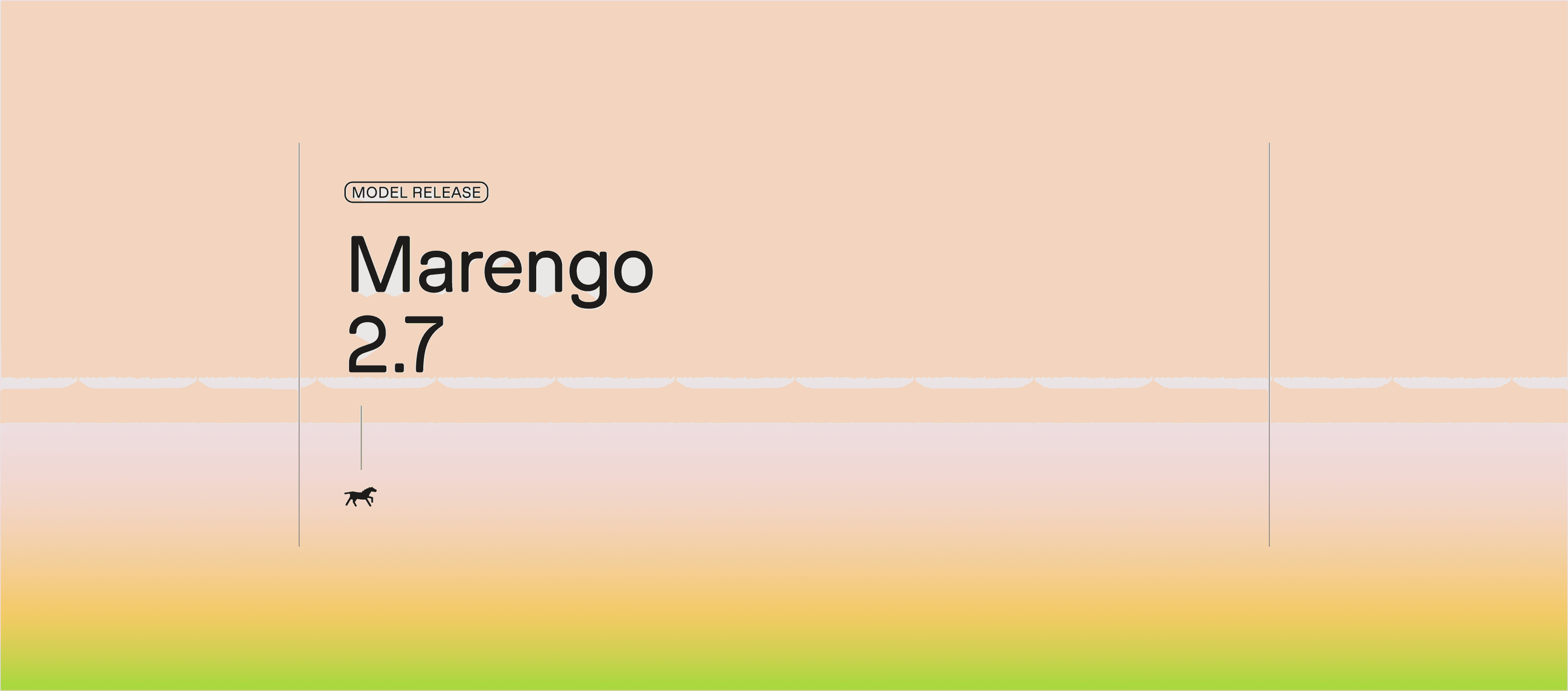
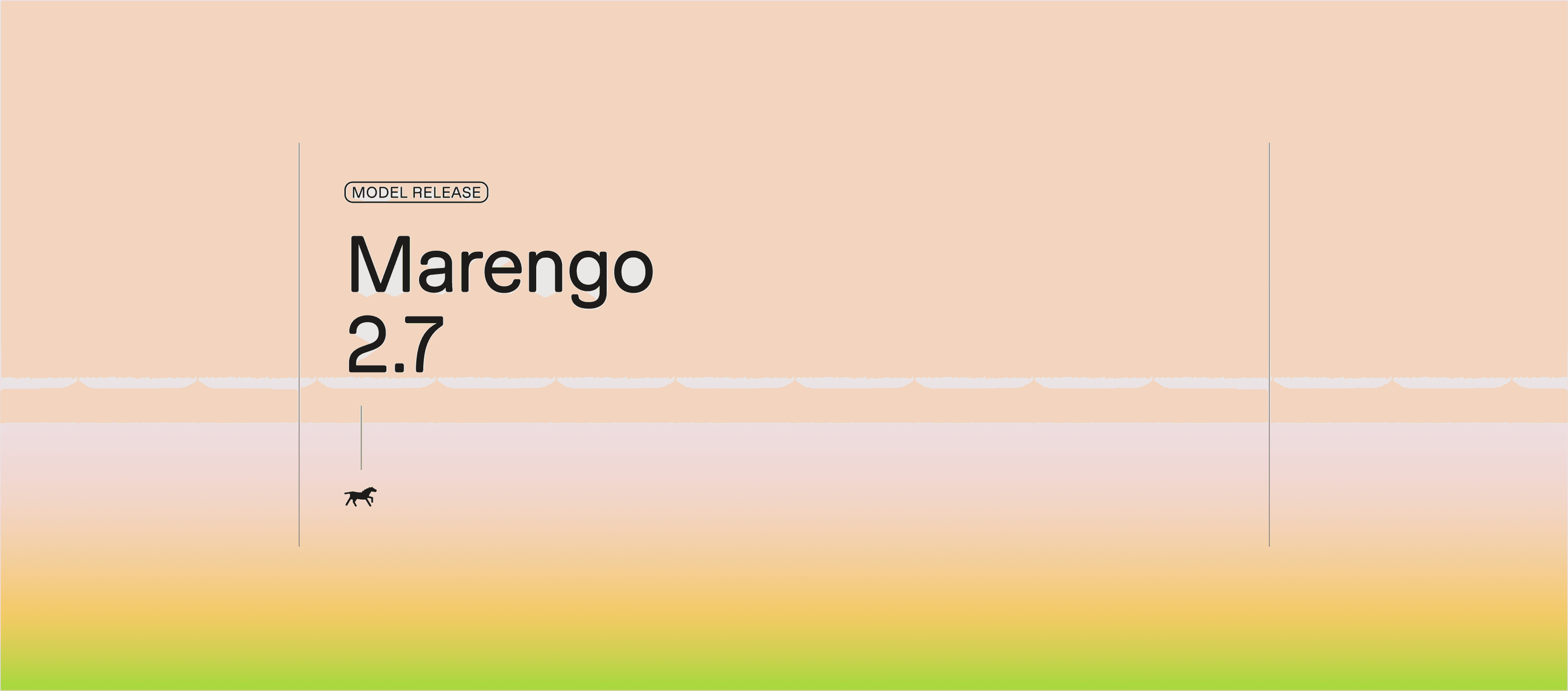
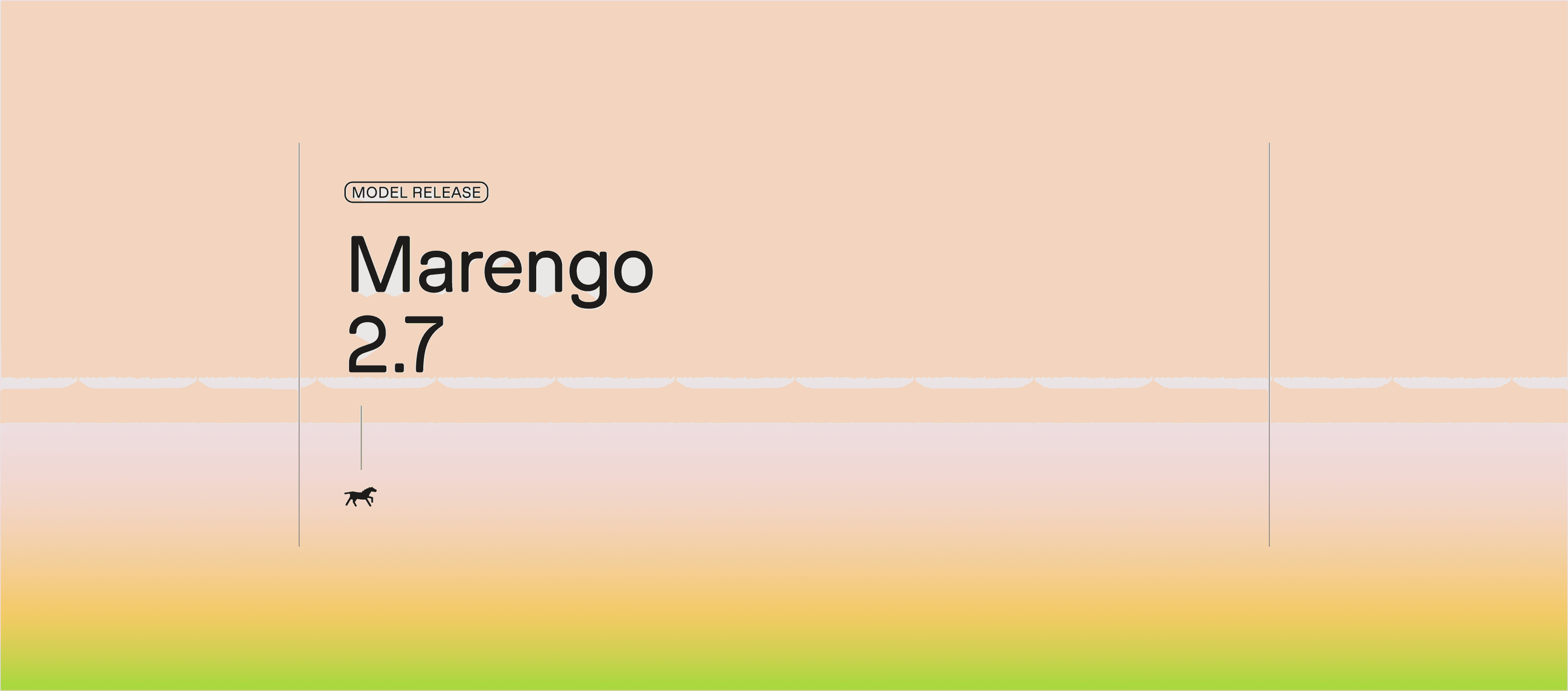
Introducing Marengo 2.7: Pioneering Multi-Vector Embeddings for Advanced Video Understanding
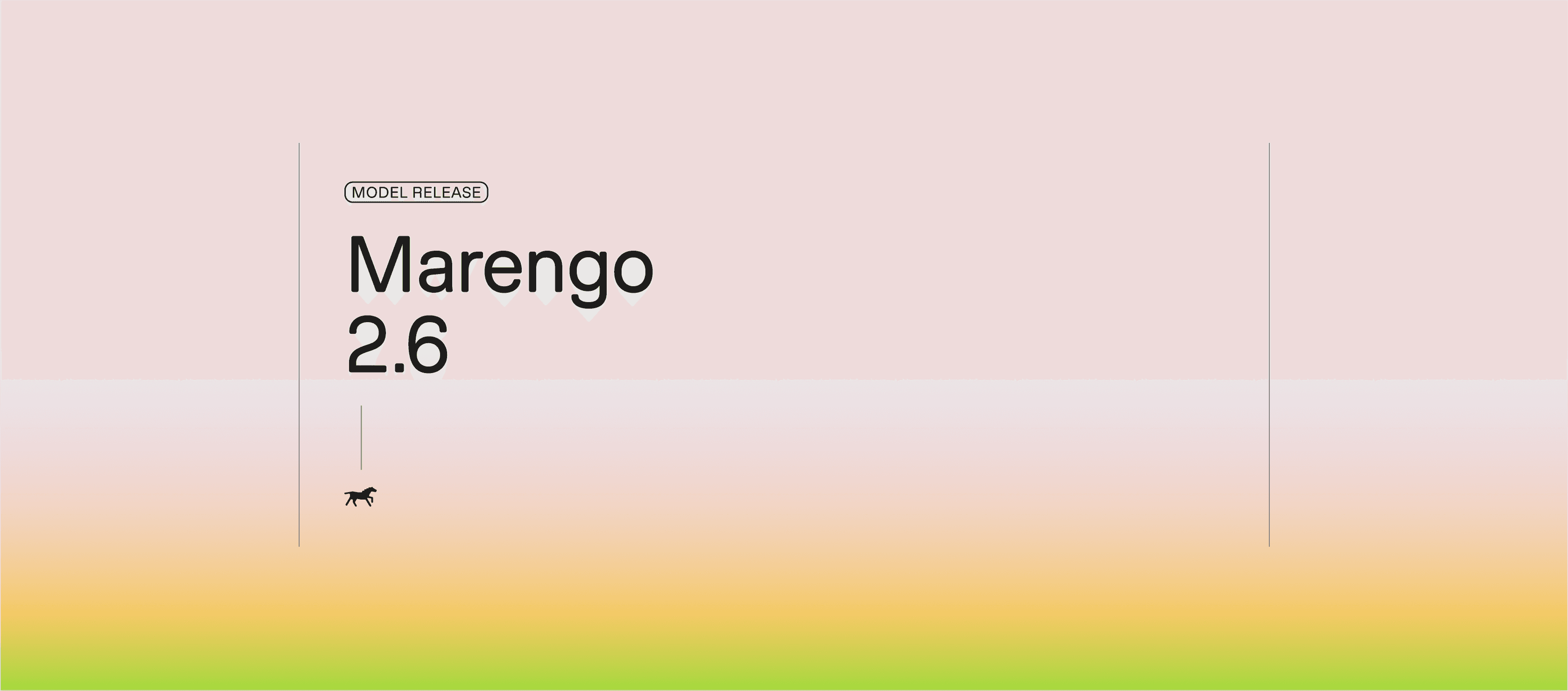
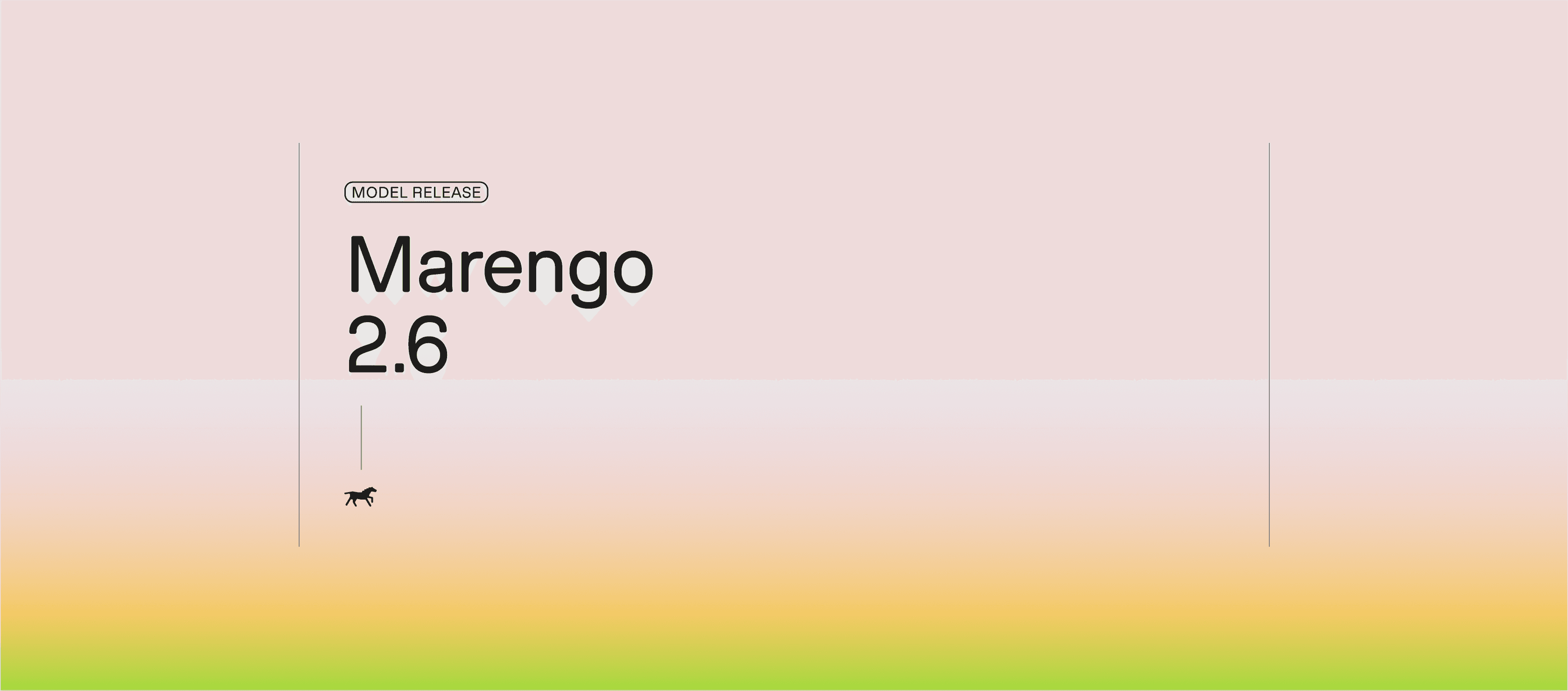
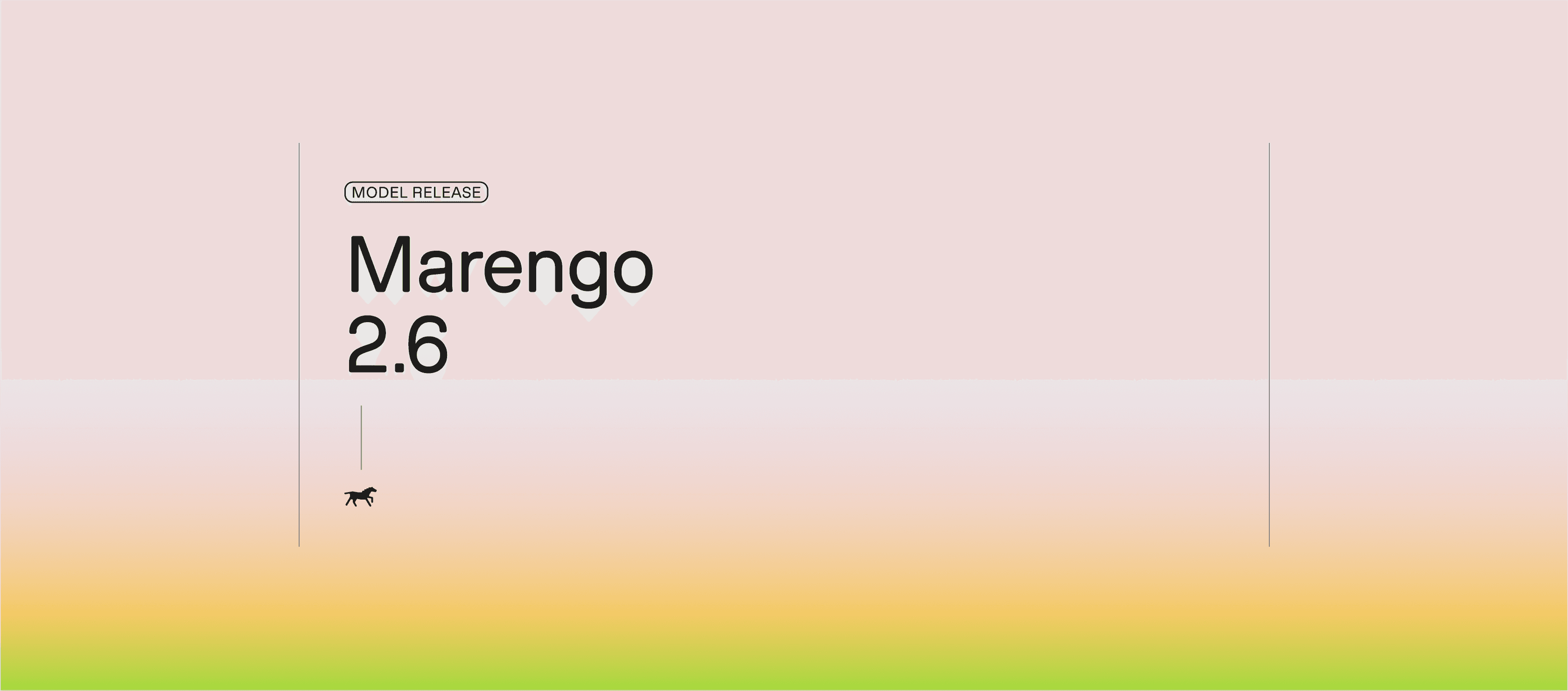
Introducing Marengo-2.6: A New State-of-the-Art Video Foundation Model for Any-to-Any Search
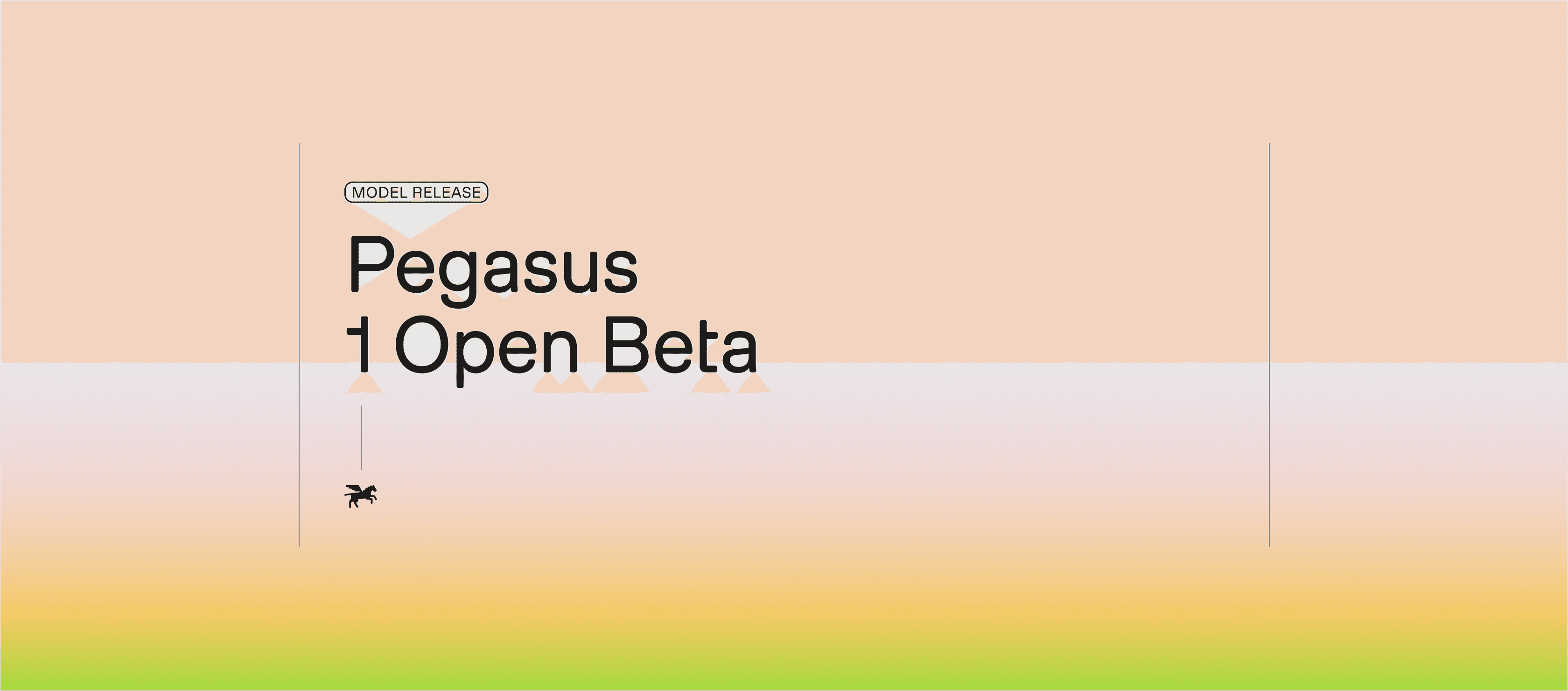
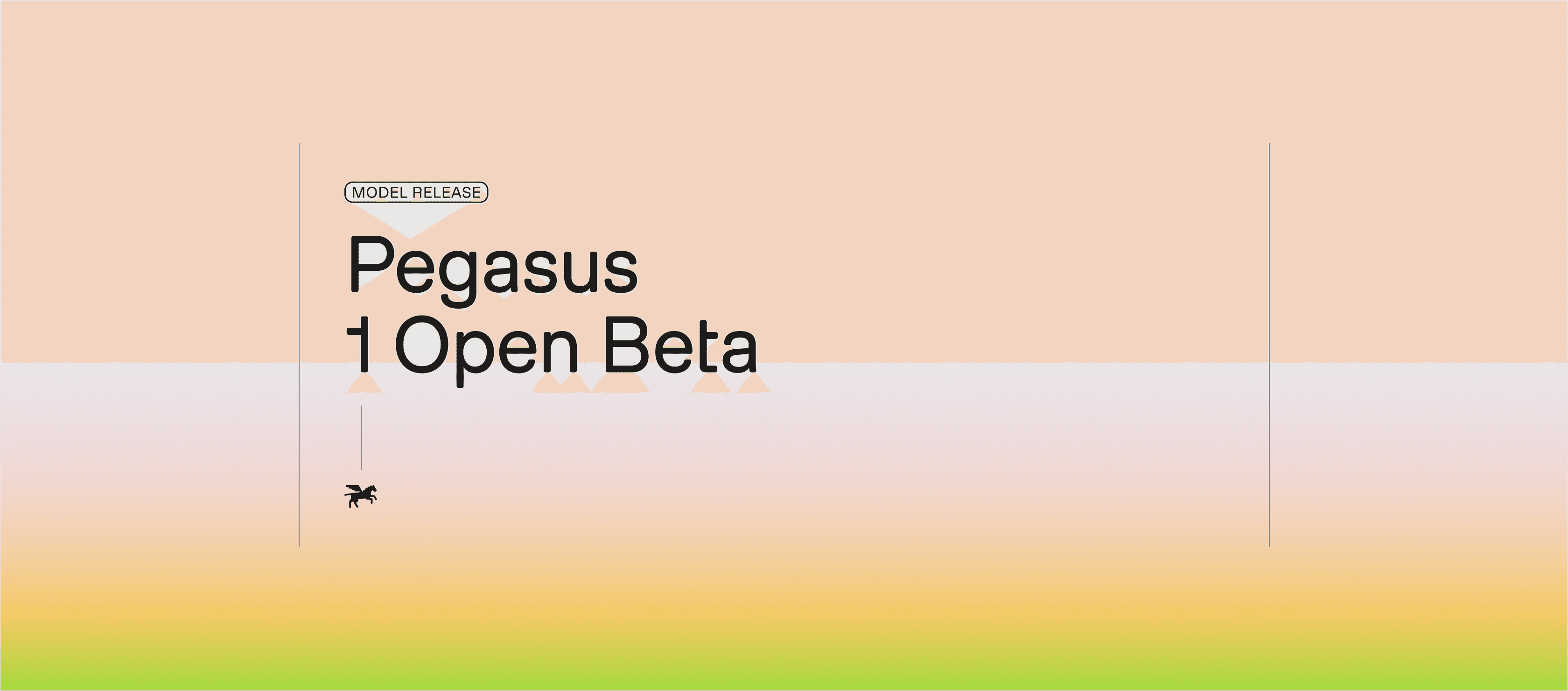
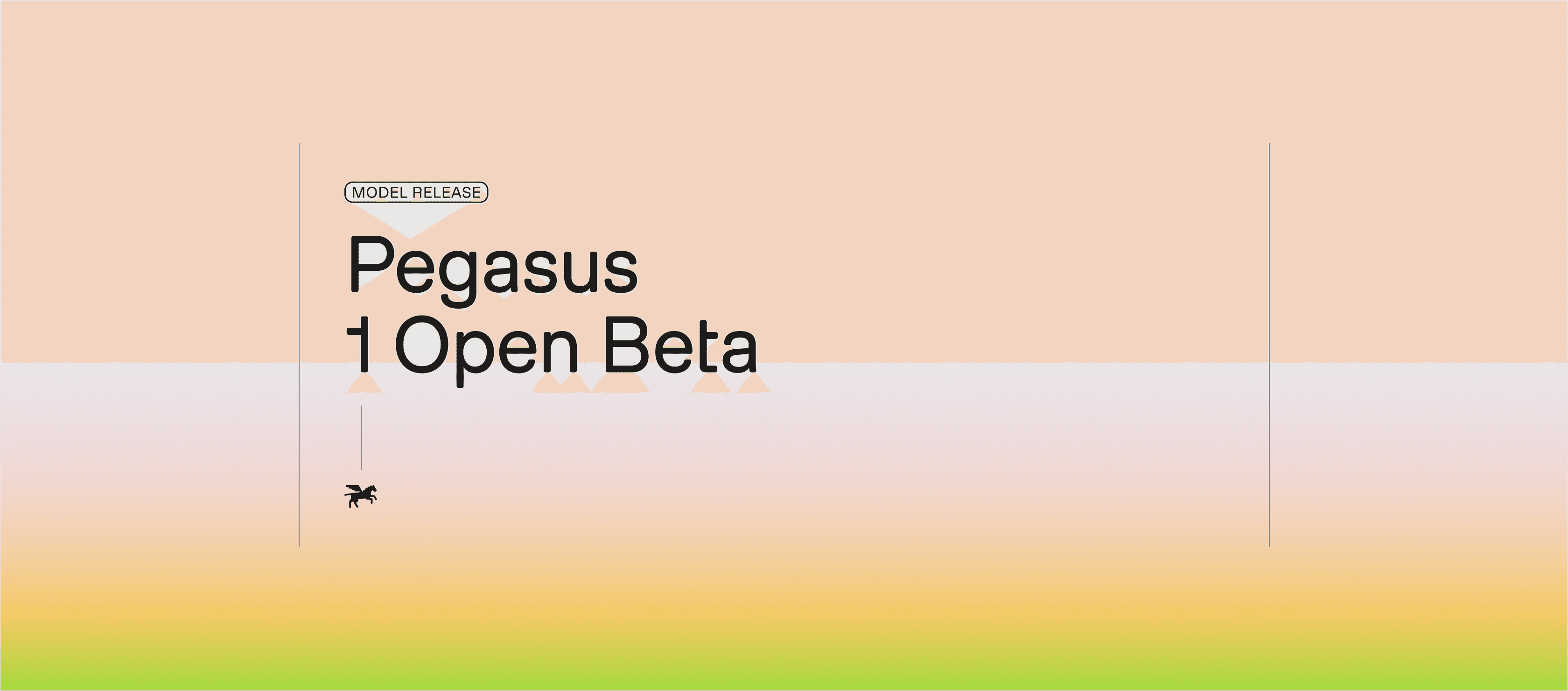
Pegasus-1 Open Beta: Setting New Standards in Video-Language Modeling
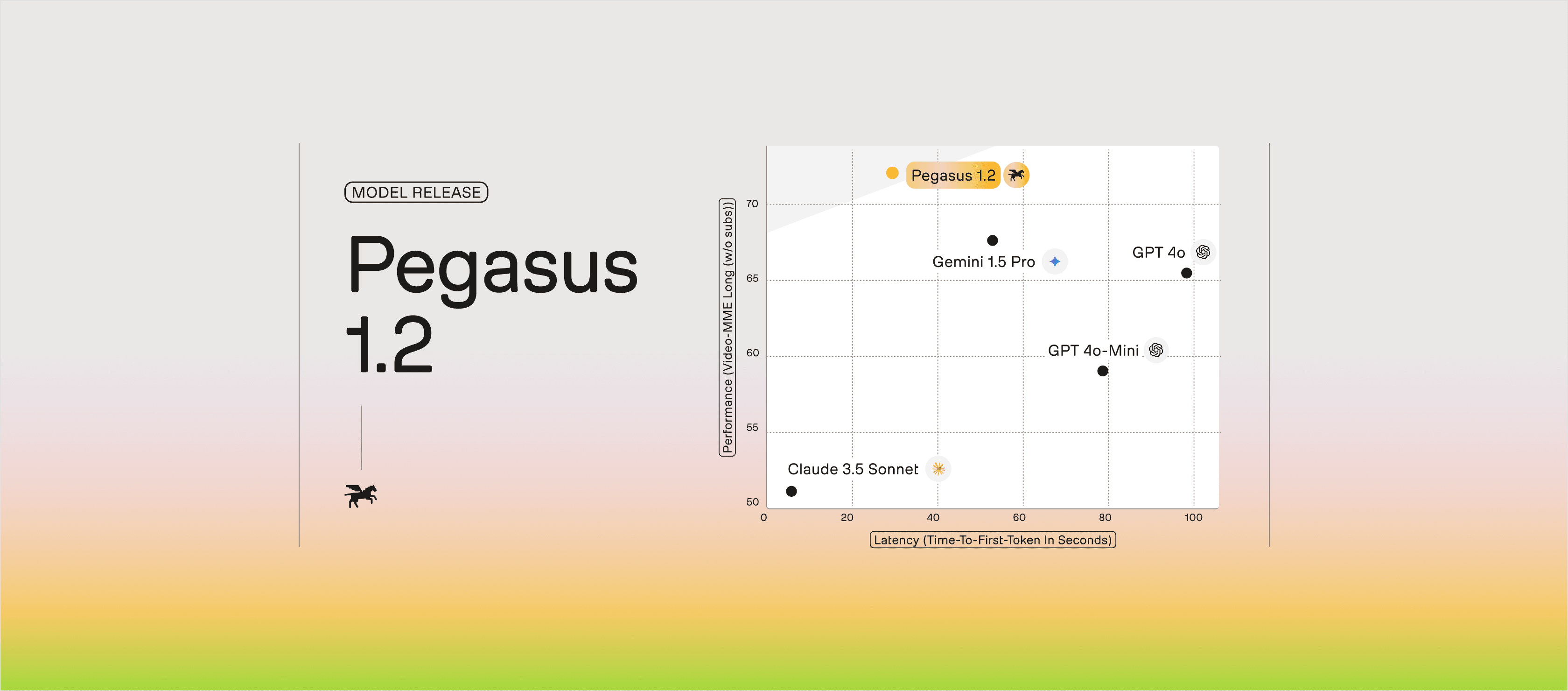
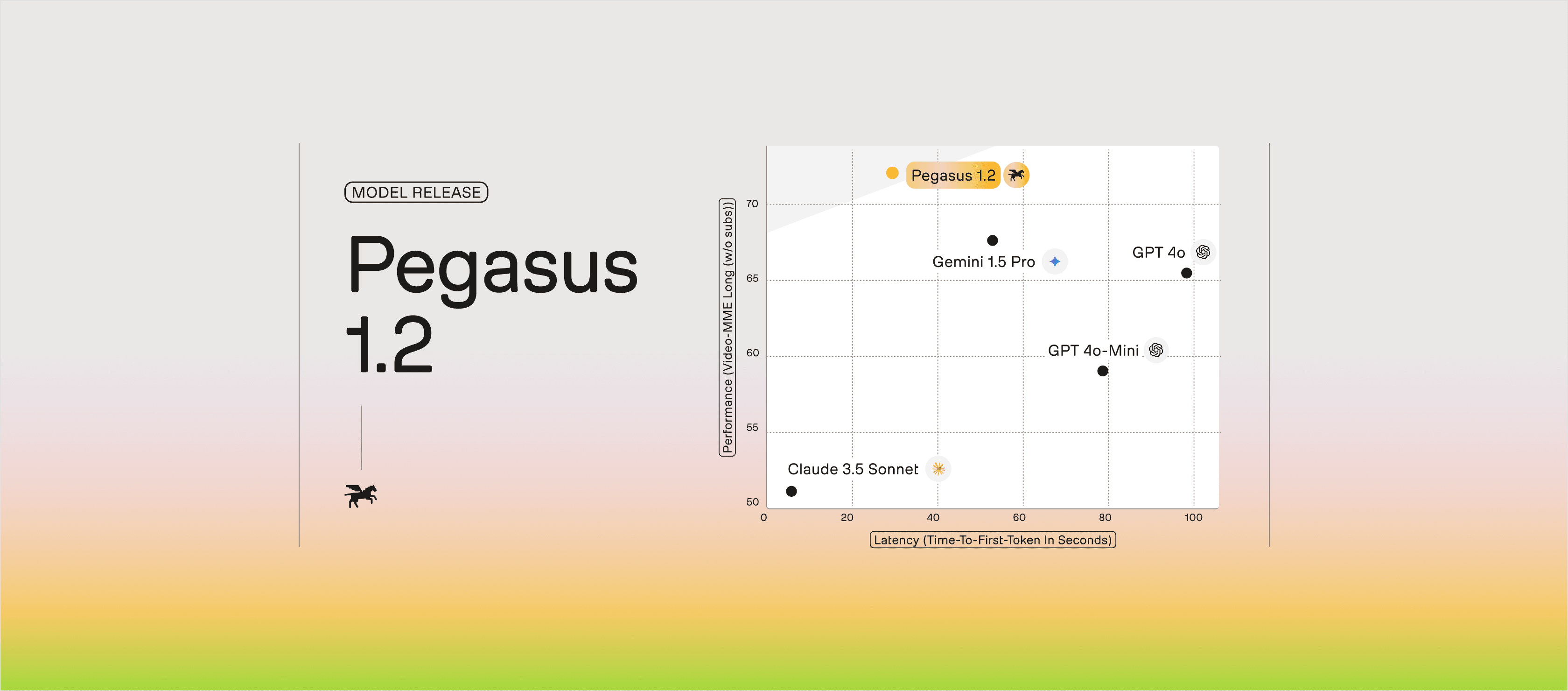
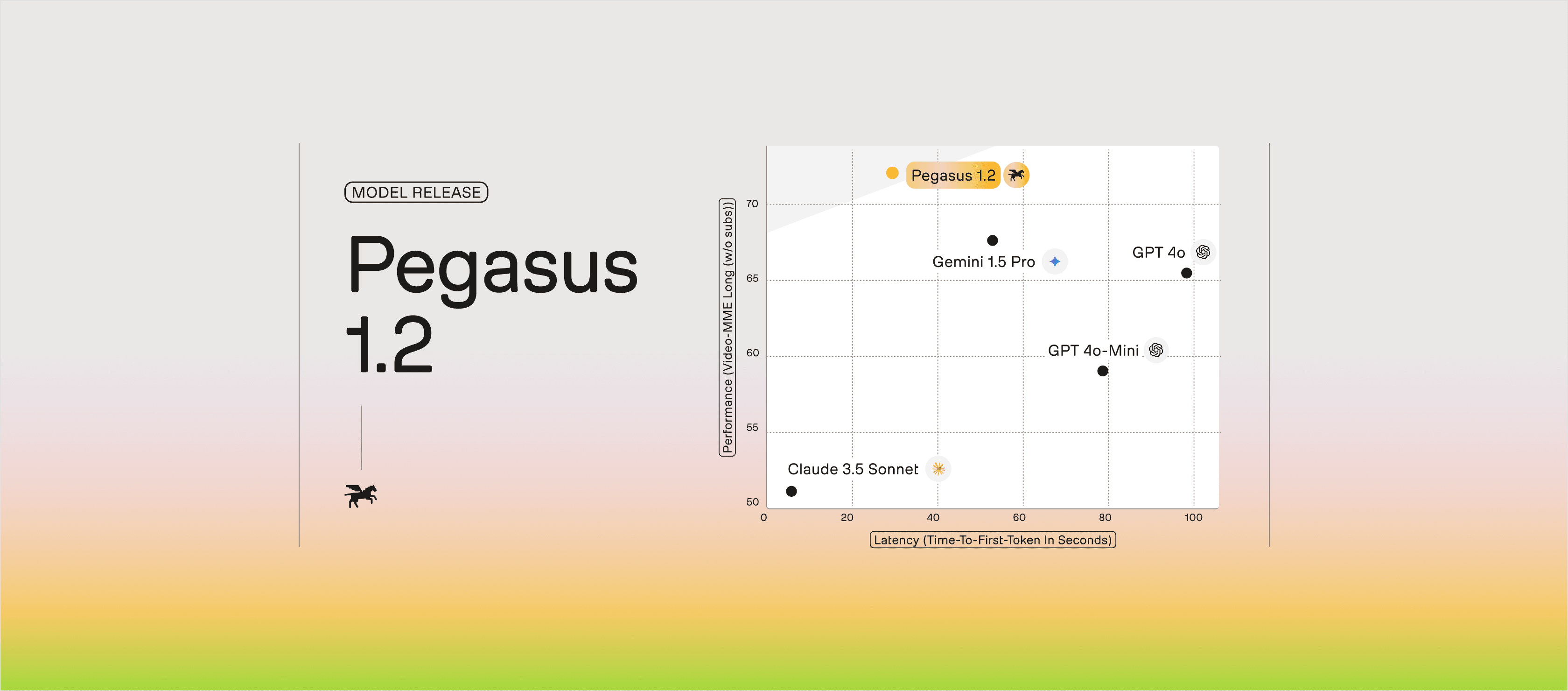
Introducing Pegasus 1.2: An Industry-Grade Video Language Model for Scalable Applications