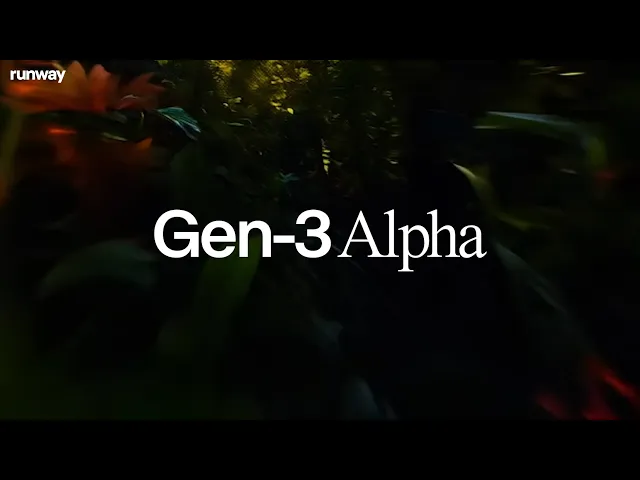
AI/ML
AI/ML
AI/ML
The State of Video-Language Models: Research Insights from the Inaugural NeurIPS Workshop


James Le
James Le
James Le
We hosted the inaugural workshop on video-language models at NeurIPS 2024 in Vancouver on December 14. Let's recap insights from the workshop!
We hosted the inaugural workshop on video-language models at NeurIPS 2024 in Vancouver on December 14. Let's recap insights from the workshop!


Join our newsletter
Receive the latest advancements, tutorials, and industry insights in video understanding
Search, analyze, and explore your videos with AI.
Dec 31, 2024
Dec 31, 2024
Dec 31, 2024
24 Min
24 Min
24 Min
Copy link to article
Copy link to article
Copy link to article
The inaugural NeurIPS 2024 Video-Language Model Workshop marked a pivotal moment in the rapidly evolving field of video understanding and generation. In his opening remarks, our CTO Aiden Lee highlighted the growing interest in video-language models across two key areas: video understanding (video-to-text) and video generation (text-to-video).
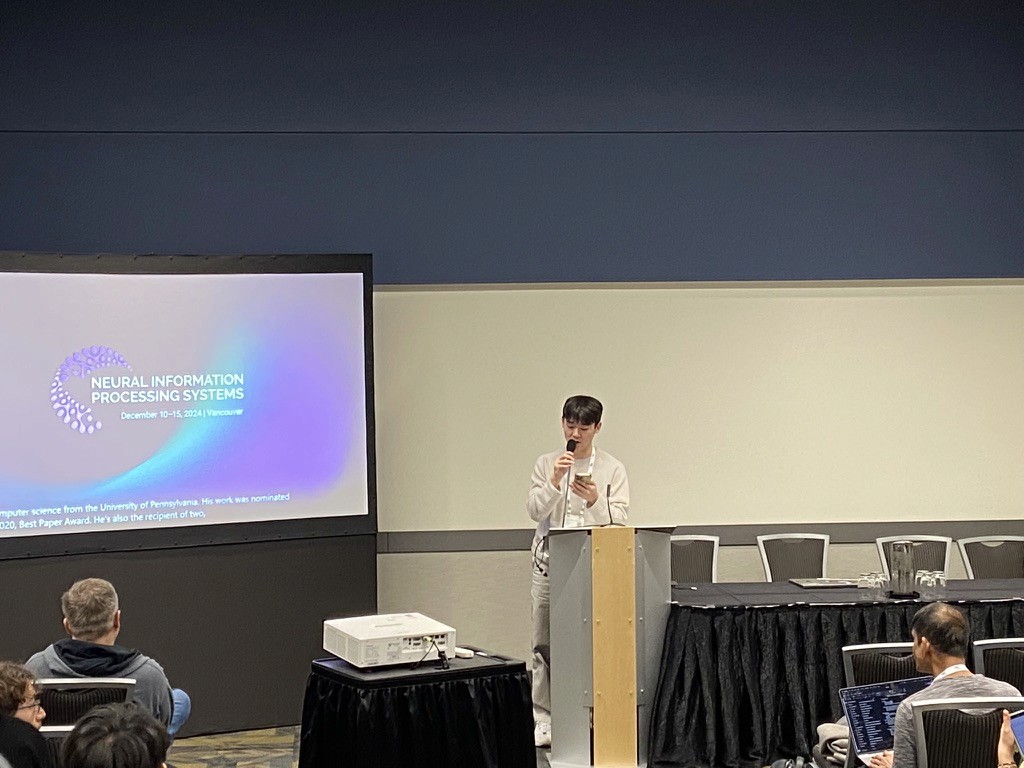
The applications of these technologies span diverse sectors, including content creation, advertising, healthcare, and robotics. Despite the exponential growth in vision-language model research, the field faces several critical challenges:
Data scarcity, particularly in high-quality video and text pairs
Scale and complexity issues in video storage and processing
Challenges in multimodal integration across visual, audio, and text components
Difficulties in benchmarking and evaluating video-language alignment
The workshop aimed to address these challenges by bringing together researchers to discuss current obstacles, foster open collaboration, and accelerate the development of video foundation models for real-world applications.
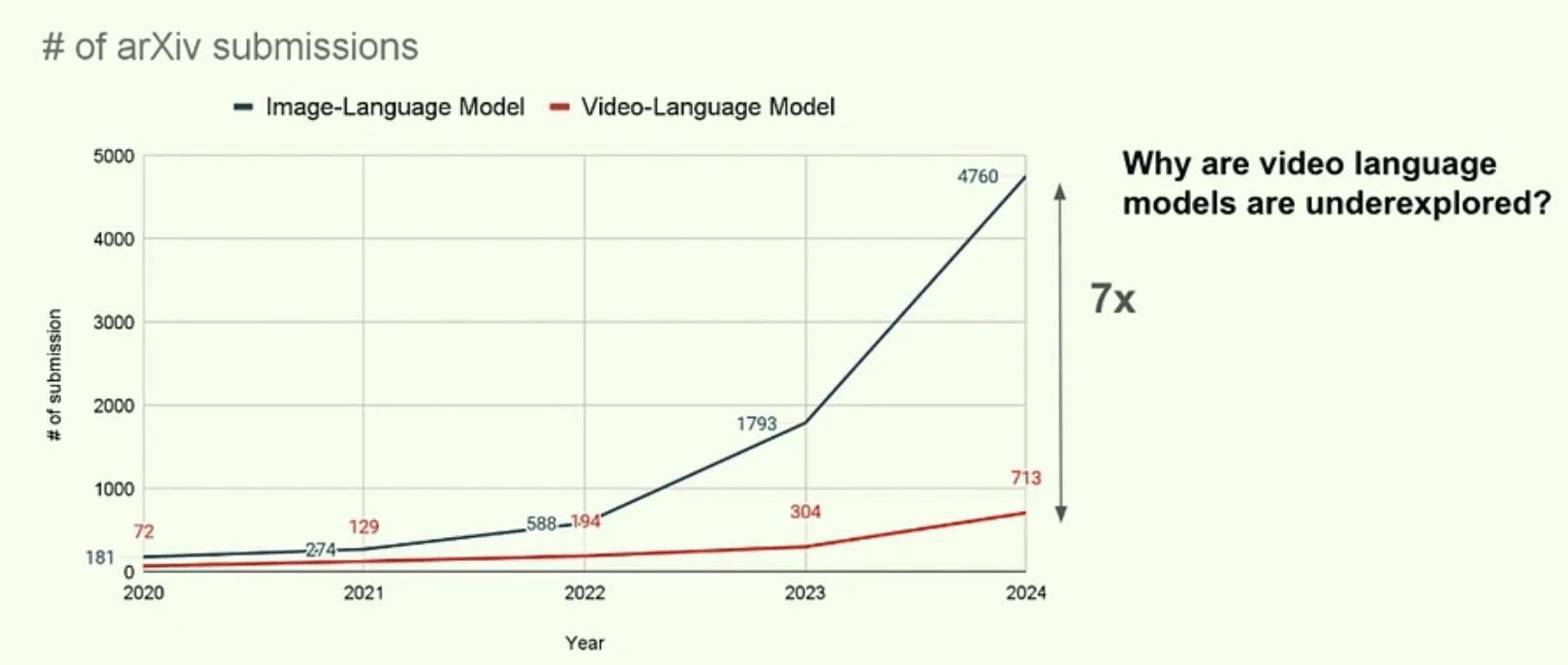
1 - Egocentric Video and Language Understanding - Dima Damen
Professor Dima Damen's comprehensive talk explored four cutting-edge aspects of egocentric video and language understanding. Her research draws from the EPIC-KITCHENS dataset and introduces several groundbreaking approaches.
Unique Captioning for Similar Actions
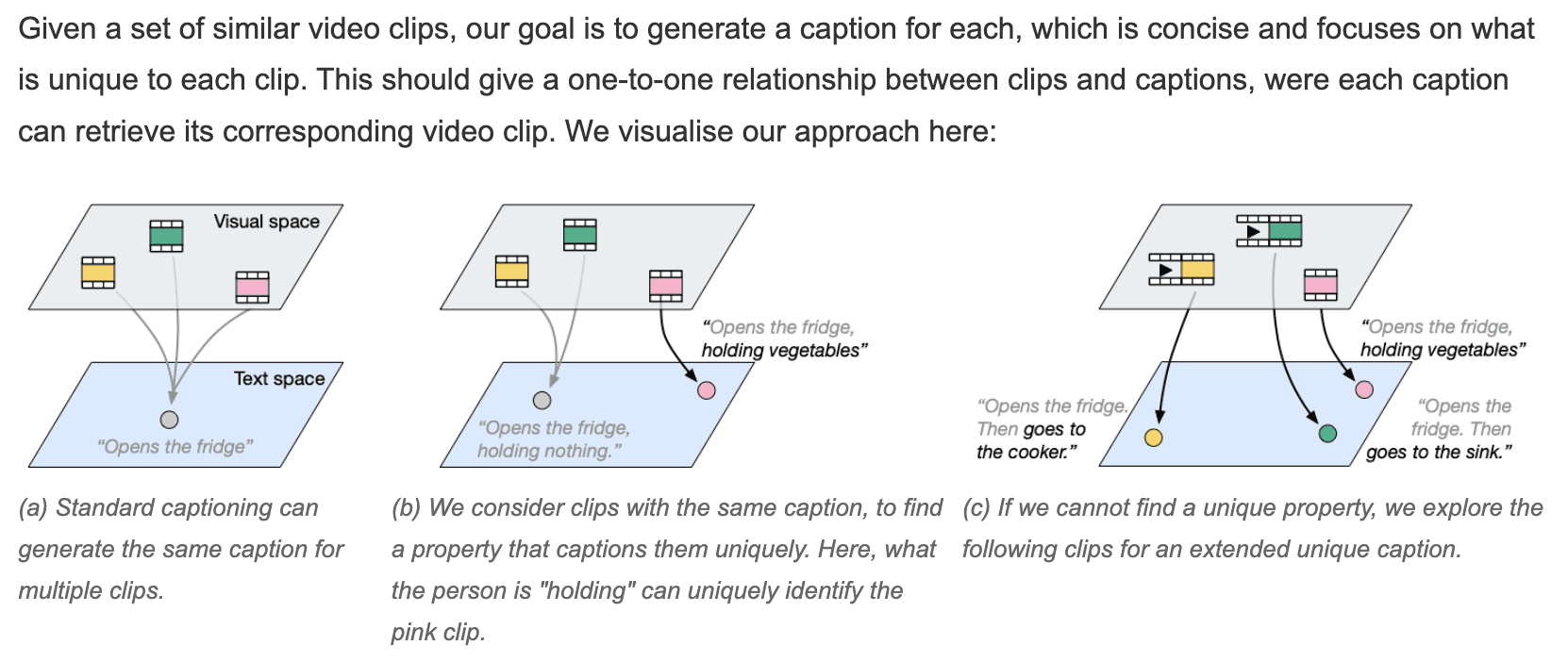
The paper "It's Just Another Day," which won the Best Paper Award at ACCV 2024, addresses a fundamental challenge in video captioning: the tendency of current methods to generate identical captions for similar clips when processing them independently.
The key insight behind this work is that while life is repetitive, we need distinct descriptions for similar actions. The researchers propose a novel approach called Captioning by Discriminative Prompting (CDP) that:
Considers clips jointly rather than in isolation
Utilizes a bank of discriminative prompts to generate unique captions
Evaluates through both video-to-text and text-to-video retrieval metrics
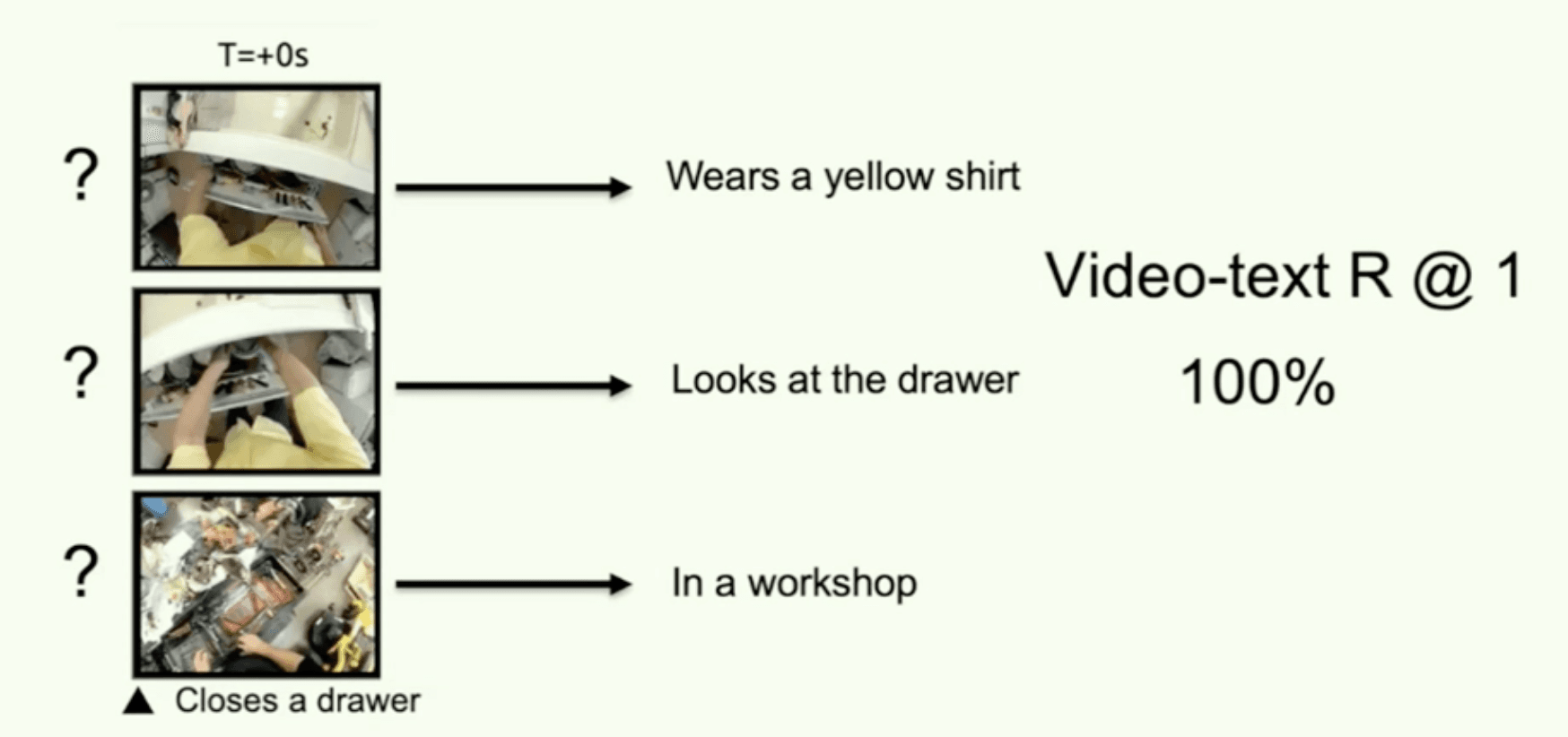
The method was evaluated on two domains: egocentric videos from Ego4D and timeloop movies. When applied to the LaViLa VCLM captioner, CDP showed significant improvements in caption uniqueness while maintaining semantic accuracy.
Domain Generalization Using Language
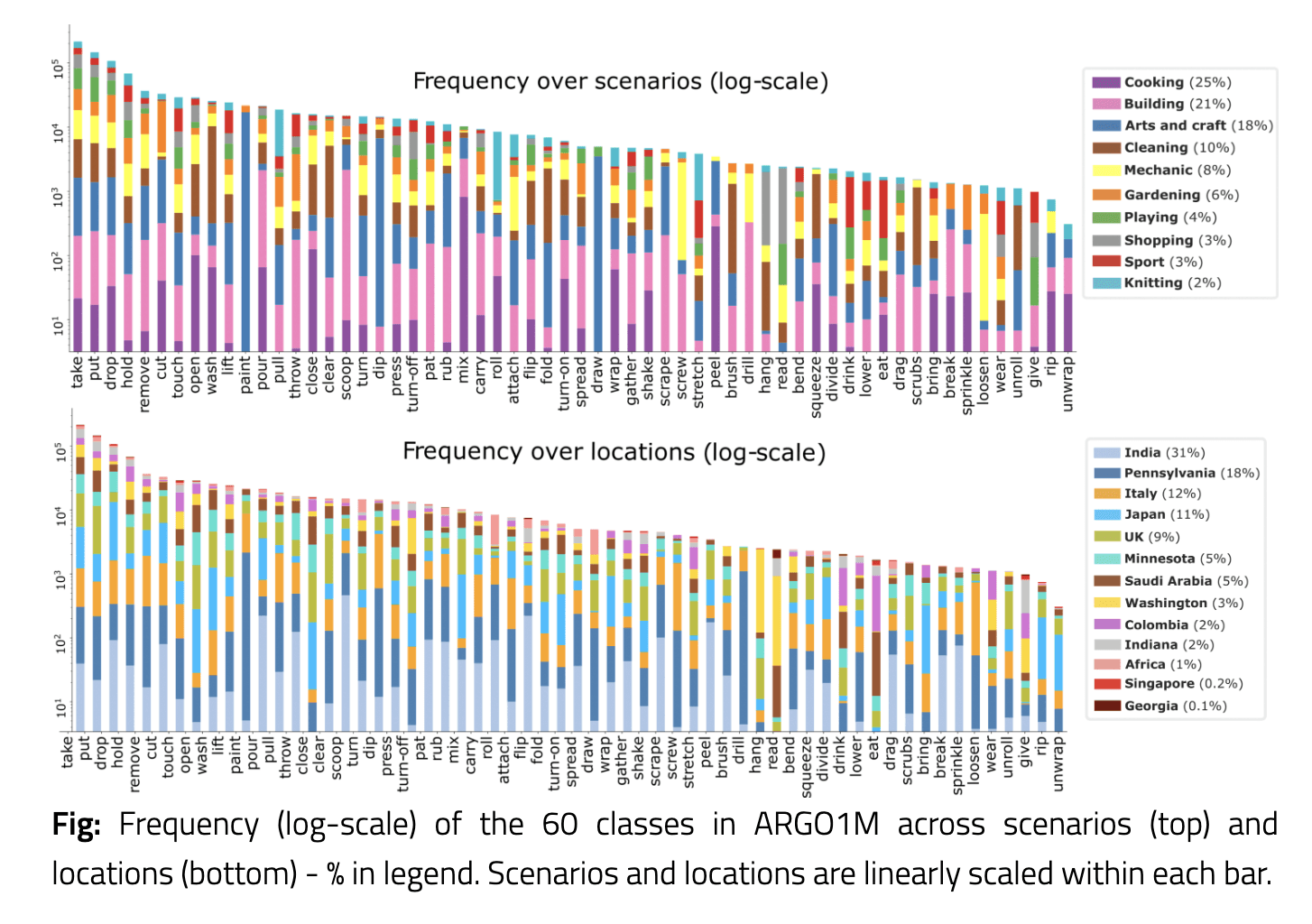
A major contribution is the ARGO1M dataset, the first of its kind designed specifically for Action Recognition Generalization across scenarios and locations. The dataset features:
1.05M action clips spanning 60 action classes
Recordings from 13 different locations
10-second clip duration standard
The accompanying paper "What can a cook in Italy teach a mechanic in India?" introduces Cross-Instance Reconstruction (CIR), which employs two key reconstructions:
Video-text association reconstruction using text narrations
Classification reconstruction trained for action class recognition
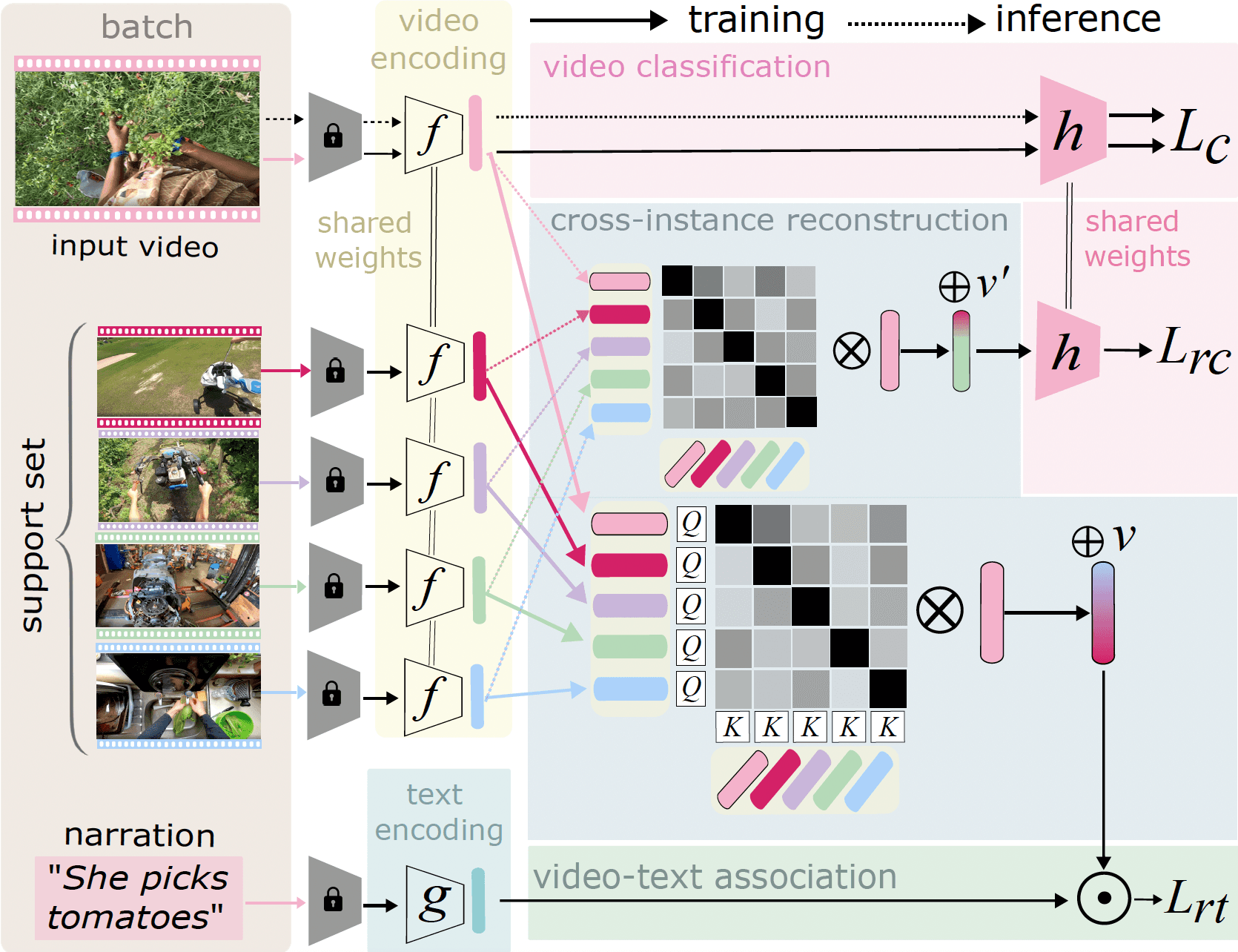
CIR represents actions as weighted combinations of actions from different scenarios and locations, enabling robust cross-domain generalization.
Hand-Object Interaction Referral
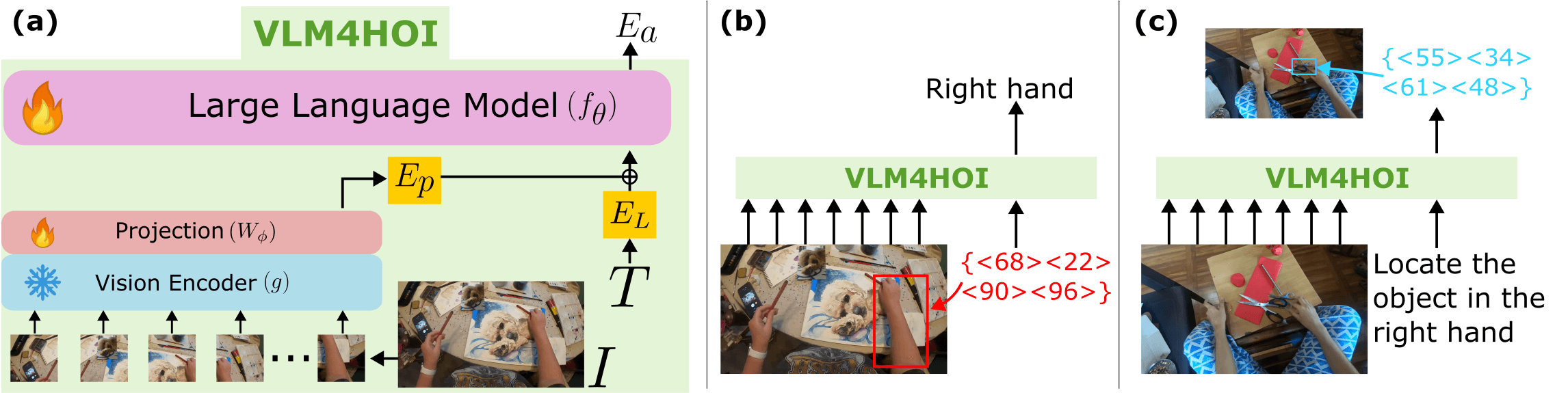
The HOI-Ref project introduces VLM4HOI, a sophisticated model for hand-object interaction referral in egocentric images. The model architecture includes:
A vision encoder (g) and projection layer (Wφ) for image processing
Language model embedding space integration
Capability to identify both hands and interacted objects
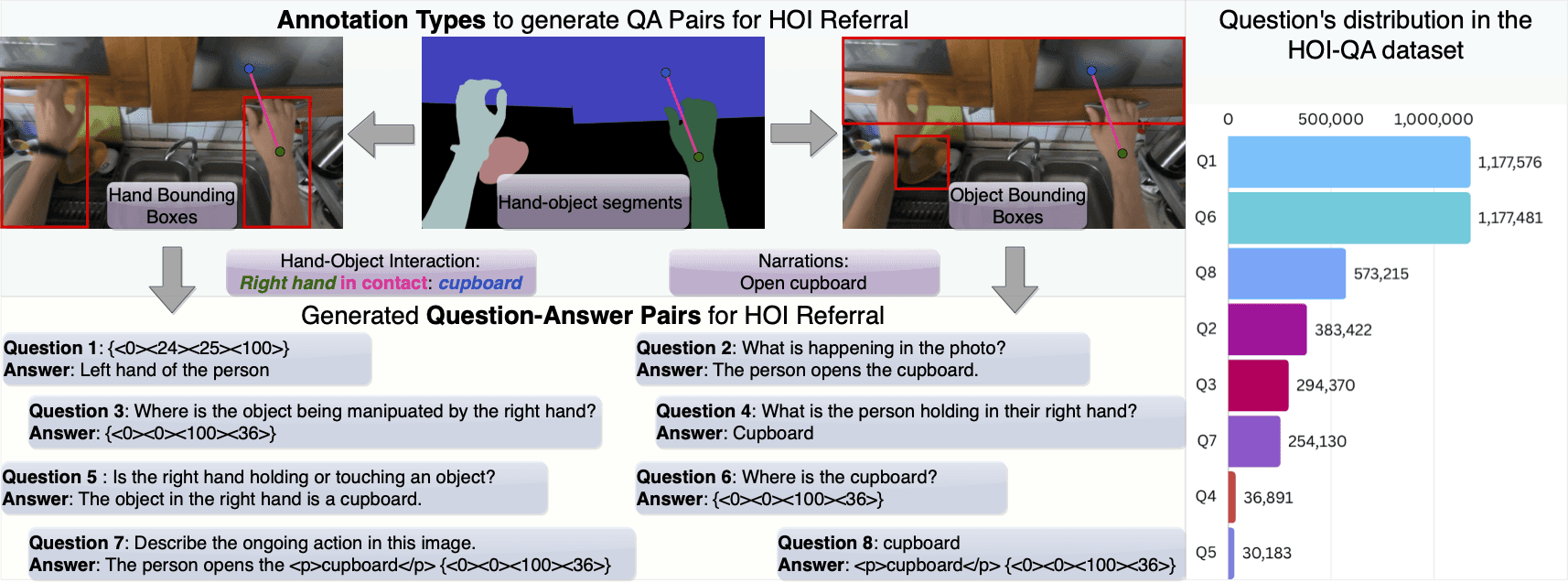
The researchers developed HOI-QA, a comprehensive dataset containing 3.9M question-answer pairs, focusing on:
Spatial referral and recognition of hands and objects
Understanding of hand-object interactions
Direct referral versus interaction referral capabilities
Audio-Visual Semantic Integration
This section presented two significant contributions to the field:
EPIC-SOUNDS Dataset
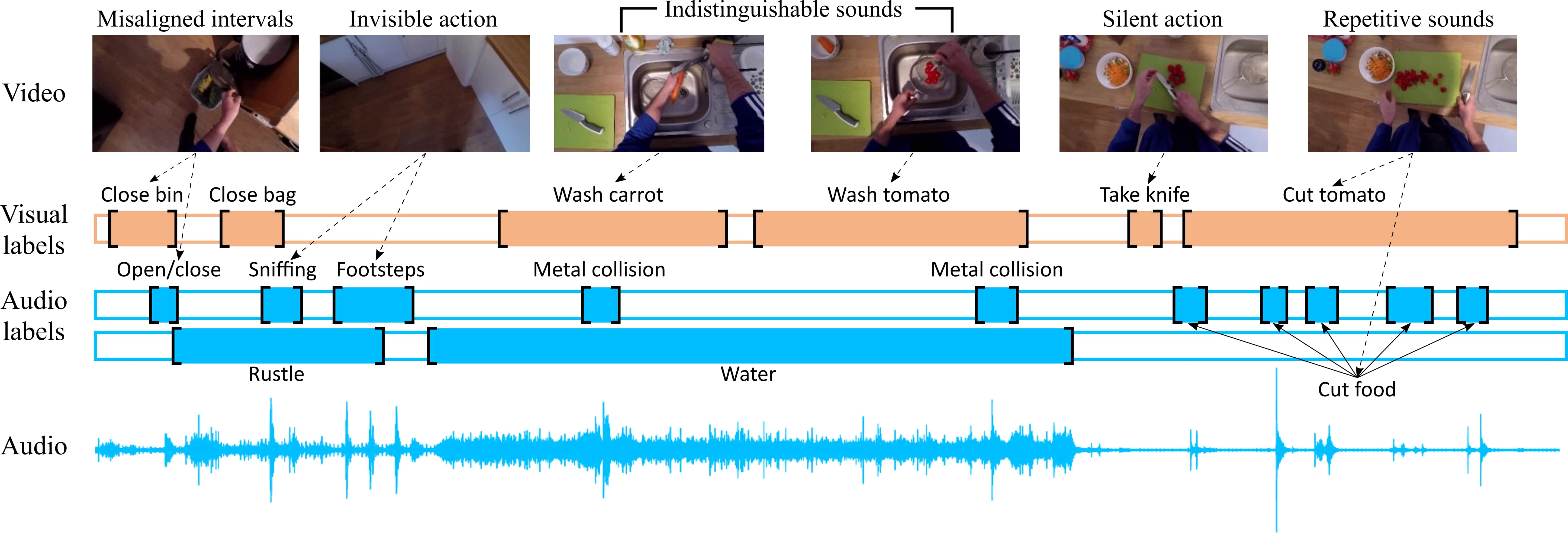
This large-scale dataset captures audio annotations within egocentric videos from EPIC-KITCHENS-100, featuring:
75.9k segments of audible events and actions
44 distinct action classes
Detailed material annotations for object collision sounds
Temporal labeling of distinguishable audio segments
Time Interval Machine (TIM)
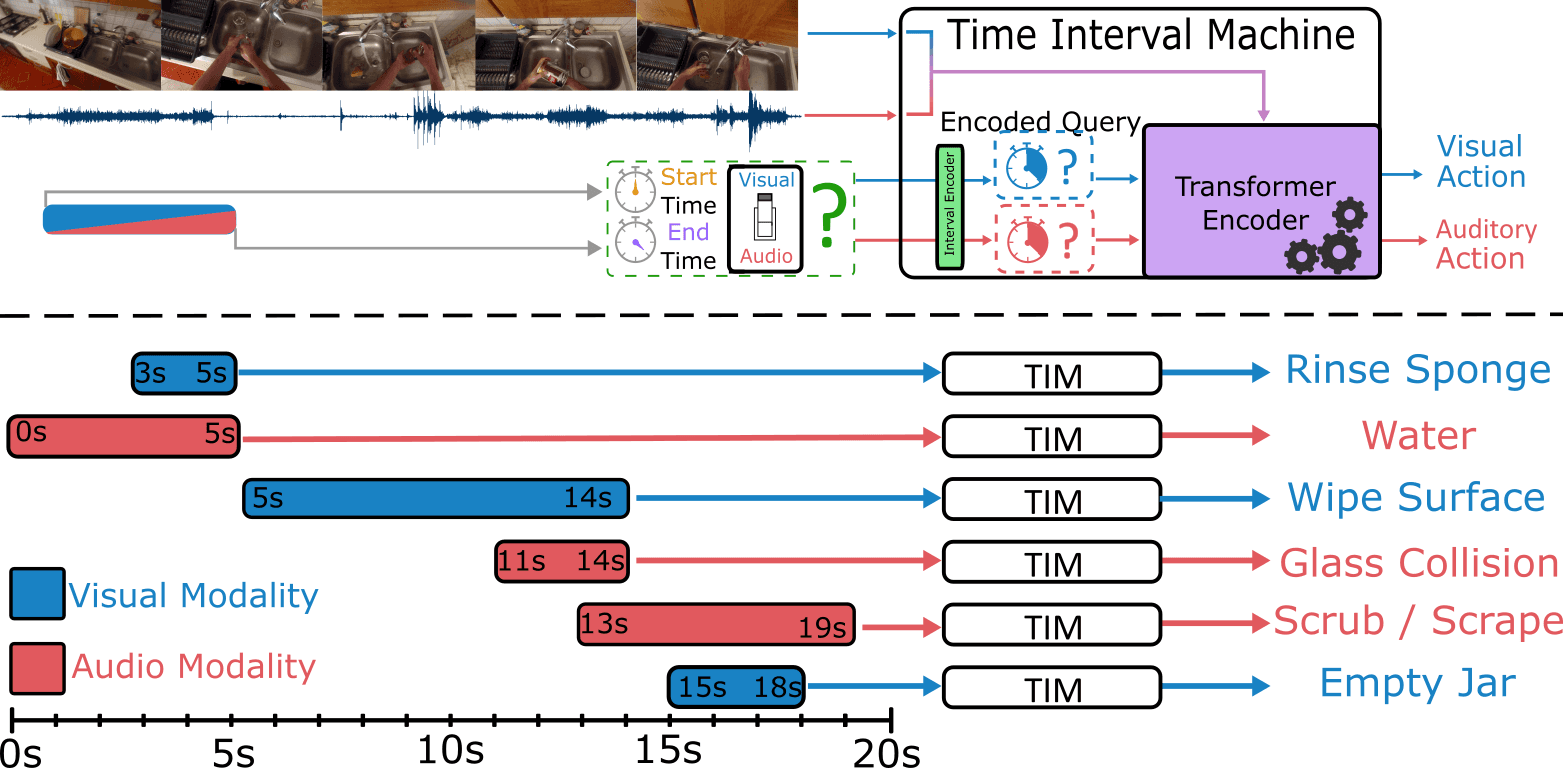
TIM represents a breakthrough in handling the different temporal extents of audio and visual events in long videos. Key features include:
A transformer encoder that processes modality-specific time intervals
Attention mechanisms for both interval-specific and surrounding context
State-of-the-art performance on multiple benchmarks
The model achieved remarkable results:
2.9% improvement in top-1 action recognition accuracy on EPIC-KITCHENS
Superior performance on Perception Test and AVE datasets
Successful adaptation for action detection using dense multi-scale interval queries
These advances collectively demonstrate significant progress in understanding and processing egocentric video content, particularly in handling the complex interplay between visual, textual, and audio modalities. The research from Dima’s group presents a comprehensive framework for addressing the challenges of multimodal integration in egocentric video understanding.
2 - Complex Video Understanding using Language and Video - Gedas Bertasius
Professor Gedas Bertasius's talk highlighted both the remarkable progress and current limitations of video-language models (VLMs) in complex video understanding tasks. While acknowledging the significant advances in video understanding due to LLMs and VLMs, he emphasized that modern VLMs still face fundamental challenges with complex video understanding tasks.
Addressing Long-Range Video Understanding
A key technical challenge identified is the struggle of existing models to localize relevant content in long video sequences. Bertasius introduced Structured State-Space Models (S4) as an efficient approach to model long-range dependencies in video (as seen in this work and this work). However, he noted that S4 models struggle with tasks requiring selective attention to specific parts of the input sequence, as their parameters remain constant for every input token.
BIMBA: Selective-Scan Compression
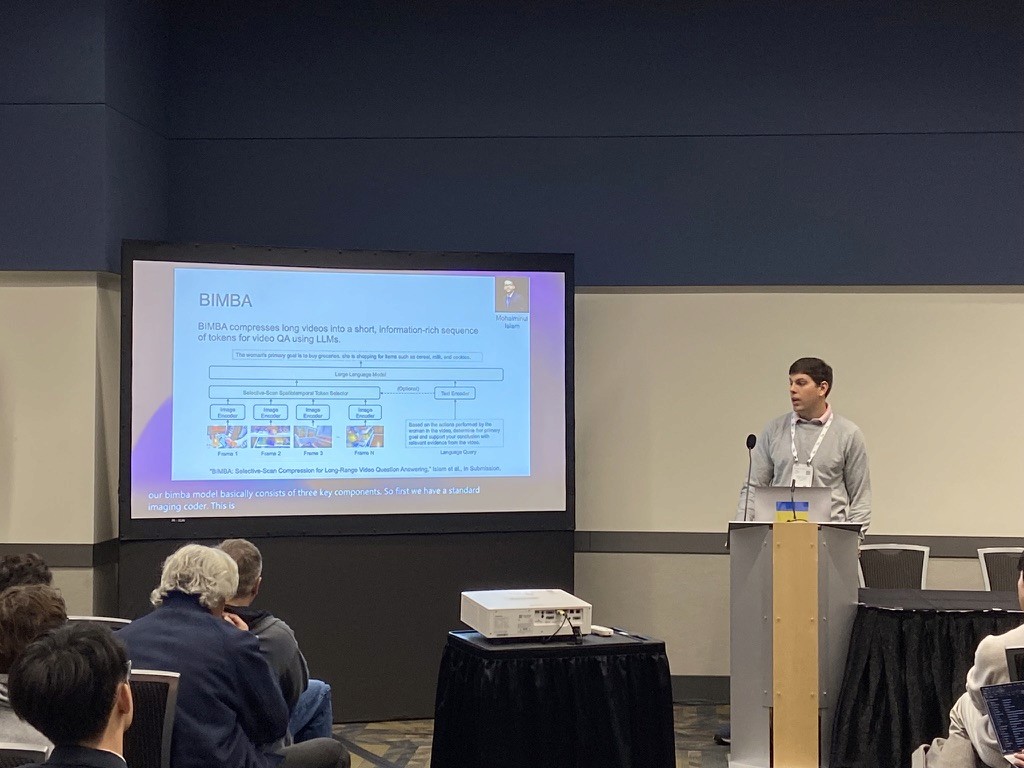
BIMBA addresses these limitations by introducing the selective scan algorithm (also known as Mamba) to choose which parts of the input should be stored in the hidden state in a data-dependent manner. The model features:
A spatiotemporal token selector using bidirectional selective-scan mechanism
Interleaved visual queries for precise content selection
Input-dependent parameters for adaptive processing
Efficient compression of long videos into information-rich token sequences
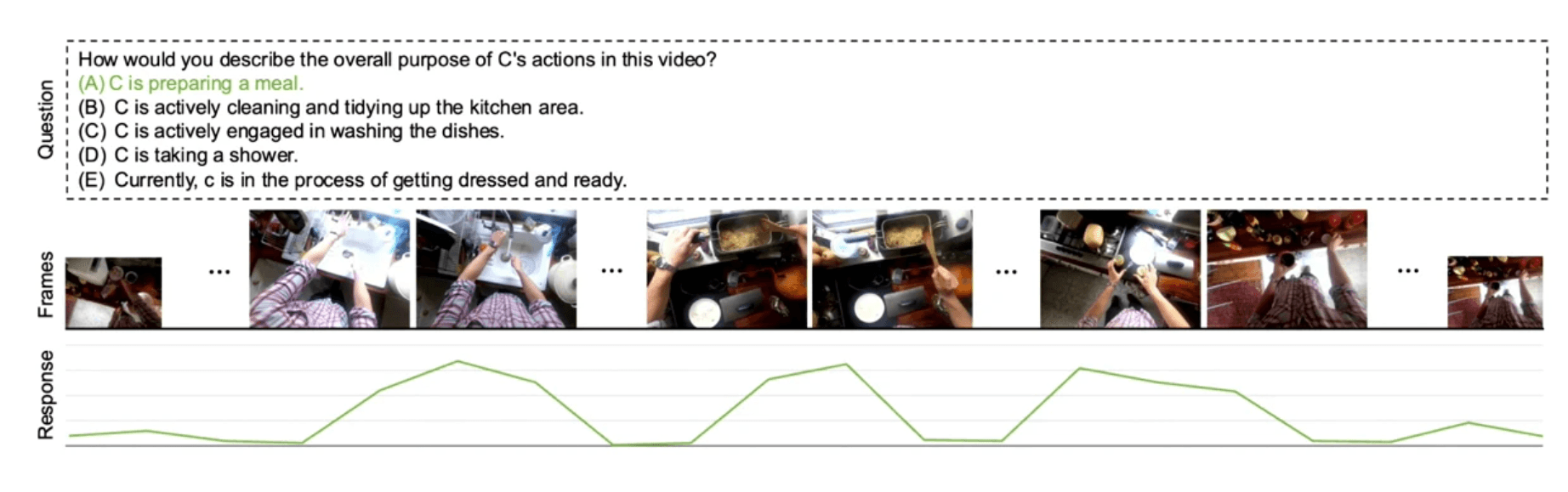
In evaluation on the EgoSchema benchmark, BIMBA demonstrated superior performance in identifying the most relevant parts of videos for question answering tasks, with qualitative results showing precise selection of video segments relevant to given queries.
LLoVi: LLM Framework for Long VideoQA
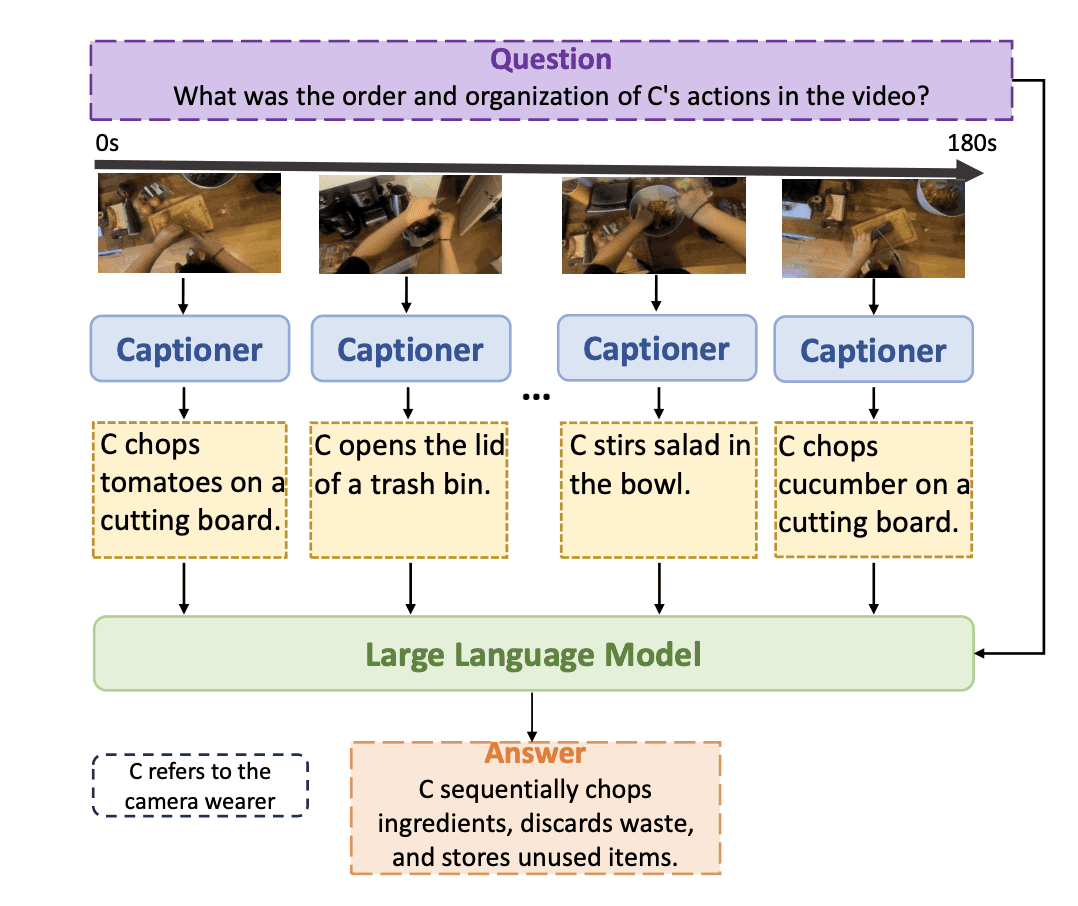
LLoVi introduces a more straightforward but highly effective approach to long-range video question answering through a two-stage process:
Stage 1: Video Processing
Samples short clips from long videos
Extracts captions for each clip
Maintains temporal ordering of information
Stage 2: Question Answering
Feeds temporally ordered captions into an LLM
Employs a novel two-round summarization prompt
Enhances complex question-answering capabilities
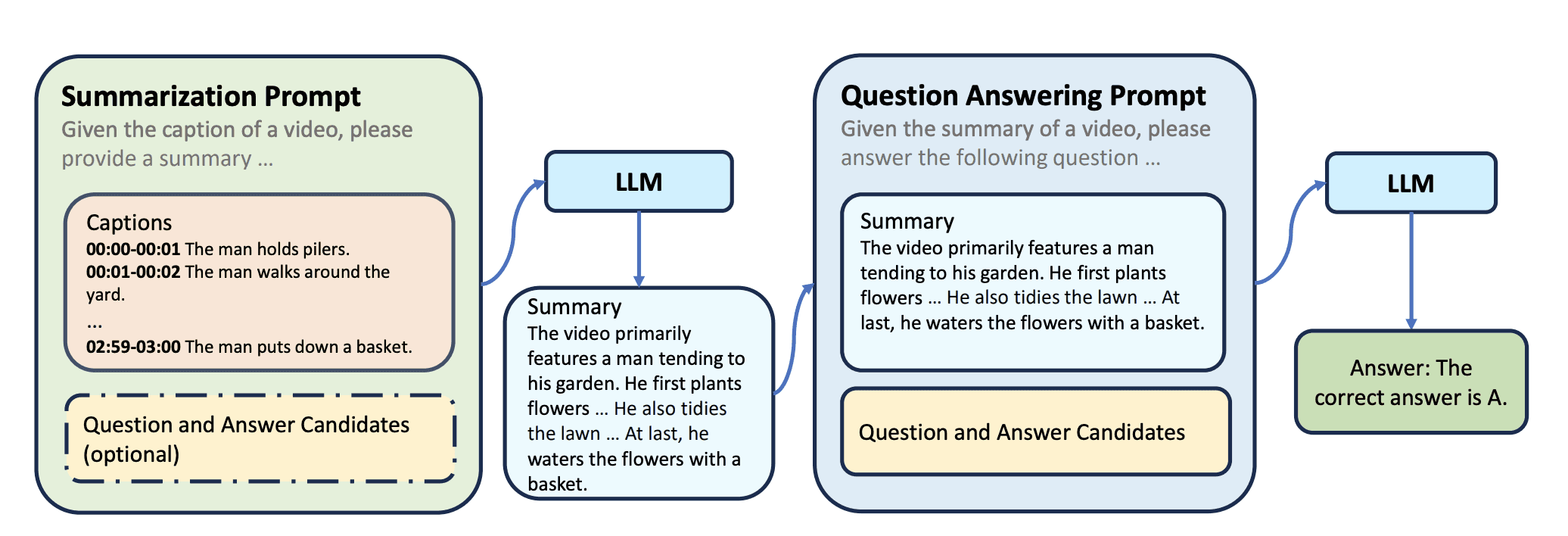
The evaluation results on EgoSchema demonstrated LLoVi's effectiveness in handling long-form video content, though Bertasius noted that the model uses 1750 times more parameters to model language than video, raising questions about efficiency and information preservation.
Beyond Language-Centric Approaches
Bertasius emphasized the limitations of representing videos purely through language, noting that captions and summaries dramatically reduce the rich information present in original video content.
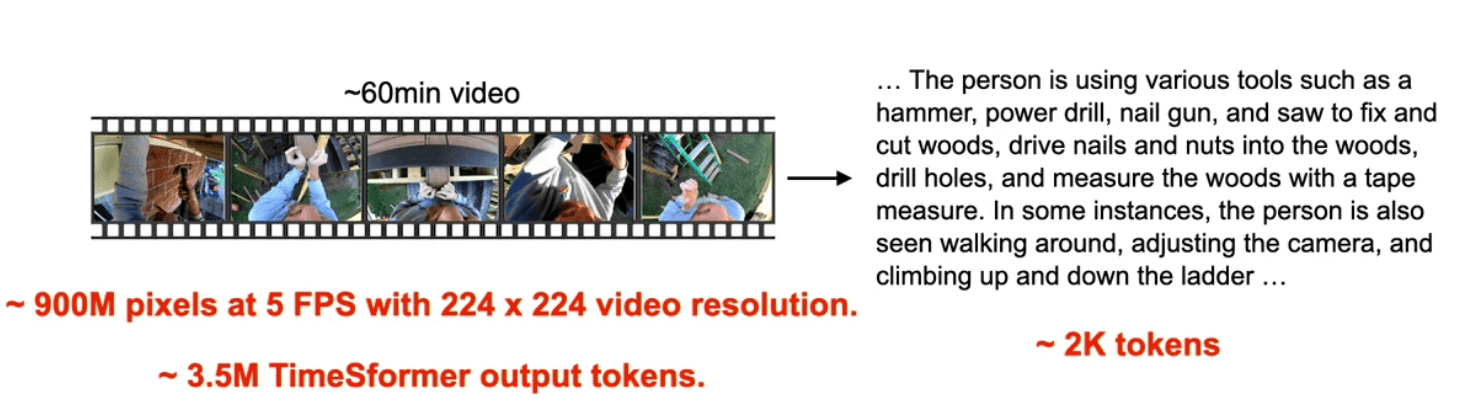
BASKET: Fine-Grained Skill Estimation Dataset
To address these limitations, the talk introduced BASKET, a comprehensive dataset for fine-grained skill estimation that focuses on visual understanding rather than language description. The dataset includes:
4,400+ hours of video content
32,232 players from 30+ countries across 4 continents
20 fine-grained basketball skills
8-10 minute highlight videos per player
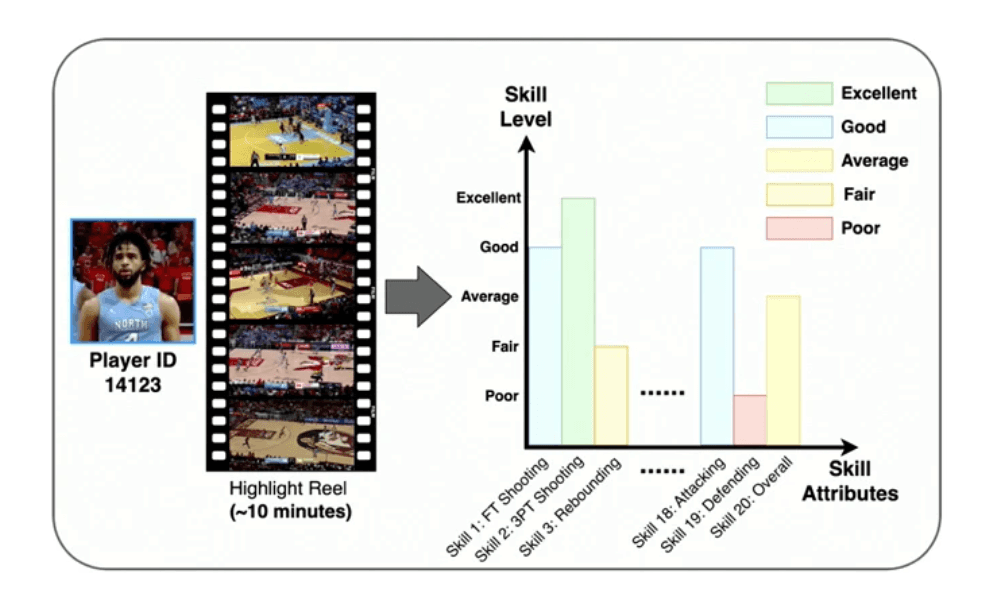
Basketball was selected as the focus sport due to several key advantages:
Global reach with 400M+ fans
High participant diversity
Abundant video footage availability
Complex fine-grained skills requiring temporal modeling
Similar player appearances necessitating fine-grained visual recognition
Performance Results:
Current state-of-the-art models struggle with fine-grained skill estimation
No model achieved more than 30% accuracy on the benchmark
Results highlight the significant gap between human and AI performance in complex video understanding tasks
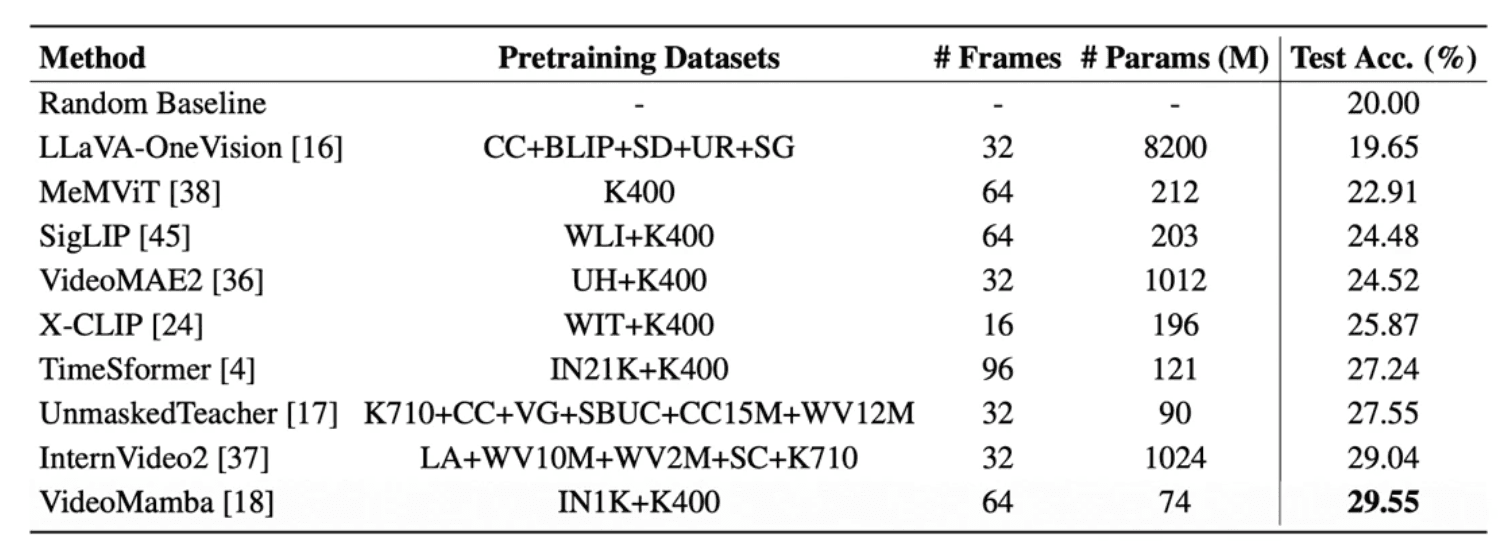
Key Takeaways and Future Directions
While LLMs have enabled remarkable progress in video understanding tasks, problems that are difficult to formulate using language don't benefit as much from language models or large-scale web video-text pre-training
The strong performance of LLMs and flaws in existing video-language benchmarks may mask underlying issues with video modeling
Current evaluation metrics may not fully capture the complexity of video understanding tasks
The field needs to develop more sophisticated approaches that can handle both language-based and purely visual understanding tasks
3 - How Intelligent Are Current Multimodal Video Models? - Yong Jae Lee
Professor Yong Jae Lee's talk challenged the conventional wisdom about the capabilities of current multimodal video models. While much of the research community has been focusing on understanding long-form videos, Lee emphasized a crucial point: understanding even short videos (less than 10 seconds) with counterfactual temporal information remains a significant challenge. This observation led to the development of two major contributions: Vinoground and Matryoshka Multimodal Models.
Vinoground: A New Benchmark for Temporal Reasoning
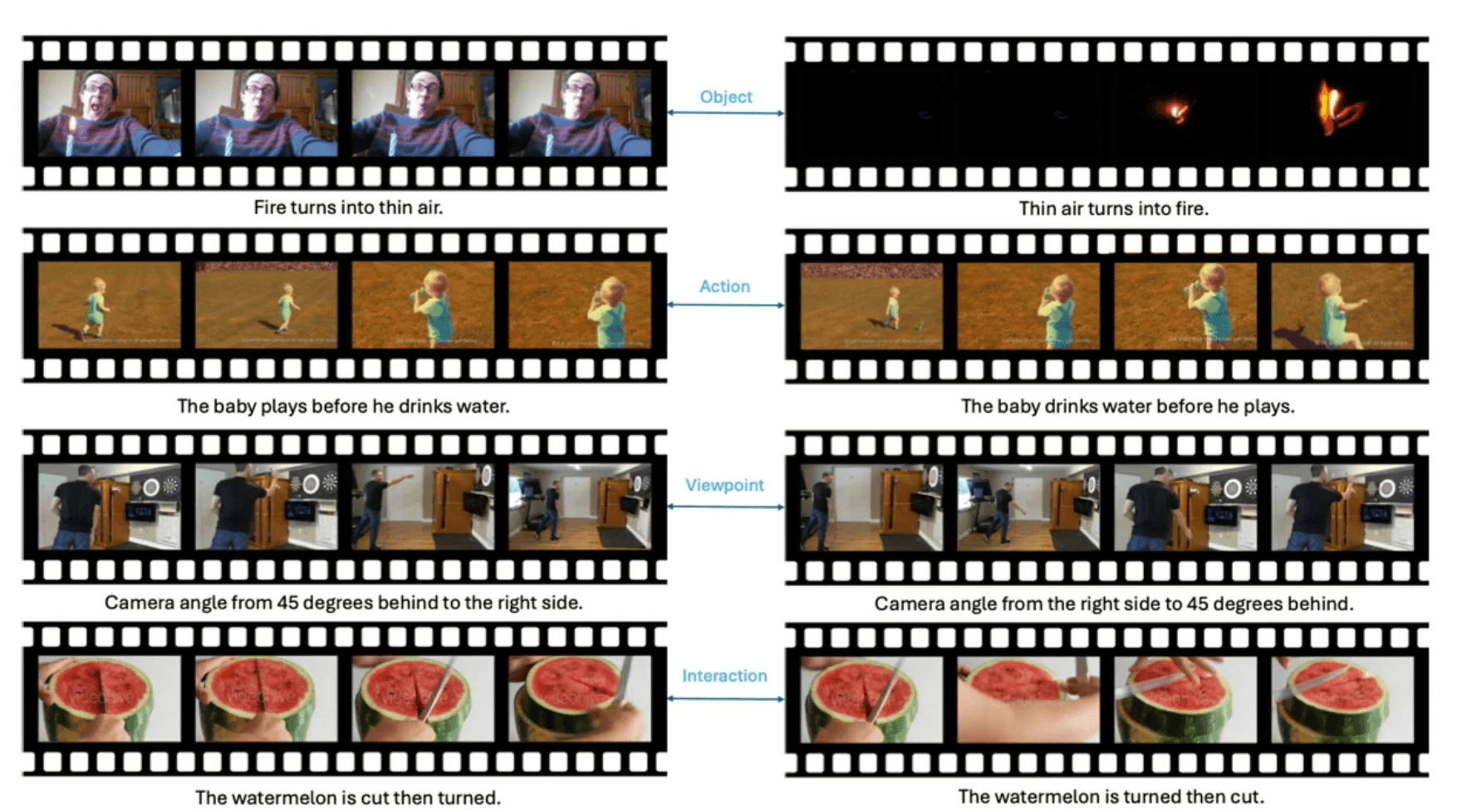
Inspired by Winoground, a challenging counterfactual benchmark for visio-linguistic compositional reasoning in images, Vinoground extends this concept to the video domain. The benchmark's name cleverly changes the 'W' to a 'V' for "video" while maintaining the focus on temporal counterfactuals as a unique element in video data.
The benchmark was designed with several crucial requirements to ensure robust evaluation of video understanding capabilities:
Videos must not contain single frame bias that could allow models to "cheat" by looking at individual frames
Tasks cannot be solved through textual cues alone, requiring true visual understanding
All videos must be natural, avoiding synthetic or artificially constructed scenarios
Complete video comprehension is necessary, with particular emphasis on event ordering
To make the benchmark especially challenging, each video pair comes with corresponding captions that use identical words but in different orders. For example, "a man waves at a woman before he talks to her" versus "a man talks to a woman before he waves at her." This design ensures that models must truly understand the temporal sequence of events rather than relying on simple pattern matching.
Technical Implementation
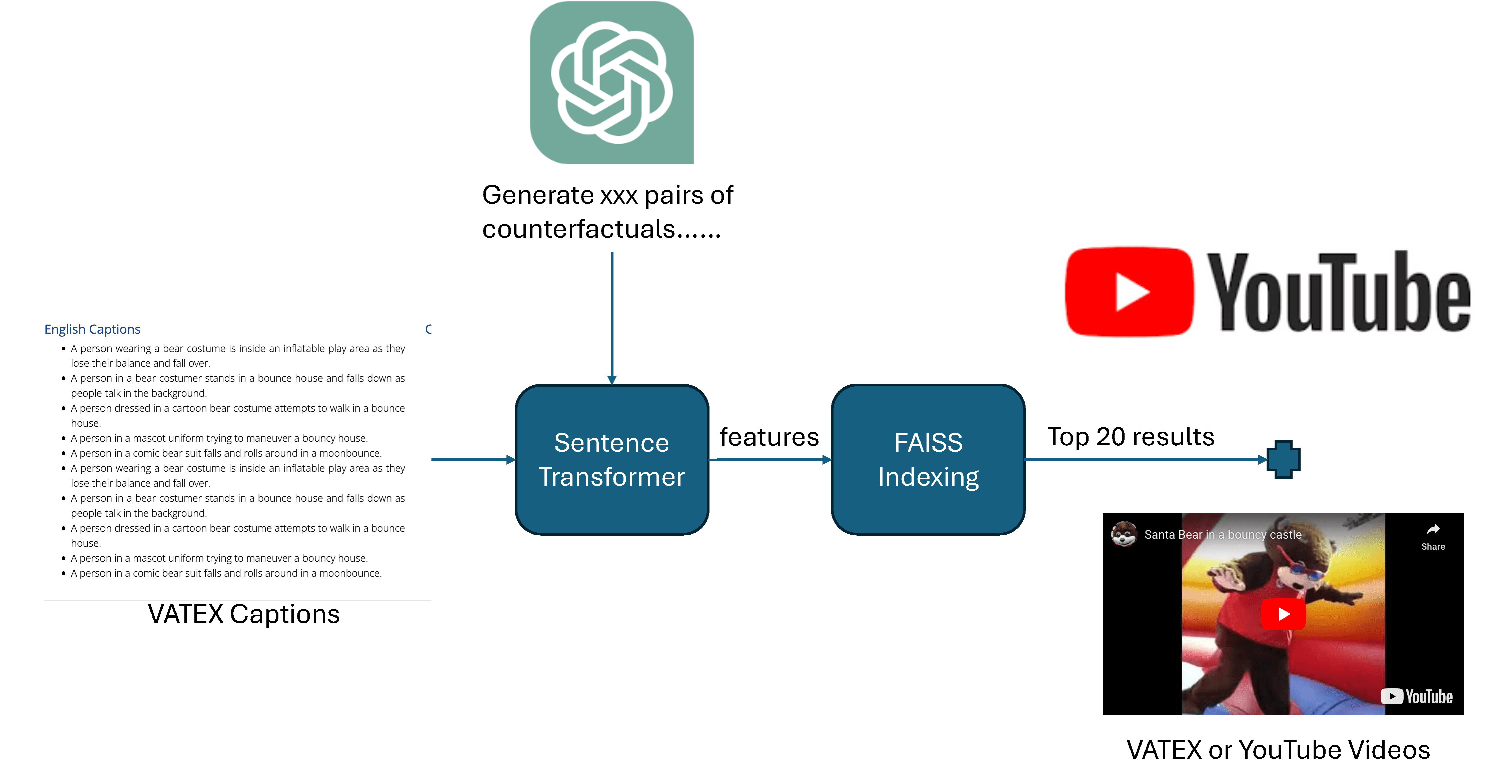
The data curation process involves a sophisticated pipeline where GPT-4 first generates counterfactual caption pair candidates. These candidates are then matched with appropriate videos using VATEX captions as an index, leveraging sentence transformers and the FAISS library for efficient similarity search. When direct matches aren't found in existing datasets, the team conducts YouTube searches using the captions to find suitable videos.
The benchmark comprises 500 carefully curated video-caption pairs, featuring:
Temporal differences in actions and objects (e.g., "the man eats then watches TV" vs. "the man watches TV then eats")
Object and viewpoint transformations (e.g., "water turning into ice" vs. "ice turning into water")
Spatial transformations that test understanding of physical relationships
Comprehensive Evaluation Framework
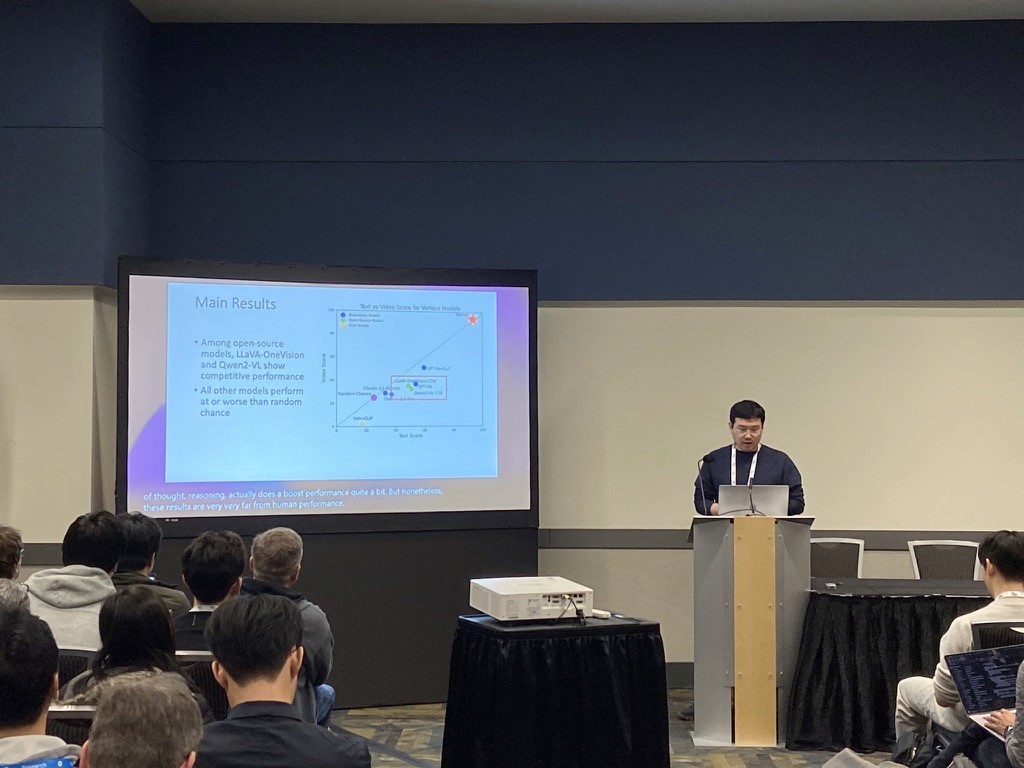
The evaluation framework employs three distinct metrics to provide a balanced assessment:
Text score: Measures the model's ability to understand linguistic differences
Video score: Evaluates visual comprehension capabilities
Group score: Assesses overall temporal understanding
Matryoshka Multimodal Models (M3)
The second major contribution addresses a fundamental bottleneck in current multimodal models: the overwhelming number of tokens, which not only makes Language-Vision Models (LMMs) inefficient but also dilutes their attention to relevant information. The Matryoshka Multimodal Models (M3) presents an elegant solution to this challenge.
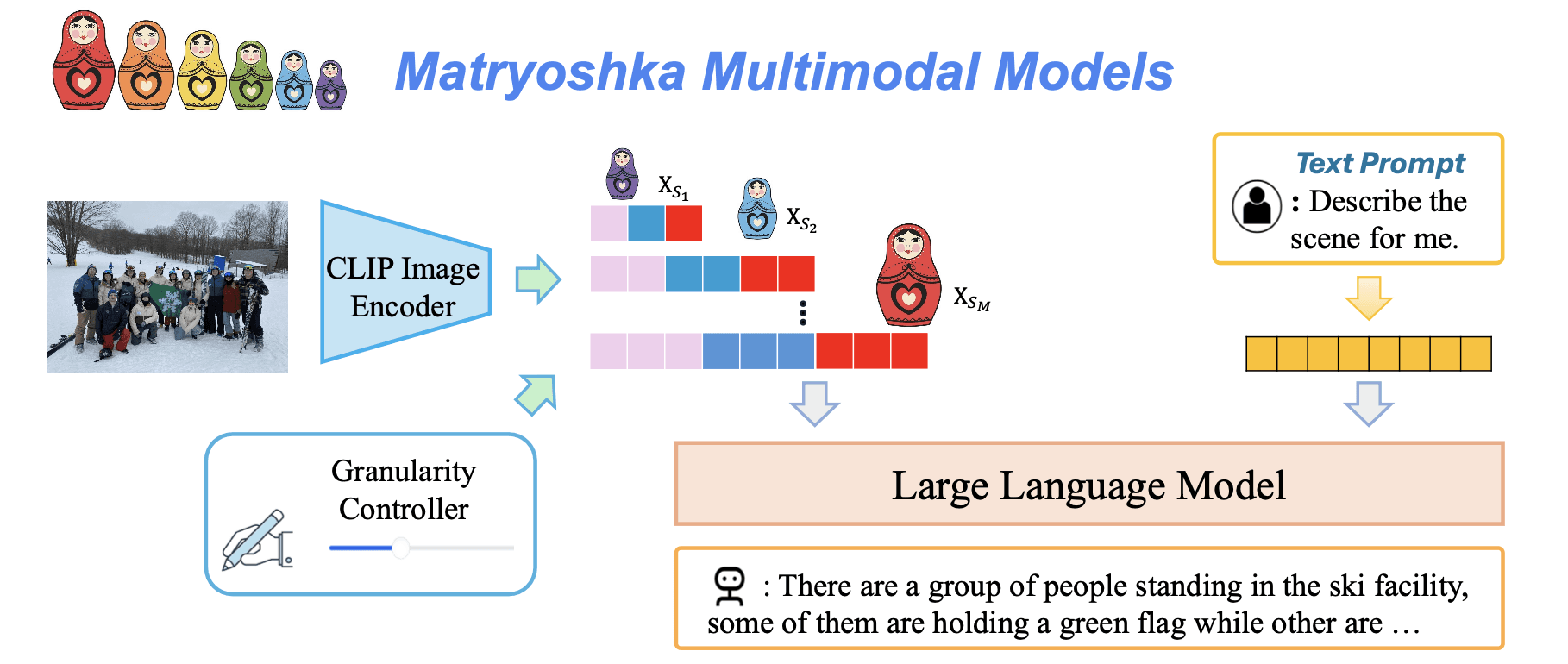
Technical Architecture
M3's training methodology is remarkably straightforward, taking the average of language generation loss across various visual token scales. The approach uses average pooling to obtain multi-granularity visual tokens, enabling a nested representation that proceeds from coarse to fine details.
Implementation Benefits
The implementation offers several significant advantages:
First, it maintains a simple design that uses vanilla LMM architecture and training data, requiring minimal code modifications and eliminating the need for a visual scale encoding module. Second, it provides unprecedented controllability, allowing researchers to explicitly adjust visual granularity during inference on a per-instance basis. Third, it serves as an analytical tool for understanding dataset requirements, revealing that many COCO-style benchmarks need only around 9 visual tokens to achieve performance comparable to using all 576 tokens.
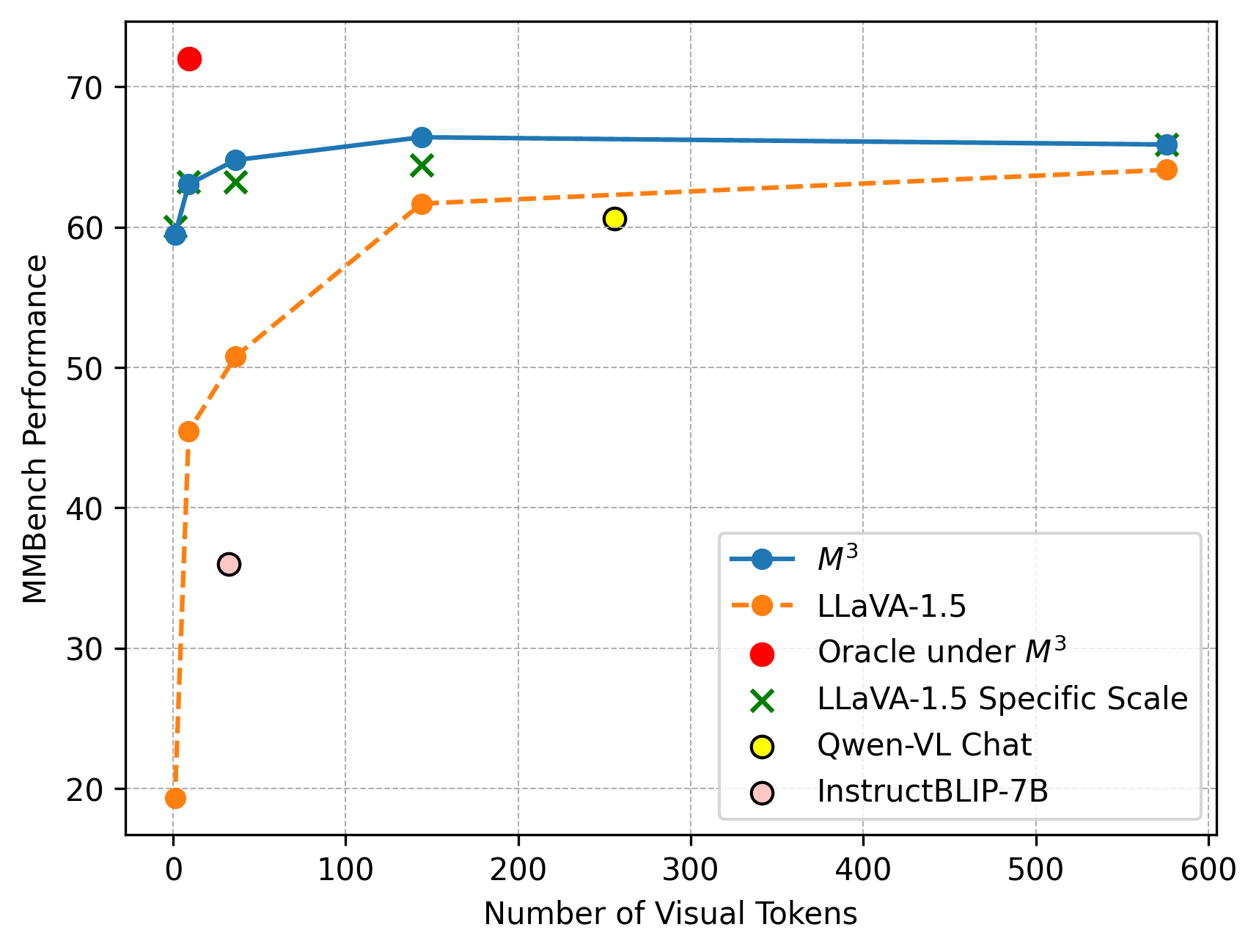
Empirical Results
Extensive testing on the MMBench revealed several crucial findings. The M3 variant of LLaVA-1.5-7B maintains or exceeds the performance of models trained on specific scales, while using significantly fewer resources. Particularly noteworthy is that M3 with just 9 visual tokens outperforms established baselines like InstructBLIP and Qwen-VL.
In video understanding tasks, full visual tokens often proved counterproductive. On four out of six benchmarks, using 720 or 180 tokens showed better performance than using the full token set, suggesting that excessive visual context might actually distract from accurate predictions. Most remarkably, for tasks like ActivityNet, IntentQA, and EgoSchema, using just 9 tokens per image grid (45 tokens total) performed within 1% accuracy of using the full 2880 tokens.
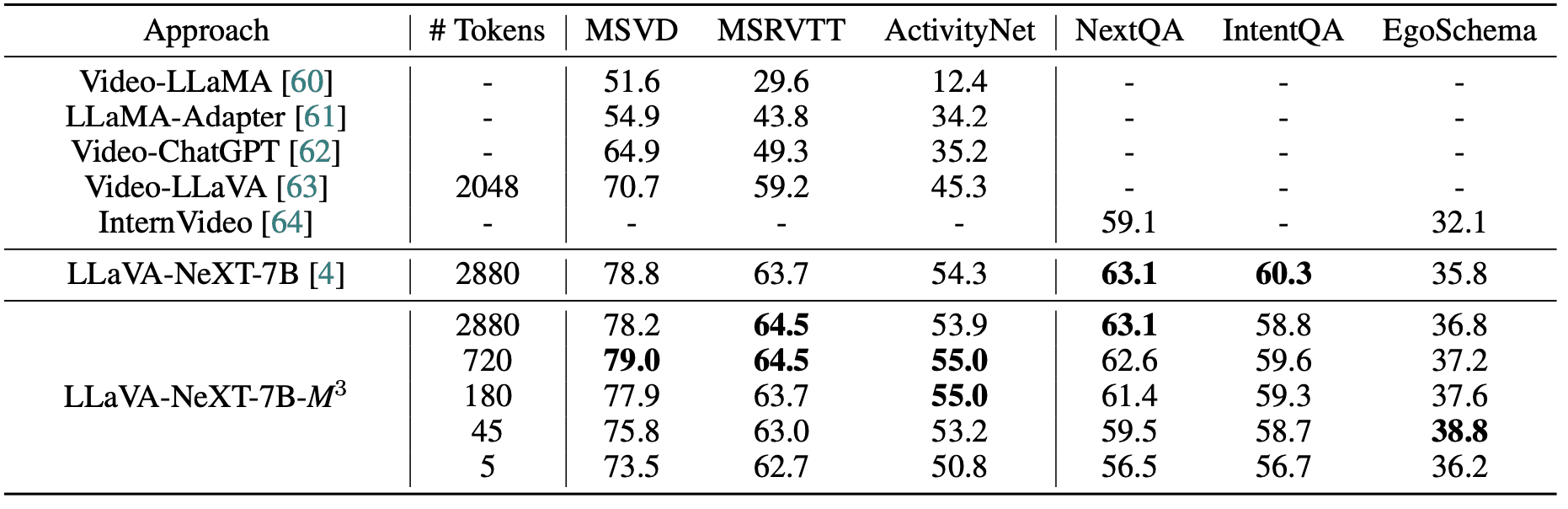
Broader Implications and Future Directions
The research presents several critical insights about the current state of video understanding models. While they show impressive capabilities in certain areas, they still fall significantly short of human-level intelligence. The Vinoground results particularly highlight how even short counterfactual temporal understanding remains challenging for current state-of-the-art models. Meanwhile, the M3 findings suggest that efficient processing of visual information might be more important than processing more visual tokens, pointing toward a promising direction for future model development.
4 - MovieGen: Foundation Model for Video Generation - Ishan Misra
Ishan Misra's presentation detailed Meta's MovieGen project, a groundbreaking initiative in text-to-video generation. The project addresses the fundamental challenge of generating videos from text input, with applications spanning creative content creation, video editing, image animation, simulation, and tools for both social media creators and Hollywood productions.
Evolution of Video Generation
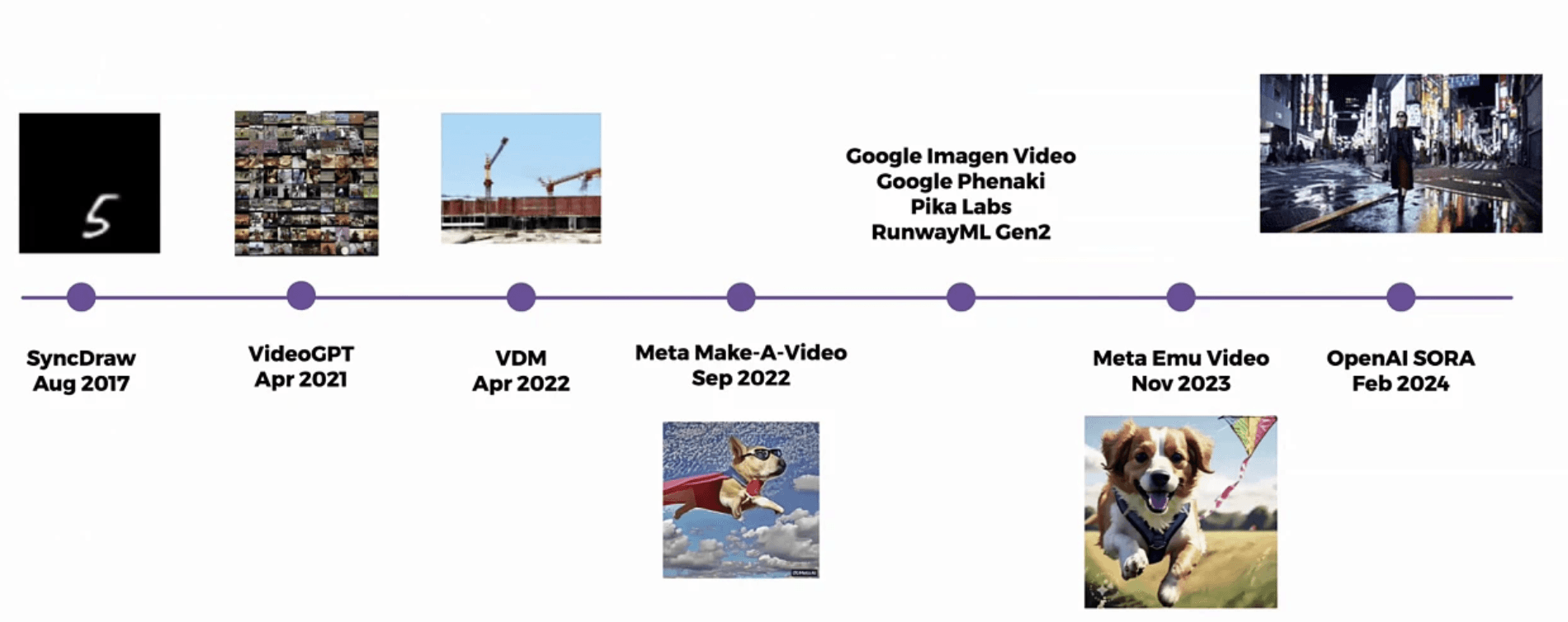
The presentation began with a comprehensive timeline of video generation models, highlighting the rapid progress in the field and setting the stage for MovieGen's contributions. This historical context emphasizes how the field has evolved from basic video synthesis to increasingly sophisticated generation capabilities.
Core Challenges in Video Generation
The field of video generation faces several fundamental challenges that MovieGen seeks to address. The first is the complex transformation from low-dimensional text input to high-dimensional video output, requiring sophisticated architectural solutions. Second, maintaining visual consistency and quality throughout generated videos demands robust temporal modeling. Third, the computational complexity of video generation necessitates efficient processing strategies and optimized architectures.
Technical Architecture and Innovations
1. Architectural Framework
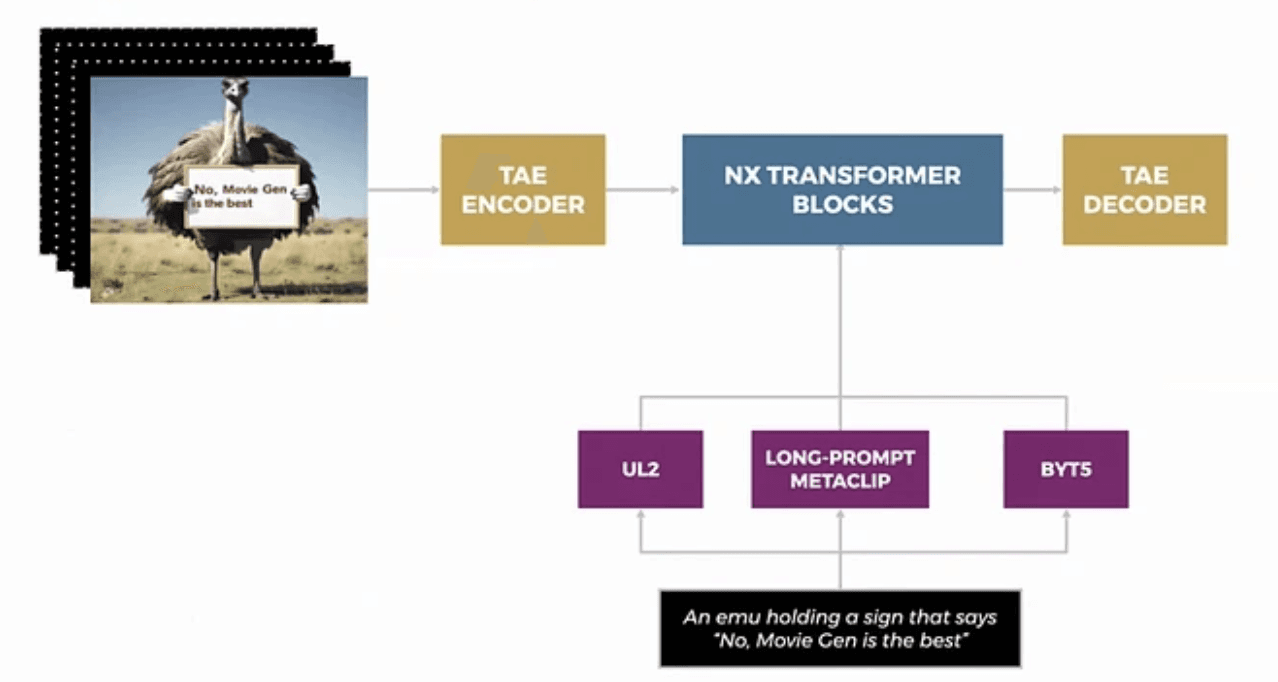
MovieGen's architecture represents a significant advancement in video generation, incorporating several innovative elements. The model employs enhanced text encodings that capture multiple aspects of input text prompts, enabling more nuanced understanding of generation requirements. The architecture is built on LLaMA-style Transformer blocks, which have been specifically adapted for video generation tasks, providing improved scalability and stability during training.
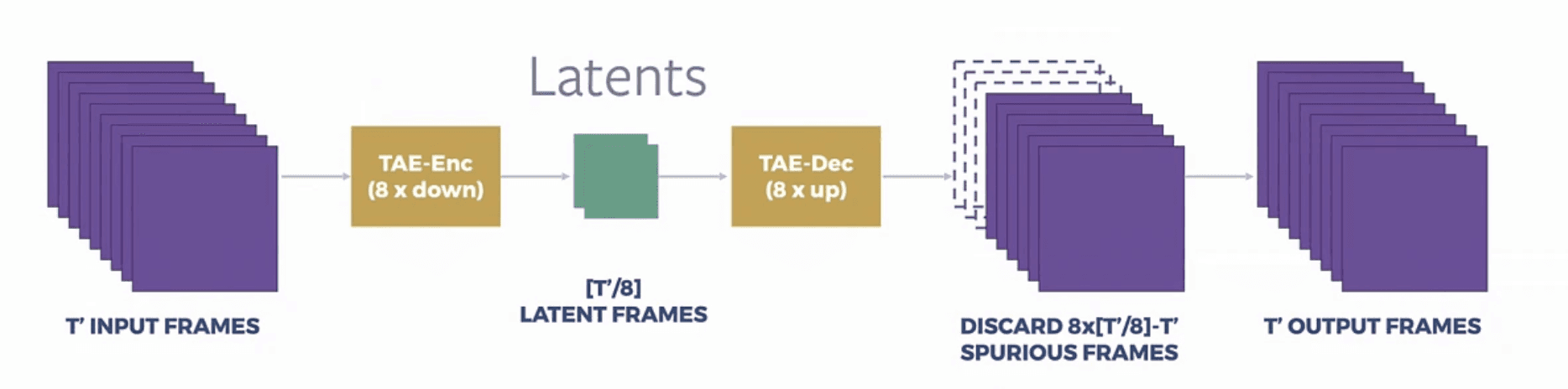
A key innovation is the model's approach to latent compression. MovieGen achieves an 8x reduction in video dimensions across height, width, and time dimensions, resulting in a remarkable 512x reduction in sequence length. This compression capability extends to both videos and images, allowing for variable-length sequence processing while maintaining generation quality.
2. Training Methodology
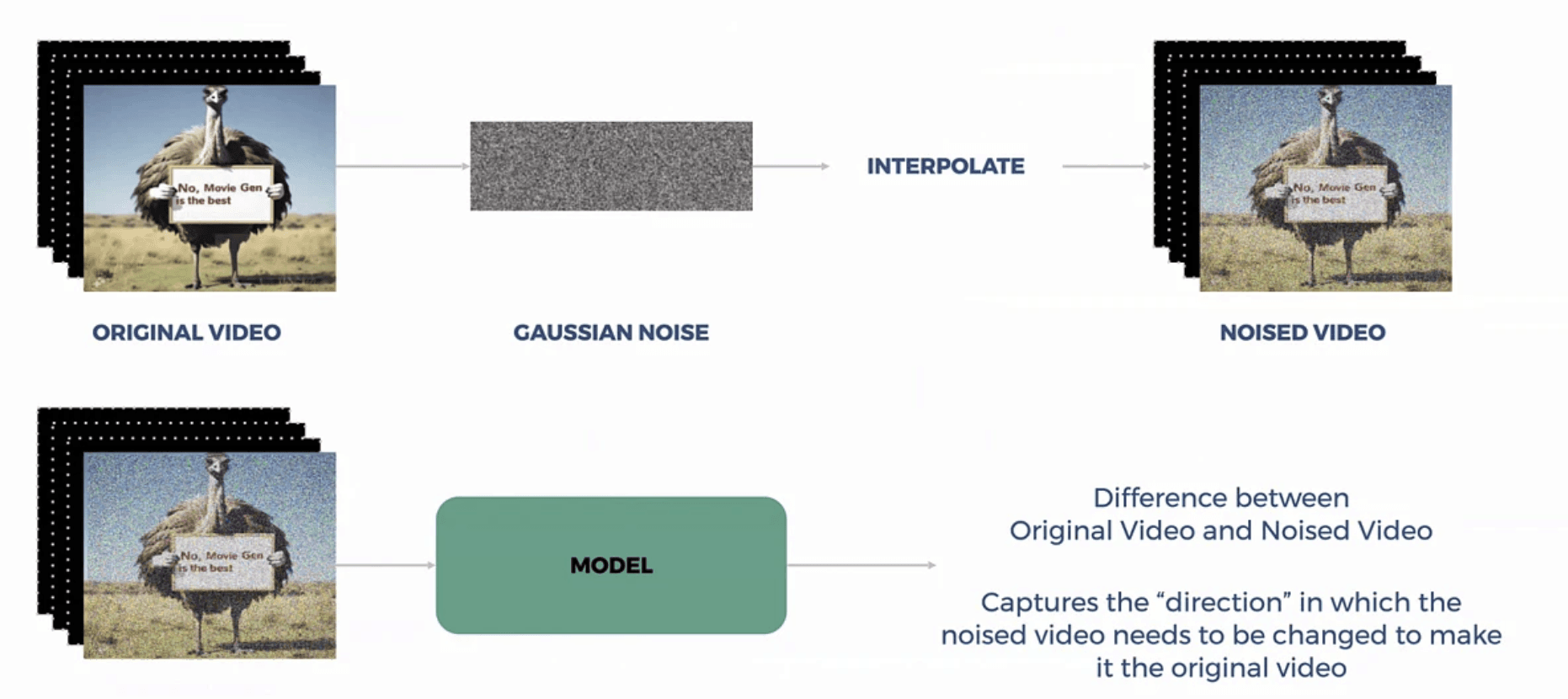
The training approach incorporates LLaMA-style blocks enhanced with diffusion Transformer (DiT) scale/shift modifications. This combination enables stable training at scale while allowing all parameters to be trained simultaneously on both images and videos, eliminating the need for frozen components. This end-to-end training approach contributes to the model's coherent understanding of both static and dynamic visual content.
3. Flow Matching Innovation
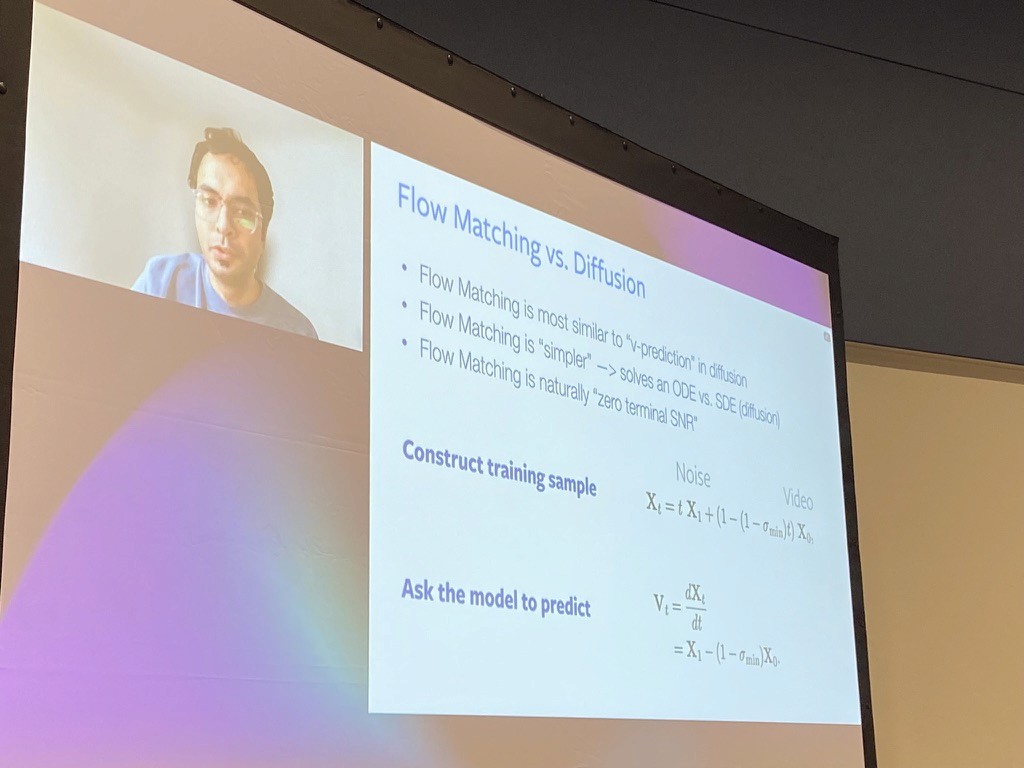
One of MovieGen's most significant technical innovations is its implementation of flow matching as an alternative to traditional diffusion approaches. This method is mathematically similar to "v-prediction" in diffusion but offers several crucial advantages. Instead of solving a stochastic differential equation (SDE) as in diffusion models, flow matching solves an ordinary differential equation (ODE), resulting in a simpler and more efficient process. The approach naturally achieves "zero terminal Signal-to-Noise ratio" characteristics, leading to both improved generation quality and faster inference speeds compared to diffusion-based methods.
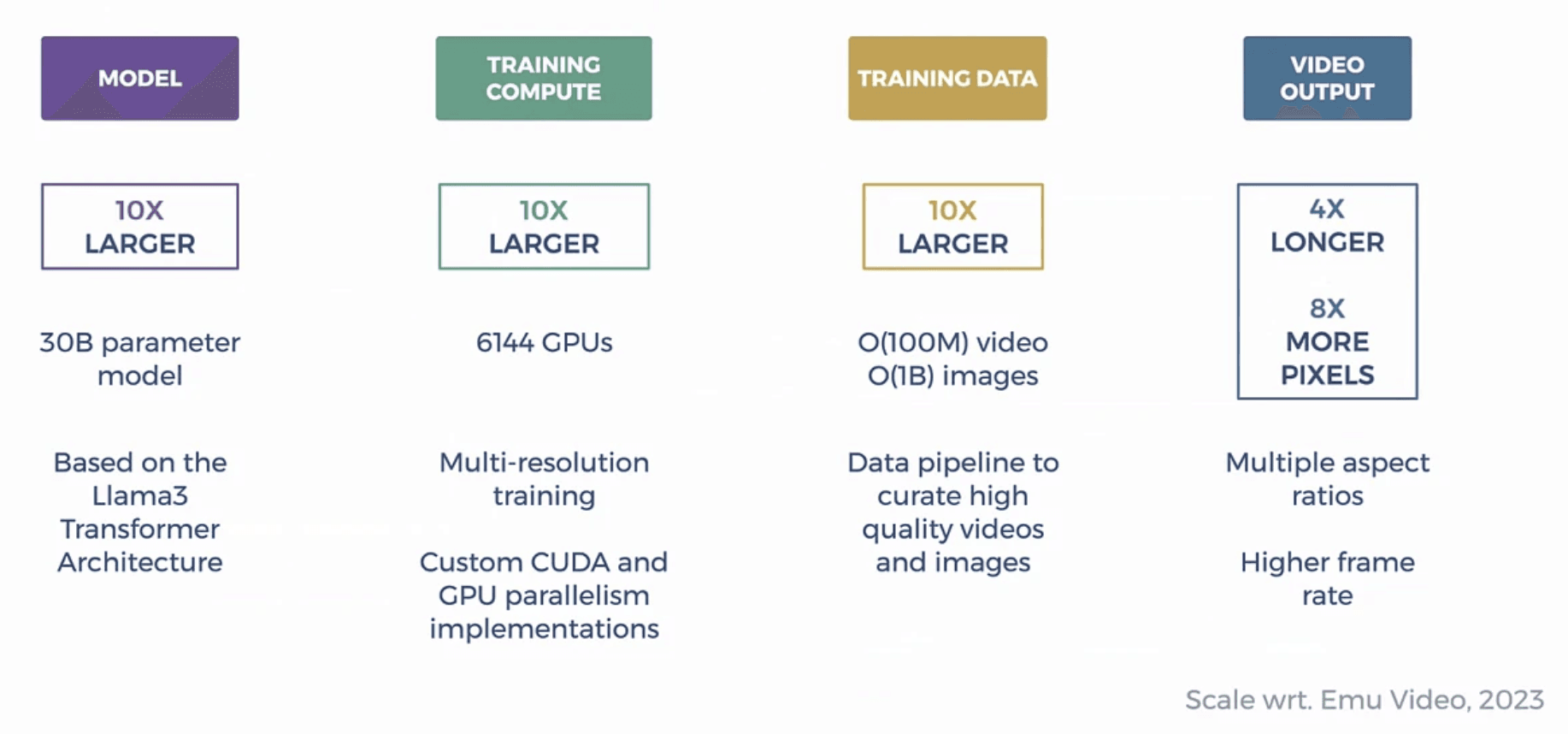
Training Data and Scaling
MovieGen's training methodology represents a significant scaling effort in both data and computation. The training dataset encompasses approximately 100 million videos and 1 billion images, with videos varying in length and frame rate to ensure robust generalization. A notable innovation is the use of LLaMA-3 for video caption generation, which substantially improves the model's ability to follow text prompts accurately.
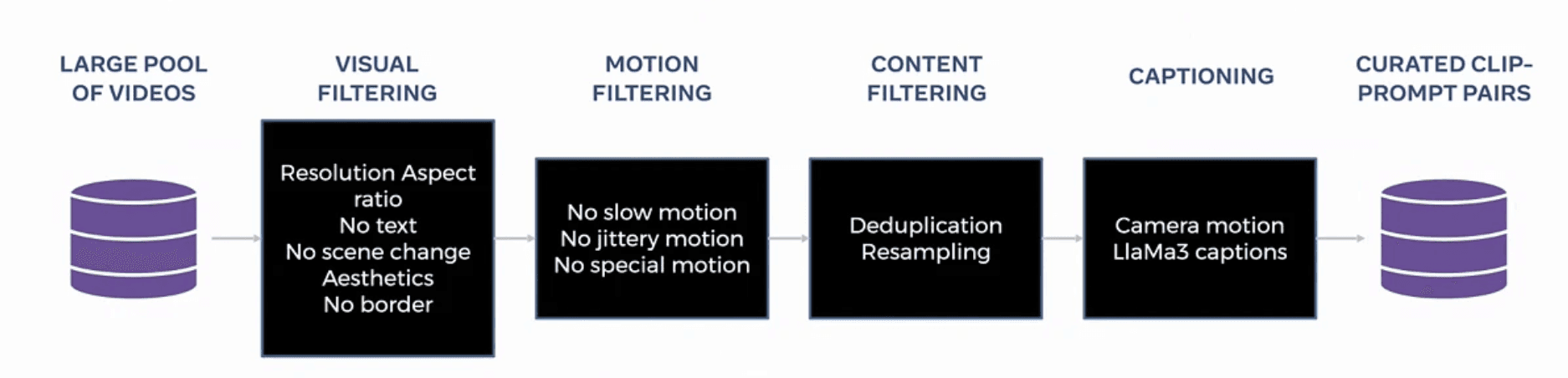
Comprehensive Evaluation Framework
The Meta team developed MovieGen Bench, a rigorous evaluation framework that surpasses previous benchmarks in both scale and comprehensiveness. This benchmark includes 1,000 non-cherry-picked videos, available publicly on GitHub, and offers three times more evaluation data than previous benchmarks. The evaluation methodology demonstrated a strong correlation between validation loss and human judgment, providing a reliable metric for model performance.
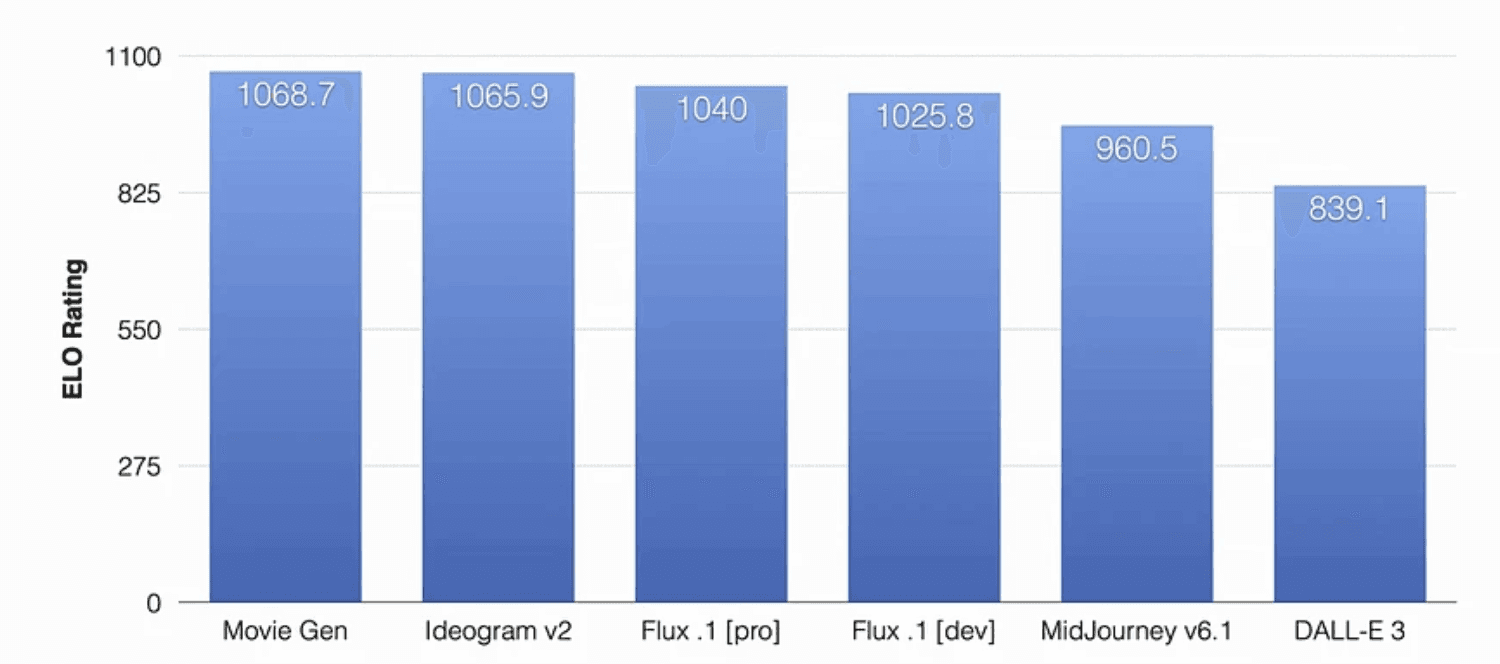
Performance and Future Directions
The evaluation results revealed impressive capabilities in several key areas. MovieGen showed competitive performance in both overall quality and realness metrics, with particularly strong results in text-to-image generation capabilities. The research uncovered important insights about scaling laws, notably that model performance has not yet reached saturation and that video generation scaling laws closely mirror those observed in LLaMA-3.
While MovieGen represents a significant advance in video generation capabilities, Ishan acknowledges important limitations and future challenges. Particularly, the extent of the model's ability to combine and generalize its understanding of the world remains an active area of investigation. This honest assessment of current limitations helps guide future research directions in the field of video generation.
5 - Towards Multimodal Agentic Models - Jianwei Yang
Jianwei Yang's presentation challenged the traditional focus on pure understanding in AI systems, arguing that interaction, not understanding alone, should be the ultimate goal. His comprehensive talk outlined a roadmap for developing multimodal AI agents capable of both understanding the past and acting for the future, with applications spanning web agents, robotics, and autonomous driving.
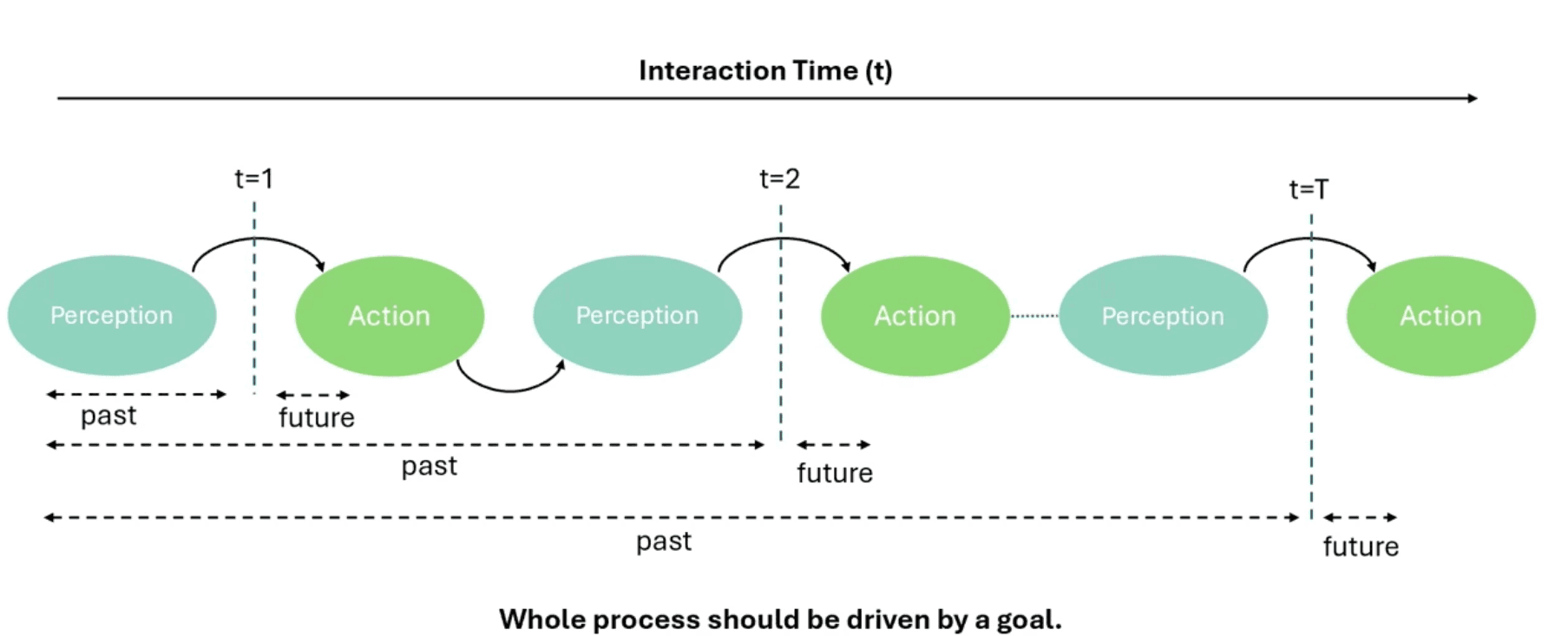
Understanding the Past: Enhanced Visual Perception
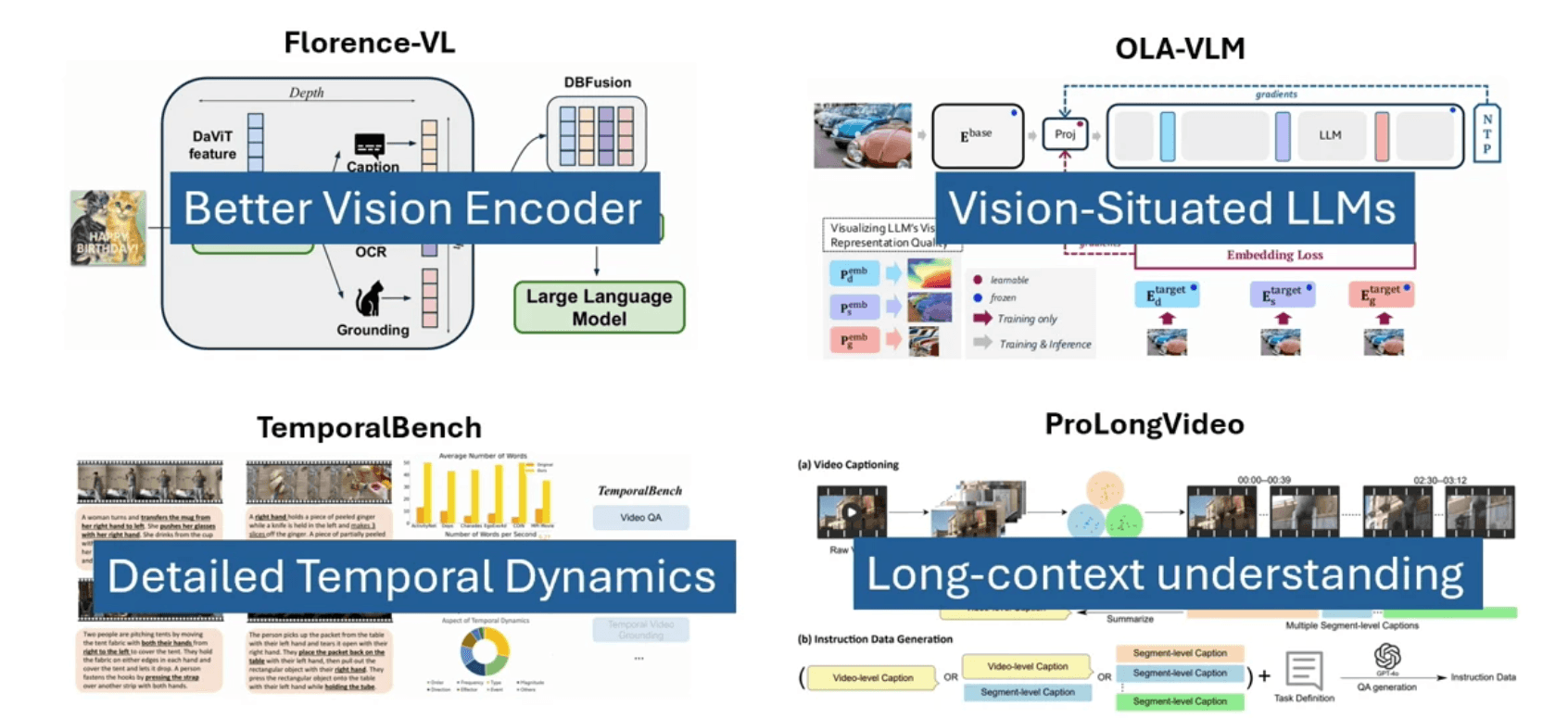
Florence-VL: Enhanced Vision-Language Models
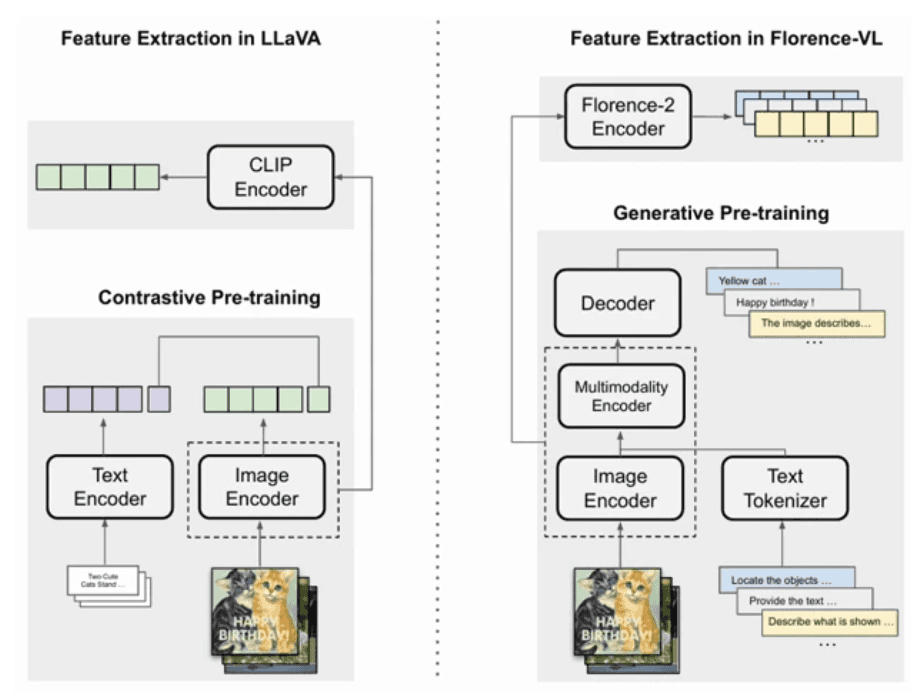
Florence-v2 represents a significant advancement in text-conditioned generative vision-language models. The model's architecture introduces a novel approach to visual perception through different prompts designed for various types of visual features. These features work complementarily, creating a richer overall representation of visual information. The model's effectiveness is measured through alignment cost between output features from the vision encoder and LLM encoder, providing a quantitative metric for evaluation.
OLA-VLM: Deepening Visual Understanding
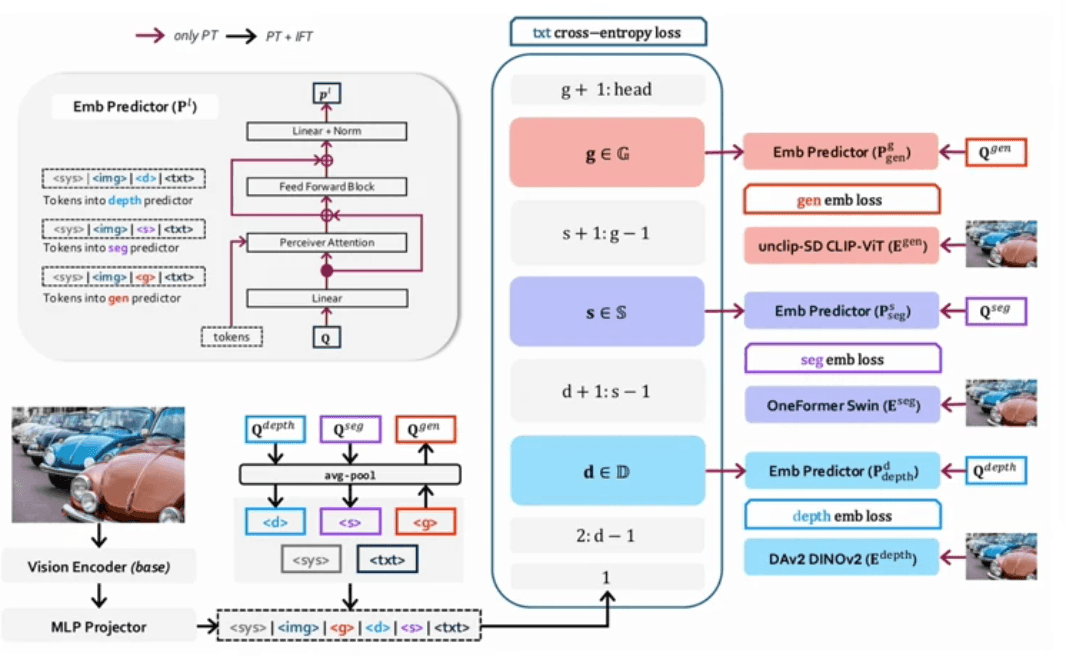
OLA-VLM pushes the boundaries of visual perception in multimodal LLMs by incorporating three distinct visual features for each image:
Depth features that capture spatial relationships
Segmentation features for object delineation
Unclip-SD features for enhanced visual representation
The research demonstrates that increasing text supervision leads to improved intermediate features for vision perception. A key innovation is the direct optimization of LLM features using "teacher" models, resulting in more robust visual understanding capabilities.
Fine-grained Temporal Dynamics
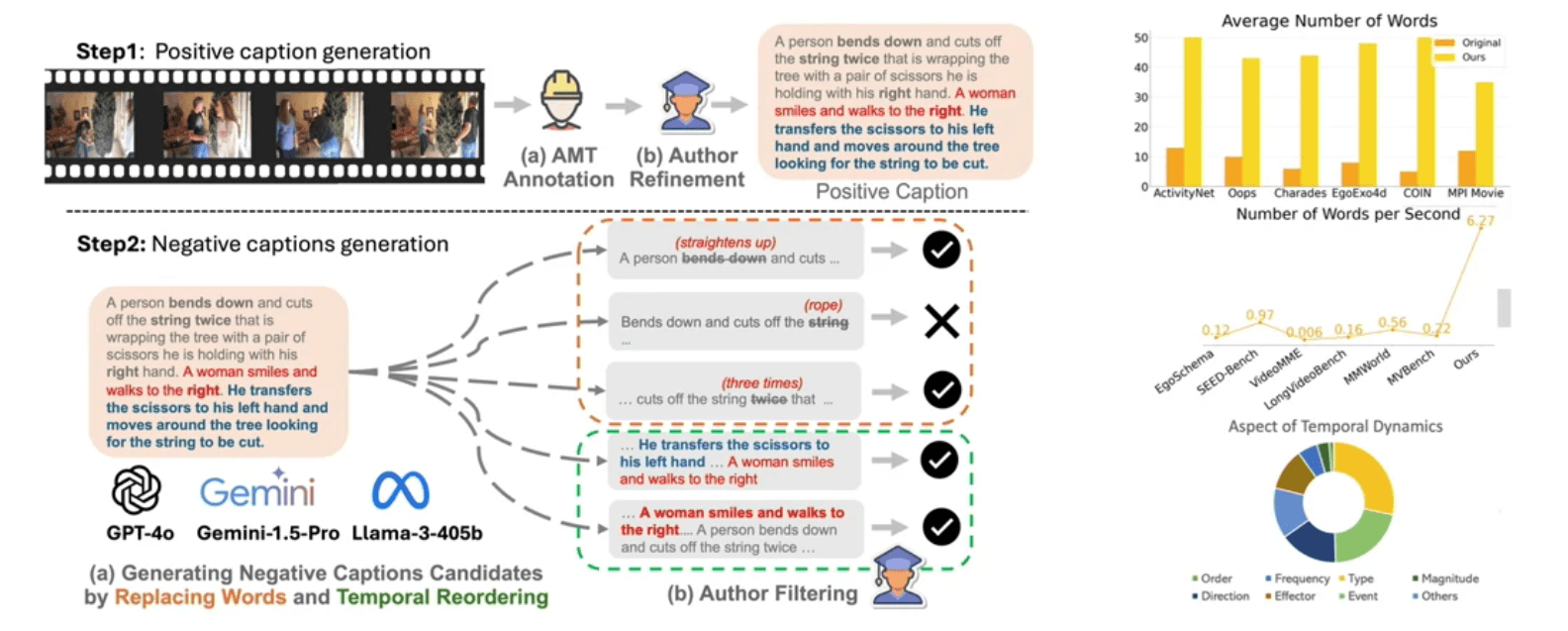
The TemporalBench project addresses a fundamental aspect of video understanding: videos contain not just static contents in individual frames but crucial fine-grained dynamics. This benchmark provides a comprehensive evaluation framework for assessing temporal understanding capabilities in multimodal video models.
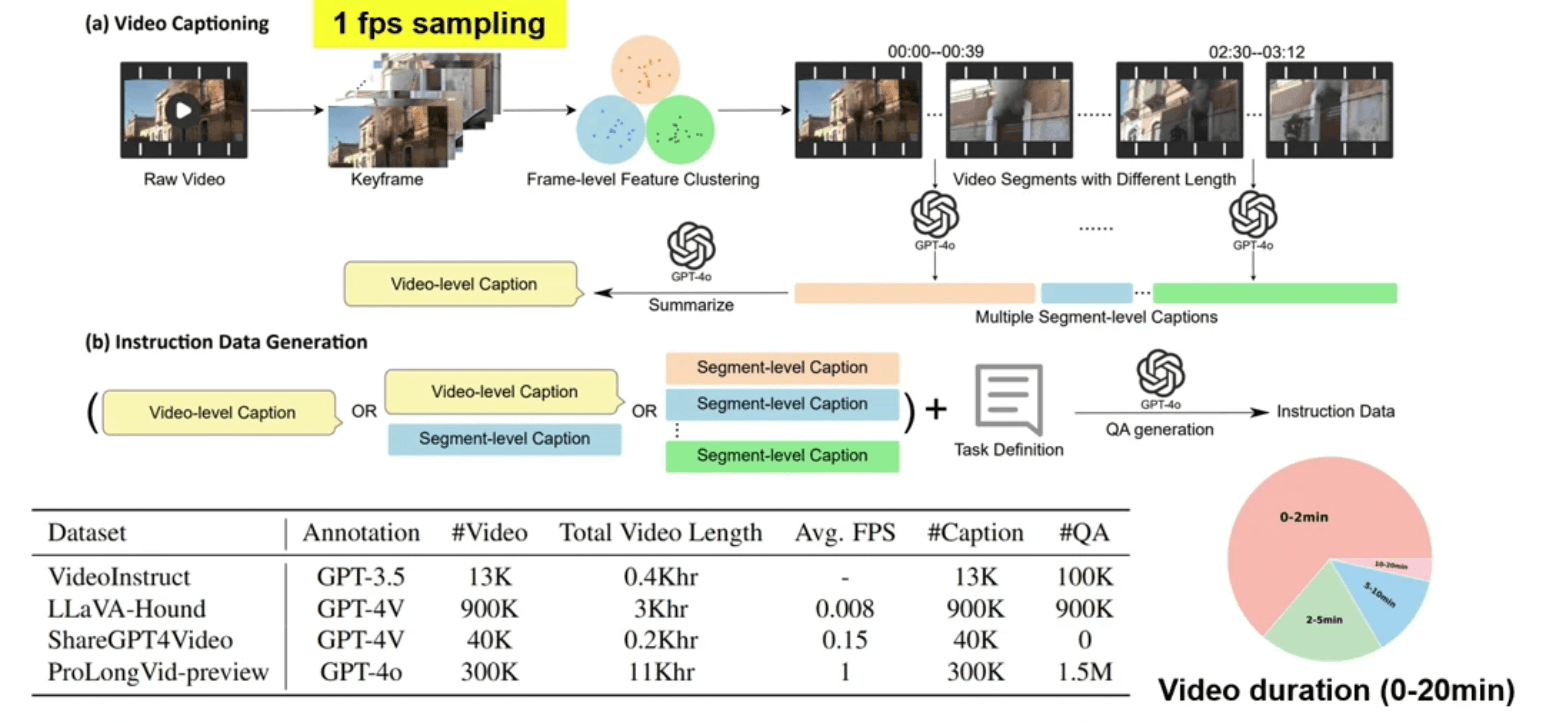
ProLongVid complements this work by focusing on two critical scaling dimensions:
Scaling video length during instruction data synthesis, enabling models to handle longer temporal sequences
Scaling context length during both training and inference, improving the model's ability to maintain coherent understanding across extended temporal spans
Acting for the Future: Multimodal Agentic Models
TraceVLA: Visual Trace Prompting
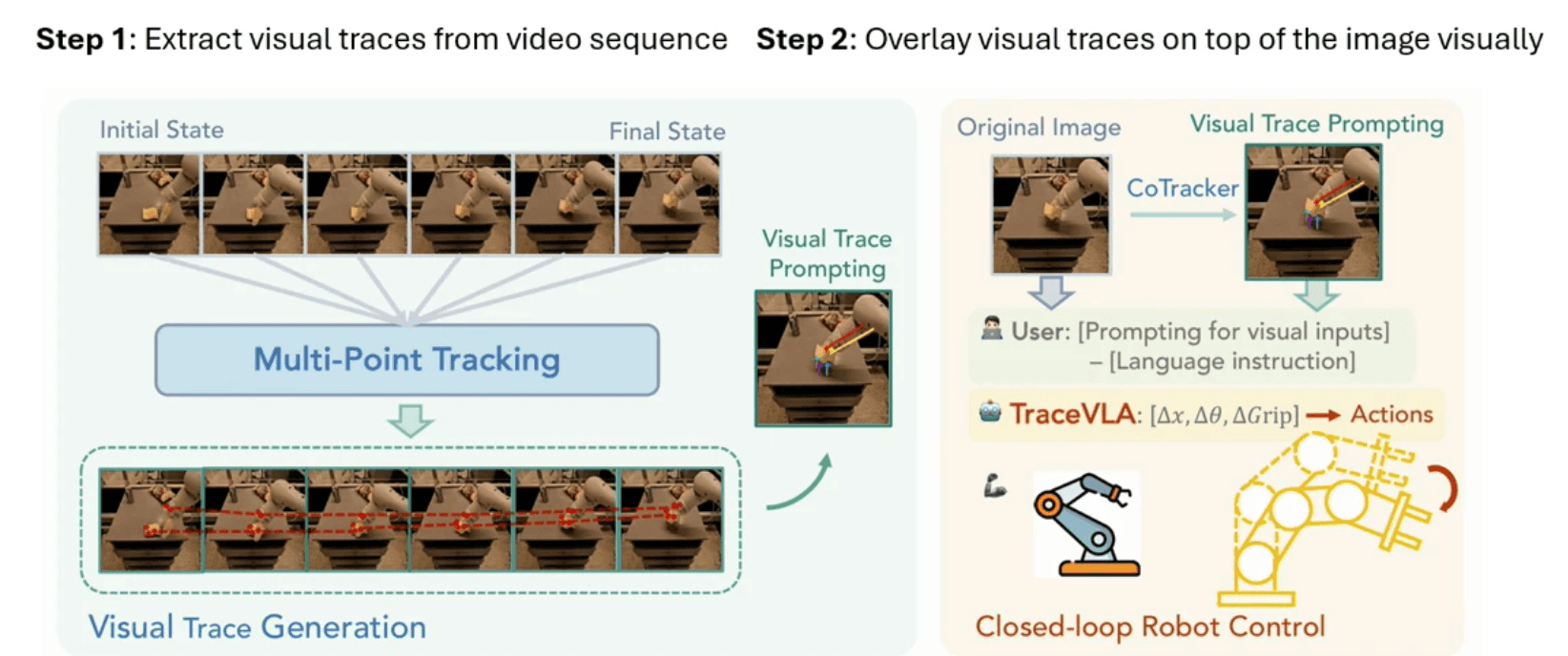
TraceVLA introduces a novel approach to enhancing spatial-temporal awareness for robotic policies. The core intuition behind this work is that better understanding of history facilitates better prediction of future actions. The system addresses several key challenges in multimodal processing:
Multi-image processing presents a significant challenge by increasing the number of visual tokens, while vision encoders struggle to capture subtle changes or motions across adjacent frames.
Text prompts, while easy to implement, fall short in capturing fine-grained movement information and spatial locations.
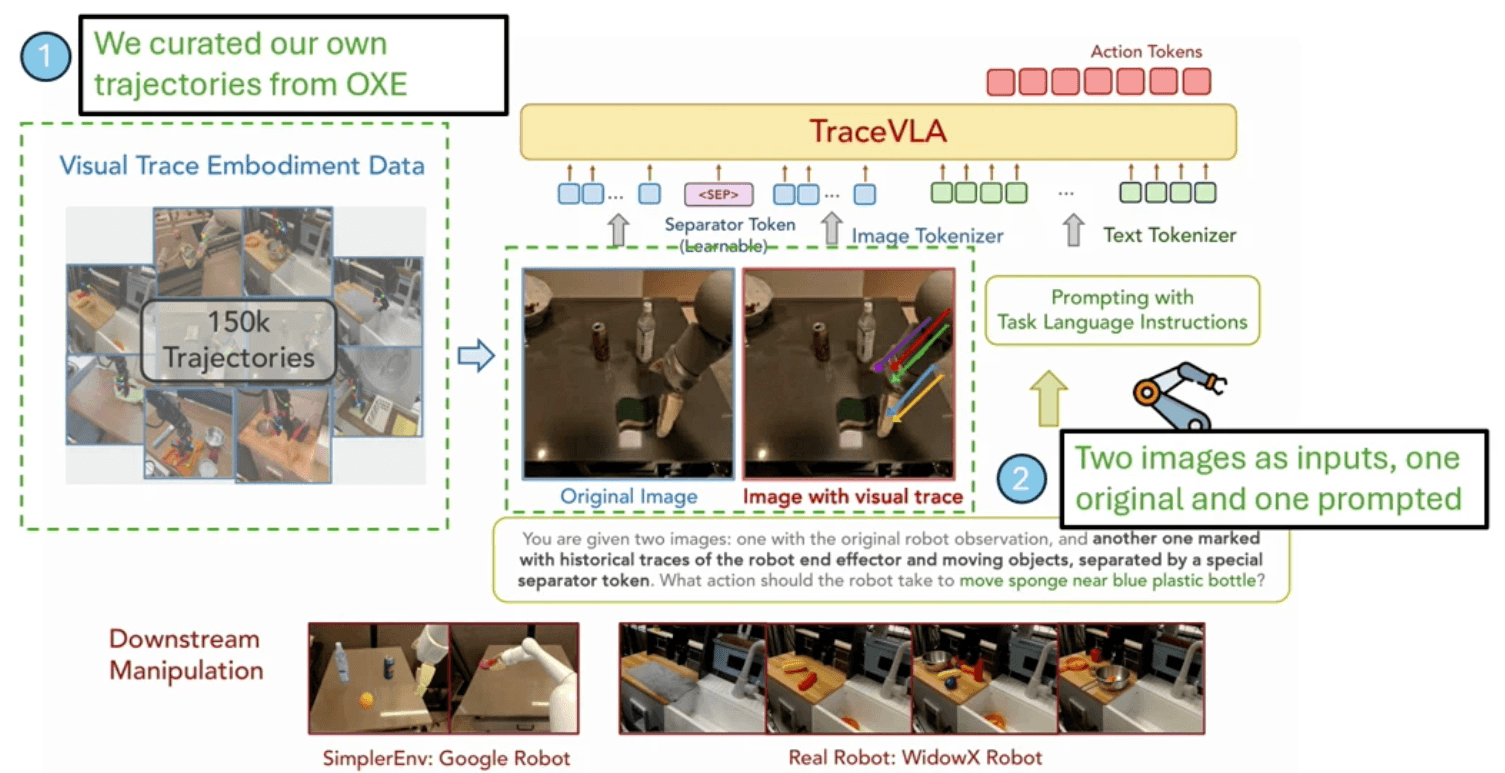
The innovation comes through visual prompts, which prove particularly effective through Set-of-Mark prompting, naturally building associations between current observations and historical context.
LAPA: Latent Action Pretraining
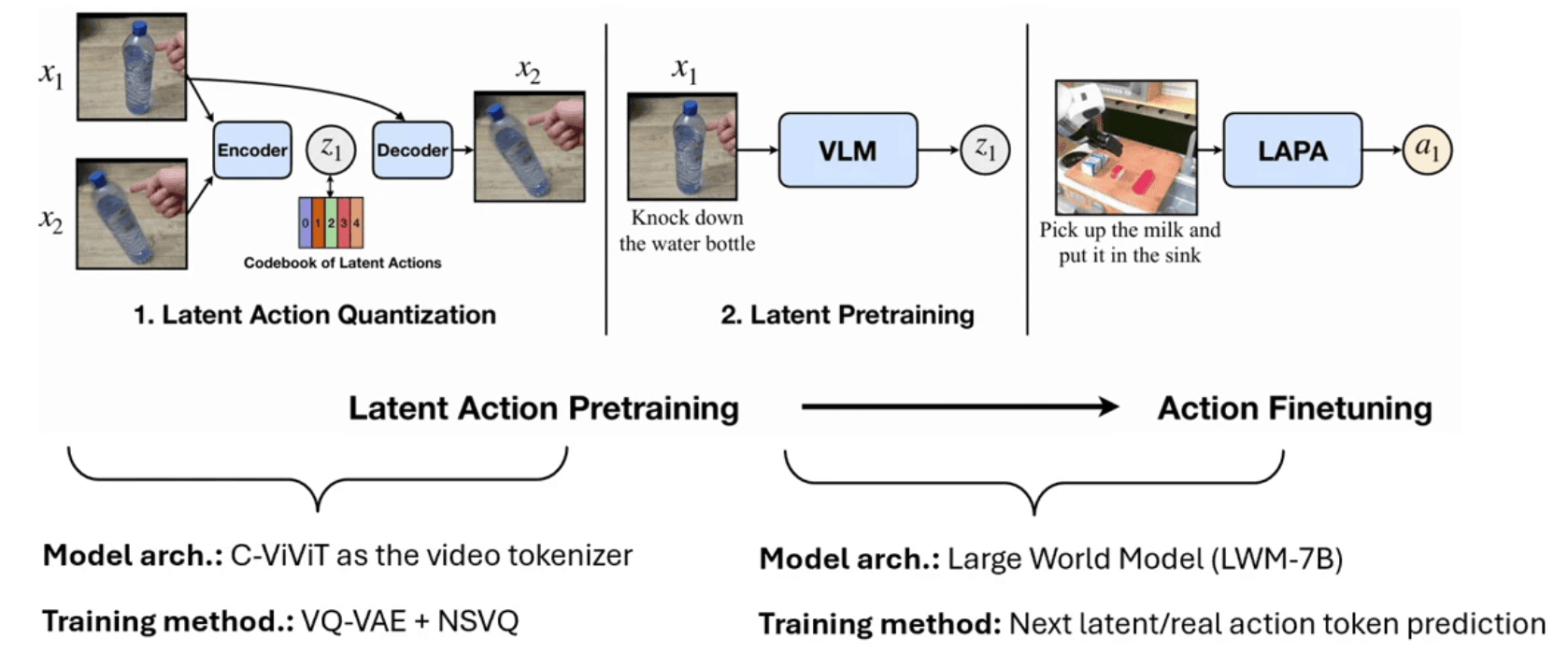
LAPA represents a breakthrough in leveraging unlabeled video data for robotics policy training. The system demonstrates impressive capabilities in learning from both robotics data without actions and human instructional videos. Using sthv2 as the human instructional video dataset, LAPA achieves significant improvements over models trained from scratch, though some gap remains compared to models pre-trained on robotics-specific data.
MAGMA: Foundation Model for Multimodal AI Agents
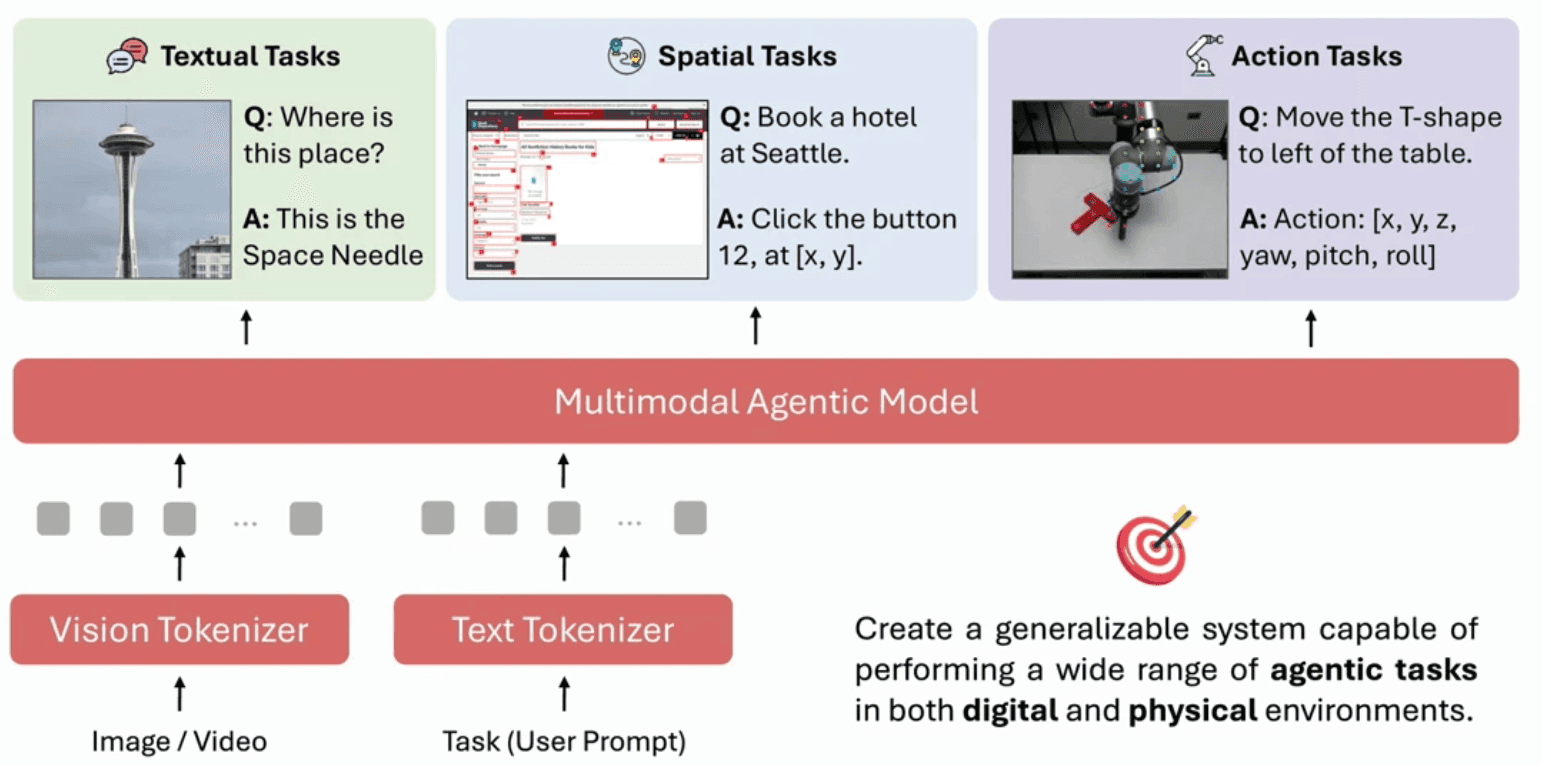
MAGMA introduces a comprehensive foundation model for multimodal AI agents, incorporating pre-training across a diverse range of images, videos, and robotics data. The model architecture is designed to facilitate both understanding and action, with particular emphasis on temporal motion supervision and action conversion. The pre-training tasks are carefully designed to develop emergent capabilities in robot trajectory planning, demonstrating effects of data scaling and task generalization.
Redefining Multimodal AI Agents
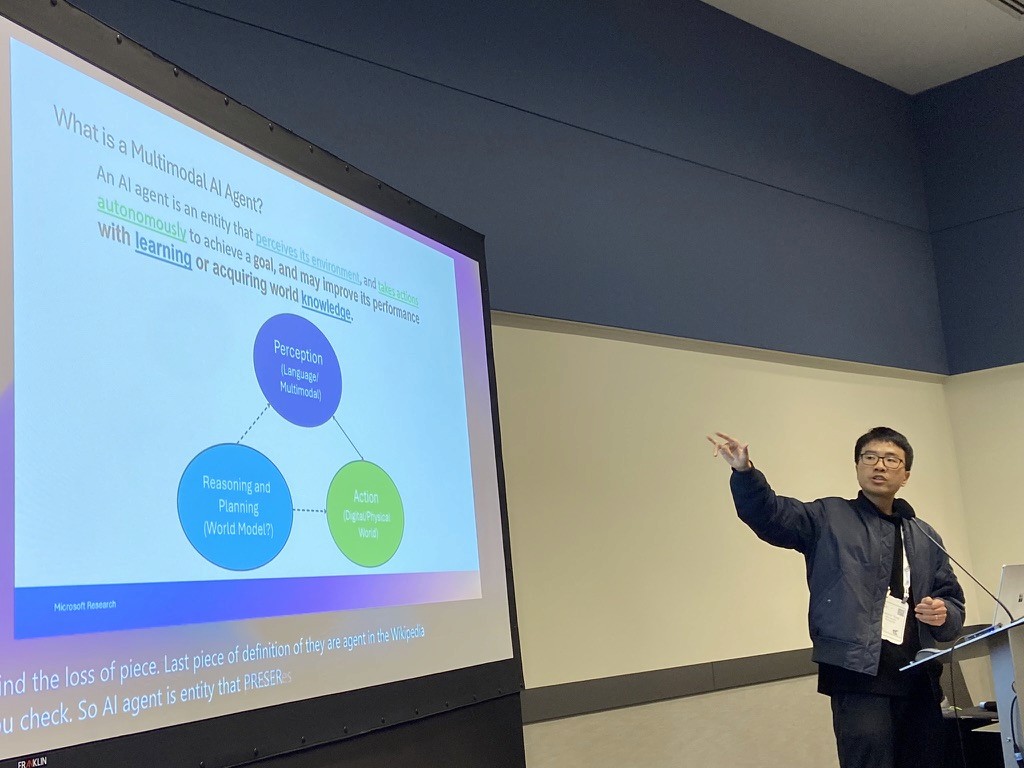
Yang concluded his talk by proposing an expanded definition of multimodal AI agents: "An AI agent is an entity that perceives its environment and takes actions autonomously to achieve a goal, and may improve its performance with learning or acquiring world knowledge." This definition emphasizes three critical aspects:
The transition from 2D to 3D perception for multimodal agents, acknowledging the importance of spatial understanding
The balance between model-free and model-based approaches in multimodal agent development
The crucial role of reasoning and planning in agent behavior
Future Directions and Challenges
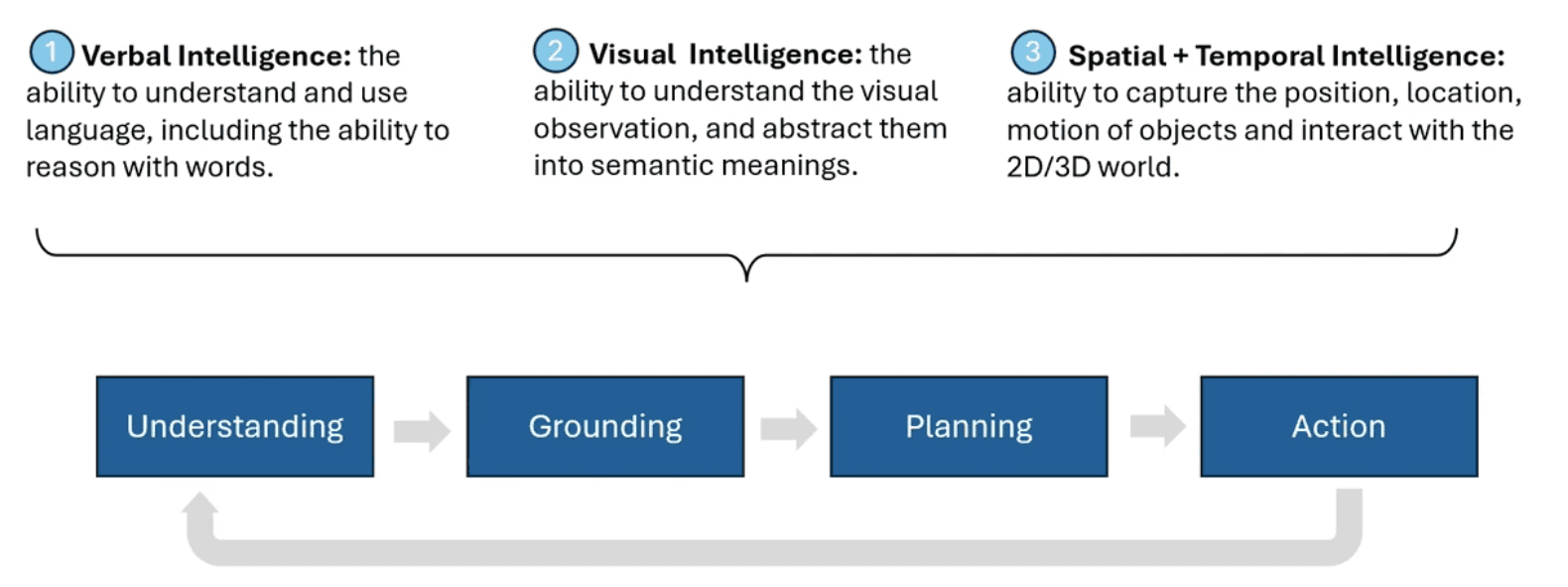
Yang emphasized that scaling remains a vital factor in advancing the field, particularly given the vast potential of video data and real-world interactions. He outlined several key directions for future research:
Multimodal Agentic Capabilities: Developing more sophisticated interaction abilities
Multimodal Reasoning: Enhancing the ability to make logical connections across modalities
Multimodal Understanding: Deepening comprehension of complex multimodal inputs
Multimodal Self-Awareness: Building systems with greater metacognitive capabilities
The presentation concluded with an important observation about current limitations: while visual understanding has improved significantly, it still falls short of human intelligence. Long-context video understanding, detail comprehension, and temporal dynamics remain challenging areas, particularly given the scarcity of high-quality video-text paired data despite the abundance of raw video content.
6 - From Video Generative Models to General World Models - Doyup Lee
Doyup Lee concluded our workshop exploring Runway’s journey from video generative models to general world models, centered around a fundamental question: "How can an intelligent machine understand the visual world?" His presentation delved deep into the nature of visual understanding and the role of imagination in AI systems, drawing from Runway's extensive experience in developing cutting-edge video generation models.
Understanding Visual Intelligence
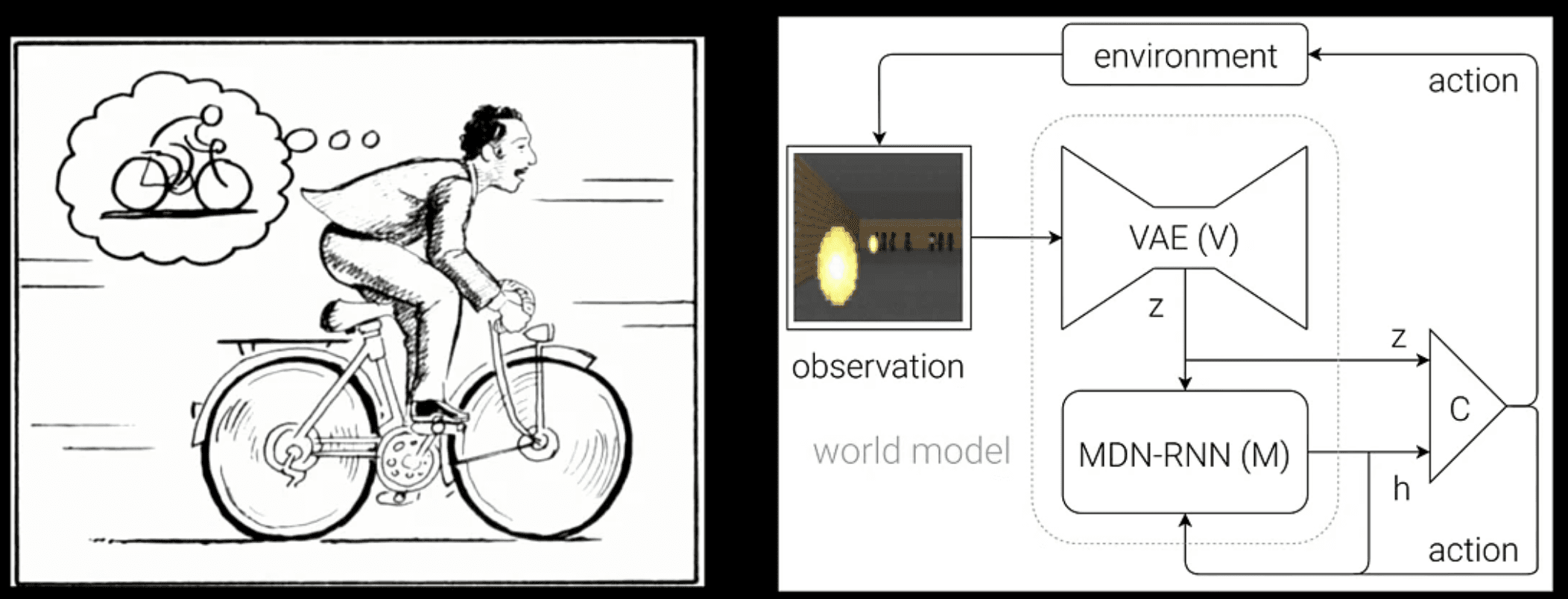
Lee began by examining what constitutes "visual understanding" through the lens of imagination and world modeling. He referenced the seminal work by Ha and Schmidhuber (2018) on World Models, which achieved impressive results in future predictions but remained confined to specific simulators or environments. Traditional visual recognition tasks - including image captioning, visual question answering, and semantic segmentation - have primarily focused on aligning visual information with human knowledge through text, but these approaches are inherently limited by the scope of visual data and world knowledge they can process.
The Platonic Representation Hypothesis
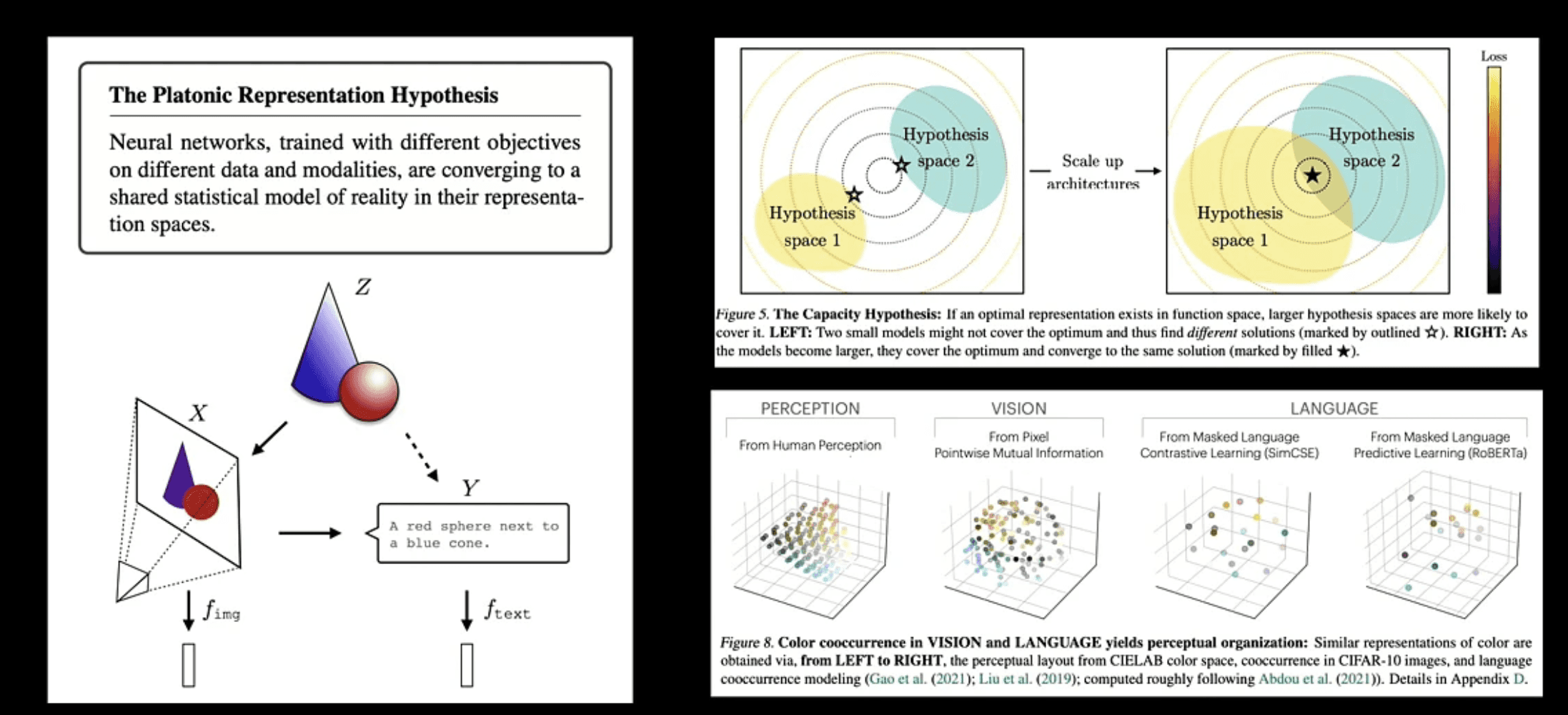
A cornerstone of Lee's theoretical framework is the Platonic Representation Hypothesis (Huh et al., ICML 2024), which identifies three essential components for visual understanding:
The ability to achieve high performance in future prediction tasks, demonstrating true comprehension of visual dynamics
Access to and effective utilization of large and diverse video datasets that capture the complexity of the visual world
Strong alignment with human knowledge, ensuring that the model's understanding corresponds to human concepts and expectations
The Five Essential Recipes for General World Models
1. The Bitter Lesson
Lee emphasized the importance of embracing the "bitter lesson" in AI development: that general methods leveraging computation and learning ultimately triumph over hand-crafted, specialized approaches. This principle guides the development of more flexible and scalable architectures.
2. High-quality and Large-scale Data
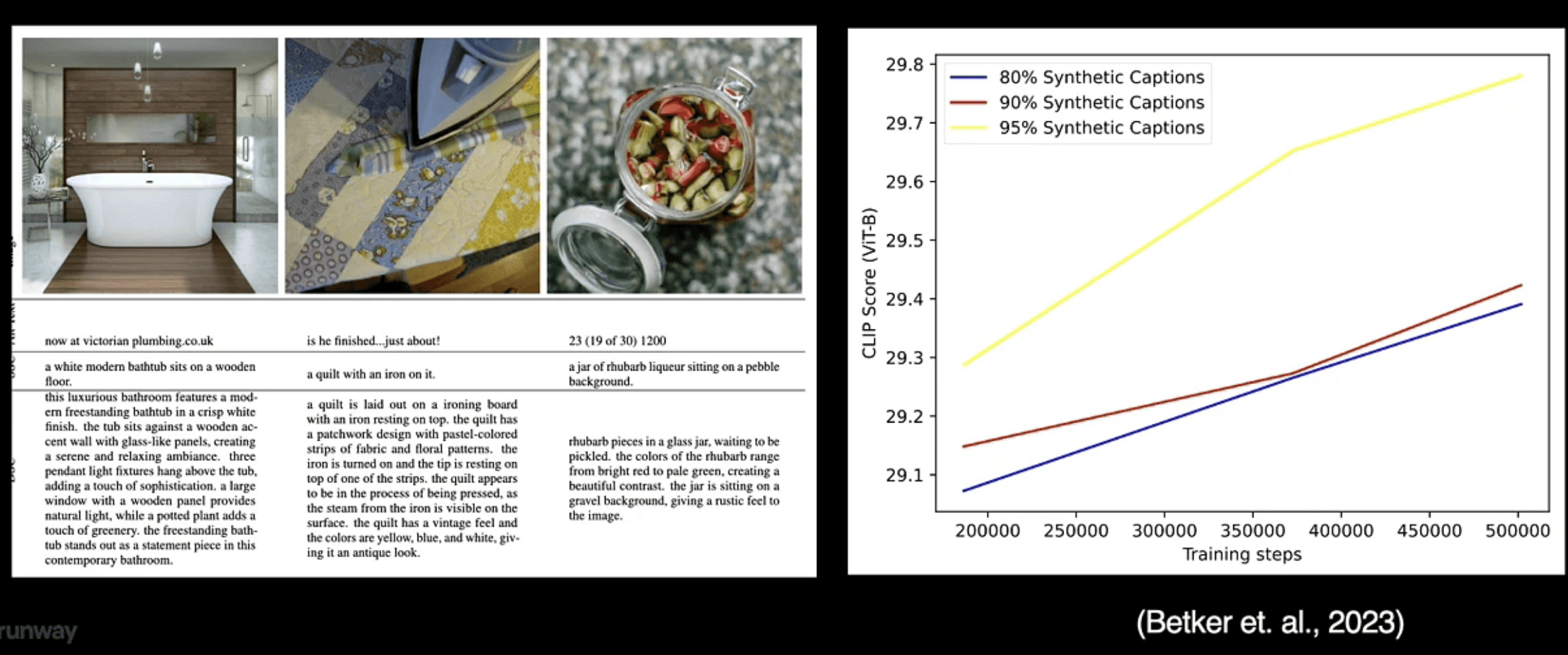
The presentation highlighted the critical role of data quality in model performance. Lee demonstrated how high-quality captions significantly improve both image and video models' capabilities. However, he noted that obtaining high-quality, large-scale video captions remains a significant challenge in the field, requiring innovative approaches to data collection and curation.
3. Scalable Neural Architecture
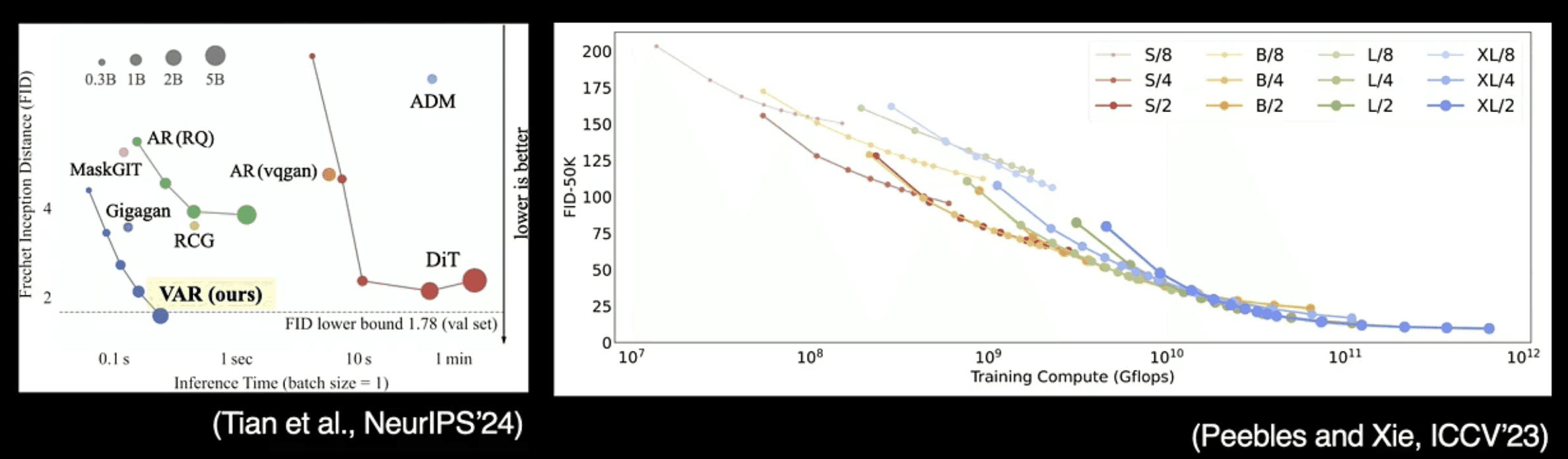
Lee detailed the importance of developing architectures that avoid relational inductive bias between data entities. The presentation included technical discussions of neural scaling laws, which provide an empirical framework for economic training with large compute resources. This approach enables more efficient utilization of computational resources while maintaining model performance.
4. Large-scale Compute Optimization
A critical technical component involves maximizing model FLOPS units (MFUs) during large-scale distributed training. Lee explained that MFU, defined as the ratio of observed throughput to theoretical maximum throughput, serves as a key metric for optimization. The approach encompasses various technical optimizations:
Implementation of low-precision computing techniques
Development of custom CUDA kernels for improved performance
Advanced parallelism strategies
Sophisticated cluster management systems
5. Cross-functional Team Integration
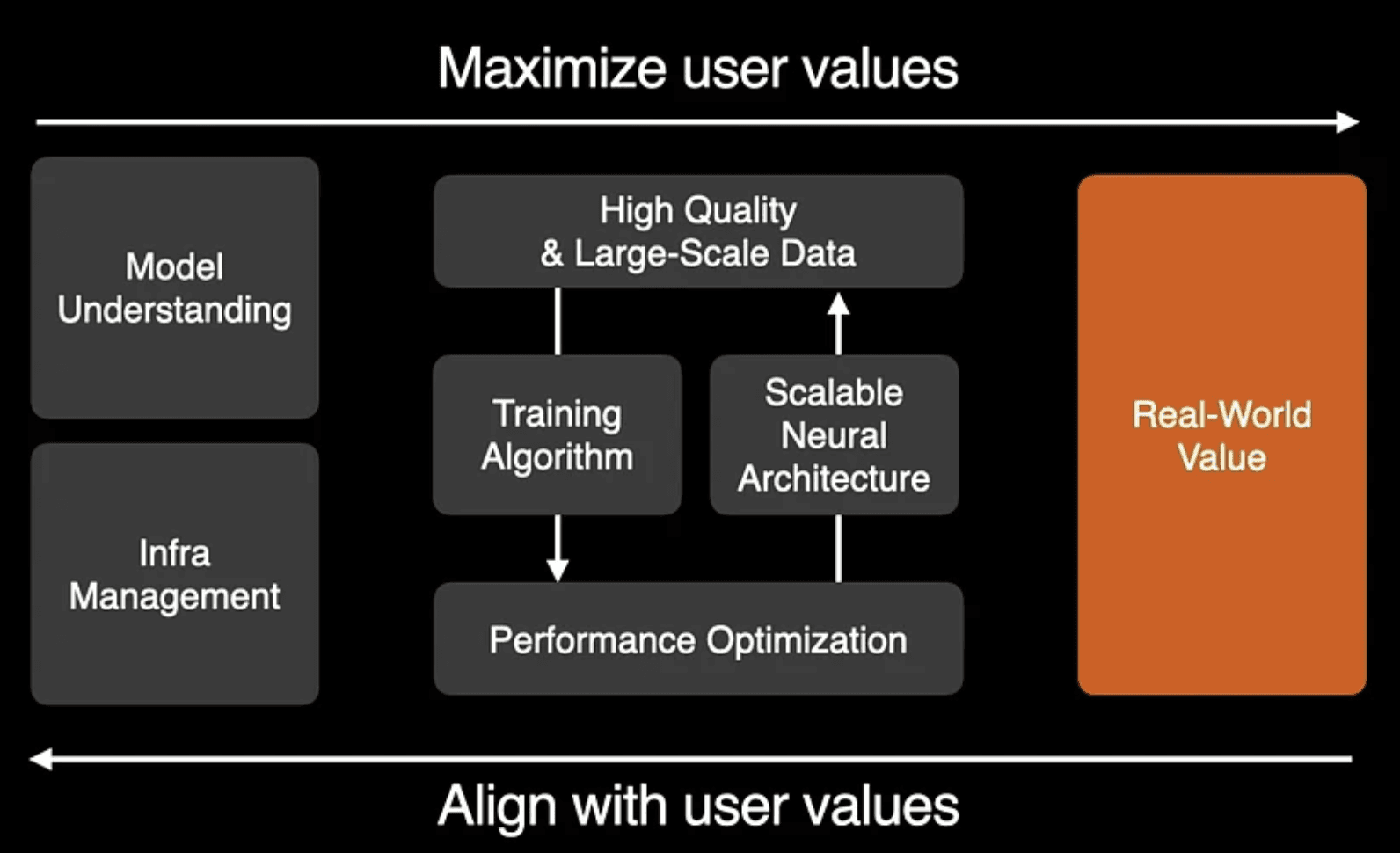
Lee emphasized that training large-scale models requires a systematic approach across functional teams. He presented a comprehensive framework for team collaboration, highlighting the importance of continuous feedback loops and cross-disciplinary expertise.
Runway's Advanced Video Generation Models
Gen-3 Alpha
Lee provided an in-depth look at Runway's Gen-3 Alpha model, which was released in June 2024. The model demonstrates remarkable capabilities in generating high-quality and diverse videos from natural language prompts. However, he acknowledged the ongoing challenge of fully understanding the model's ability to combine and generalize its world knowledge.
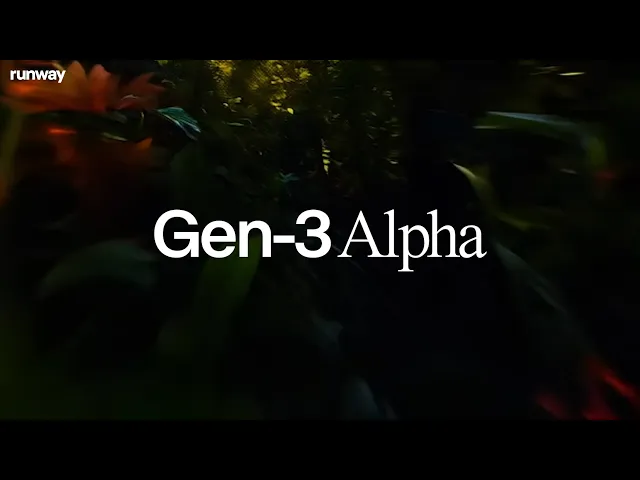
The Runway research team has conducted extensive research to understand the model's capabilities in several key areas:
Understanding and application of physical laws
Text rendering capabilities
Combinatorial generalization across visual concepts
Intuitive Control Mechanisms
Lee introduced several innovative approaches to controlling video generation:
Keyframe conditioning for precise stylistic control
Seamless integration with existing workflows through Generative VFX
Advanced camera control systems with 3D understanding
Fine-grained motion control through specialized brushes
Structural conditioning for video composition
Video out-painting capabilities for expanded creative control
Act-One: The Next Generation
The presentation included a demonstration of Act-One, representing the next evolution in video generation technology. This system builds upon the foundations of Gen-3 Alpha while incorporating more sophisticated control mechanisms and improved understanding of temporal dynamics.
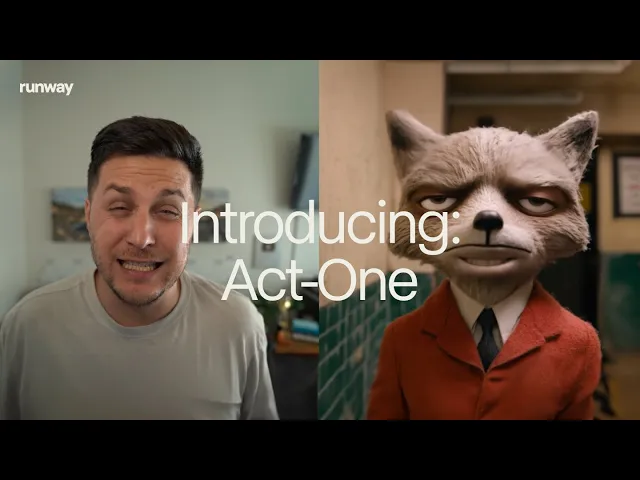
Future Directions and Challenges
Lee concluded by emphasizing that language and video serve as fundamental world descriptors: language providing the most abstract knowledge representation, and video offering the most scalable source of visual world representation. He stressed that scaling remains a crucial factor, with access to gigantic video datasets and the entire visual world for interaction.
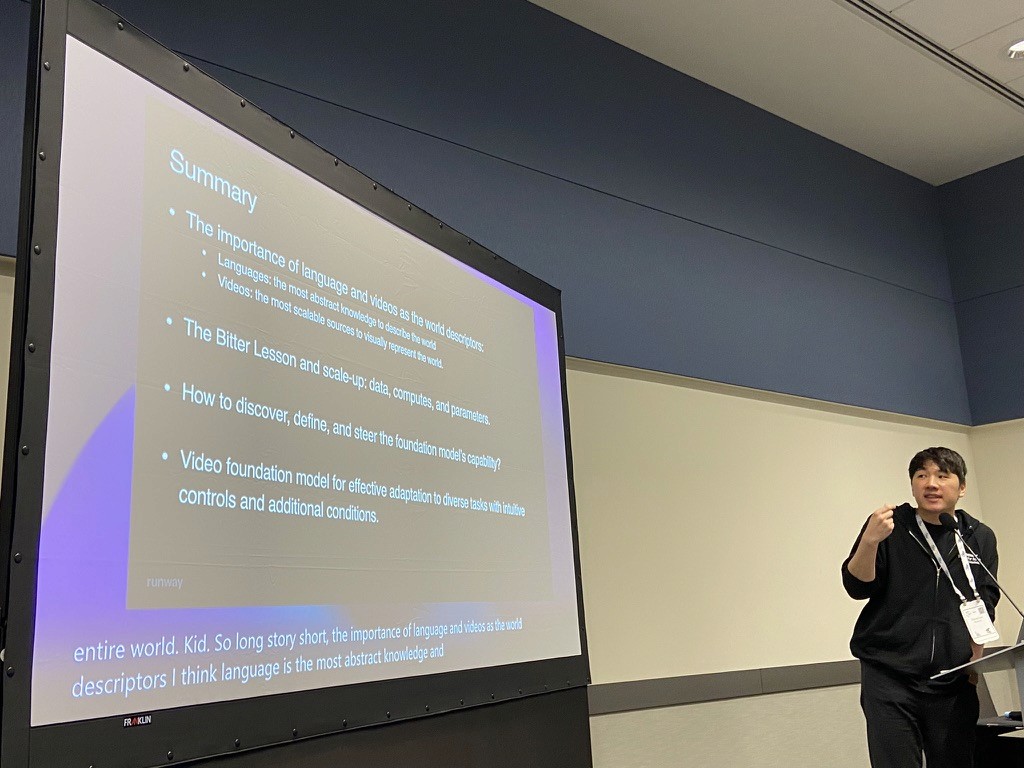
The presentation ended with a clear vision: the development of General World Models that understand the world at an unprecedented level. This goal requires continued innovation in:
Model architecture and scaling
Data collection and curation
Computational efficiency
Intuitive control mechanisms
Integration with existing creative workflows
The Future of Video-Language Models: A Vision Forward
The inaugural NeurIPS 2024 Video-Language Model Workshop has illuminated both the remarkable progress and compelling challenges in the field of video understanding. As an industry practitioner and organizer of this workshop, Twelve Labs recognizes the critical themes that emerged from our distinguished speakers' presentations.
Key Insights and Emerging Themes
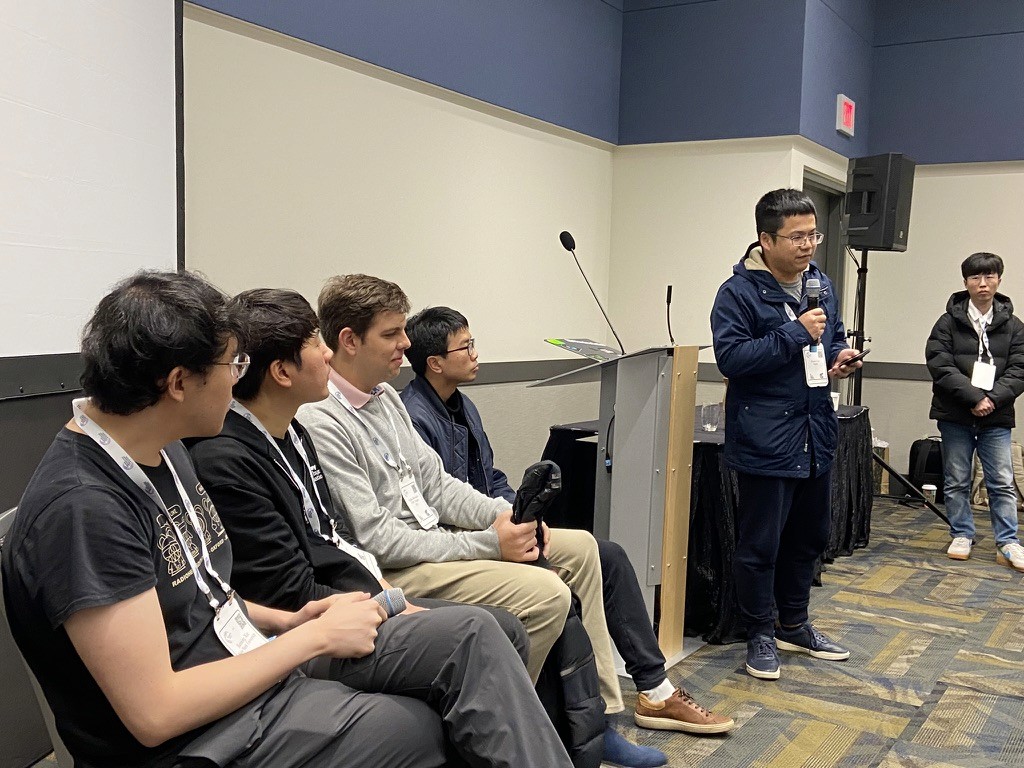
Several crucial themes resonated throughout the workshop. Professor Dima Damen highlighted the importance of understanding fine-grained temporal dynamics in egocentric videos, while Professor Gedas Bertasius emphasized the need to move beyond pure language-centric approaches in video understanding. Professor Yong Jae Lee's presentation revealed that even short-form video understanding remains challenging, particularly when dealing with counterfactual temporal information.
Jianwei Yang's vision of multimodal agentic models and Ishan Misra's insights into video generation demonstrated how the field is evolving beyond simple understanding tasks toward more sophisticated applications. Doyup Lee's exploration of general world models highlighted the potential for video-language models to develop comprehensive world understanding.
Industry Perspective and Path Forward
At Twelve Labs, these challenges resonate deeply with our experience developing video understanding technologies. Our journey with Marengo, our video embedding model, and Pegasus, our video-to-text model, has taught us firsthand about the complexities of multimodal integration, temporal understanding, and the critical importance of high-quality training data.
The workshop discussions validate our approach to tackling these challenges through:
Multi-vector embeddings that capture the multifaceted nature of video content
Sophisticated temporal modeling that addresses both short-term and long-term dependencies
Integration of multiple modalities including visual, audio, and textual information
Development of robust evaluation frameworks that go beyond traditional metrics
Vision for the Future
The future of video-language models is incredibly promising. As we move forward, we envision:
More sophisticated temporal understanding capabilities that can handle complex narratives and counterfactual reasoning
Better integration of world knowledge with visual understanding
More efficient architectures that can process longer videos while maintaining temporal coherence
Stronger bridges between academic research and industrial applications
This workshop marks an important milestone in bringing together researchers and practitioners to address these challenges collectively. As we continue to push the boundaries of what's possible in video understanding, we remain committed to fostering open collaboration and responsible development in this rapidly evolving field.
The path ahead is both challenging and exciting. By continuing to build on the insights shared at this workshop and maintaining strong collaboration between academia and industry, we can accelerate the development of more capable, efficient, and reliable video-language models that will transform how we perceive and interact with the world.
The inaugural NeurIPS 2024 Video-Language Model Workshop marked a pivotal moment in the rapidly evolving field of video understanding and generation. In his opening remarks, our CTO Aiden Lee highlighted the growing interest in video-language models across two key areas: video understanding (video-to-text) and video generation (text-to-video).
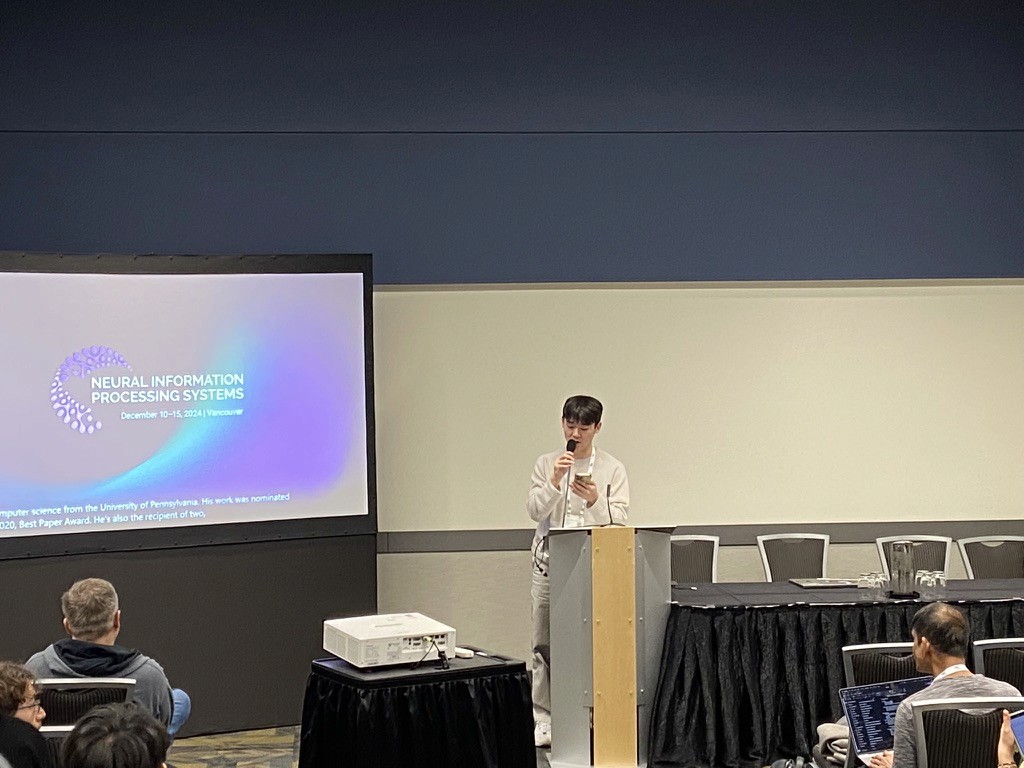
The applications of these technologies span diverse sectors, including content creation, advertising, healthcare, and robotics. Despite the exponential growth in vision-language model research, the field faces several critical challenges:
Data scarcity, particularly in high-quality video and text pairs
Scale and complexity issues in video storage and processing
Challenges in multimodal integration across visual, audio, and text components
Difficulties in benchmarking and evaluating video-language alignment
The workshop aimed to address these challenges by bringing together researchers to discuss current obstacles, foster open collaboration, and accelerate the development of video foundation models for real-world applications.
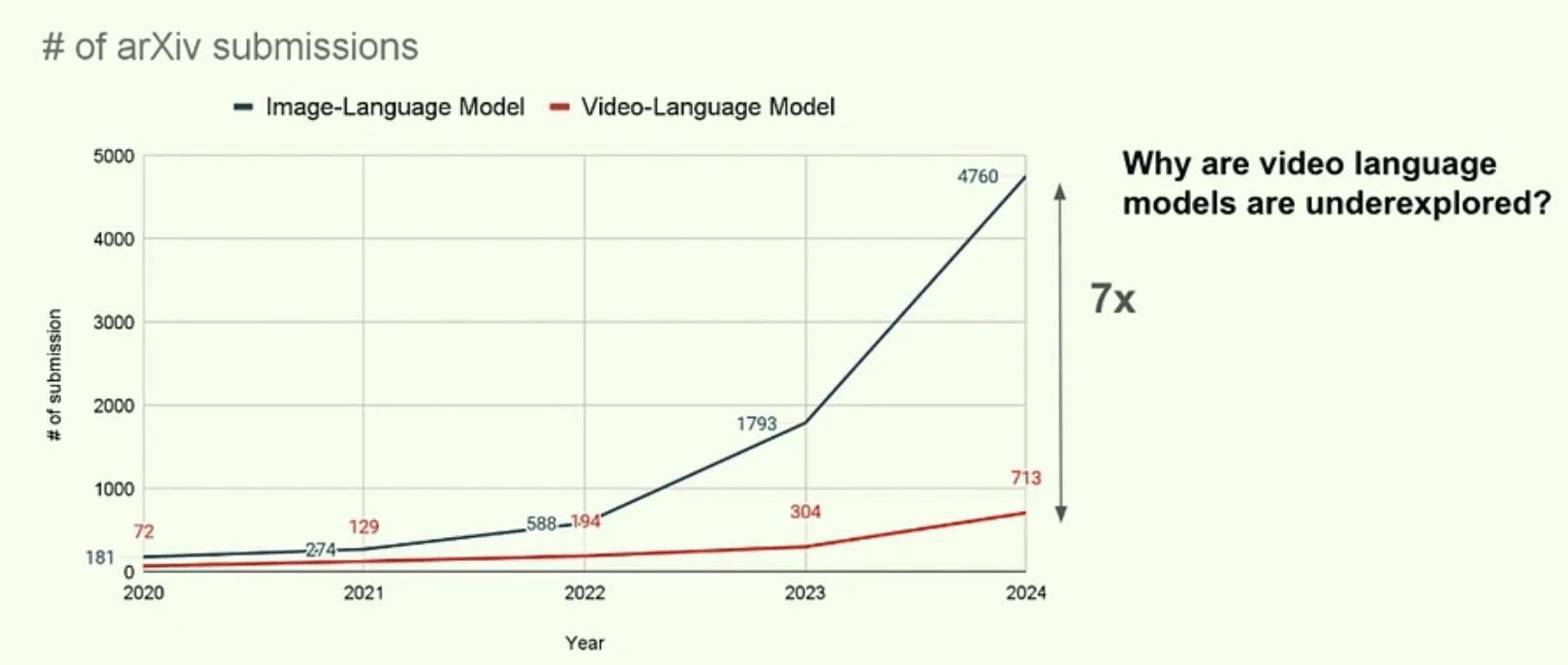
1 - Egocentric Video and Language Understanding - Dima Damen
Professor Dima Damen's comprehensive talk explored four cutting-edge aspects of egocentric video and language understanding. Her research draws from the EPIC-KITCHENS dataset and introduces several groundbreaking approaches.
Unique Captioning for Similar Actions
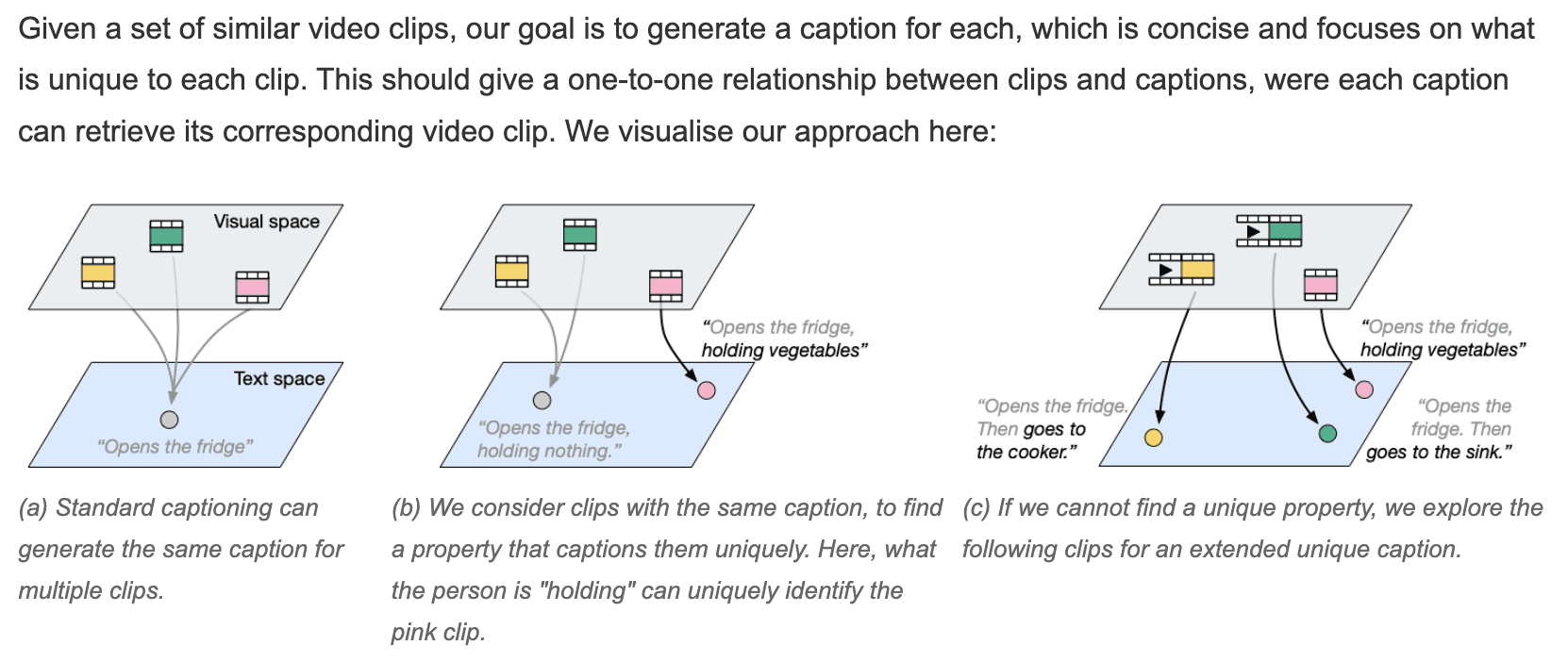
The paper "It's Just Another Day," which won the Best Paper Award at ACCV 2024, addresses a fundamental challenge in video captioning: the tendency of current methods to generate identical captions for similar clips when processing them independently.
The key insight behind this work is that while life is repetitive, we need distinct descriptions for similar actions. The researchers propose a novel approach called Captioning by Discriminative Prompting (CDP) that:
Considers clips jointly rather than in isolation
Utilizes a bank of discriminative prompts to generate unique captions
Evaluates through both video-to-text and text-to-video retrieval metrics
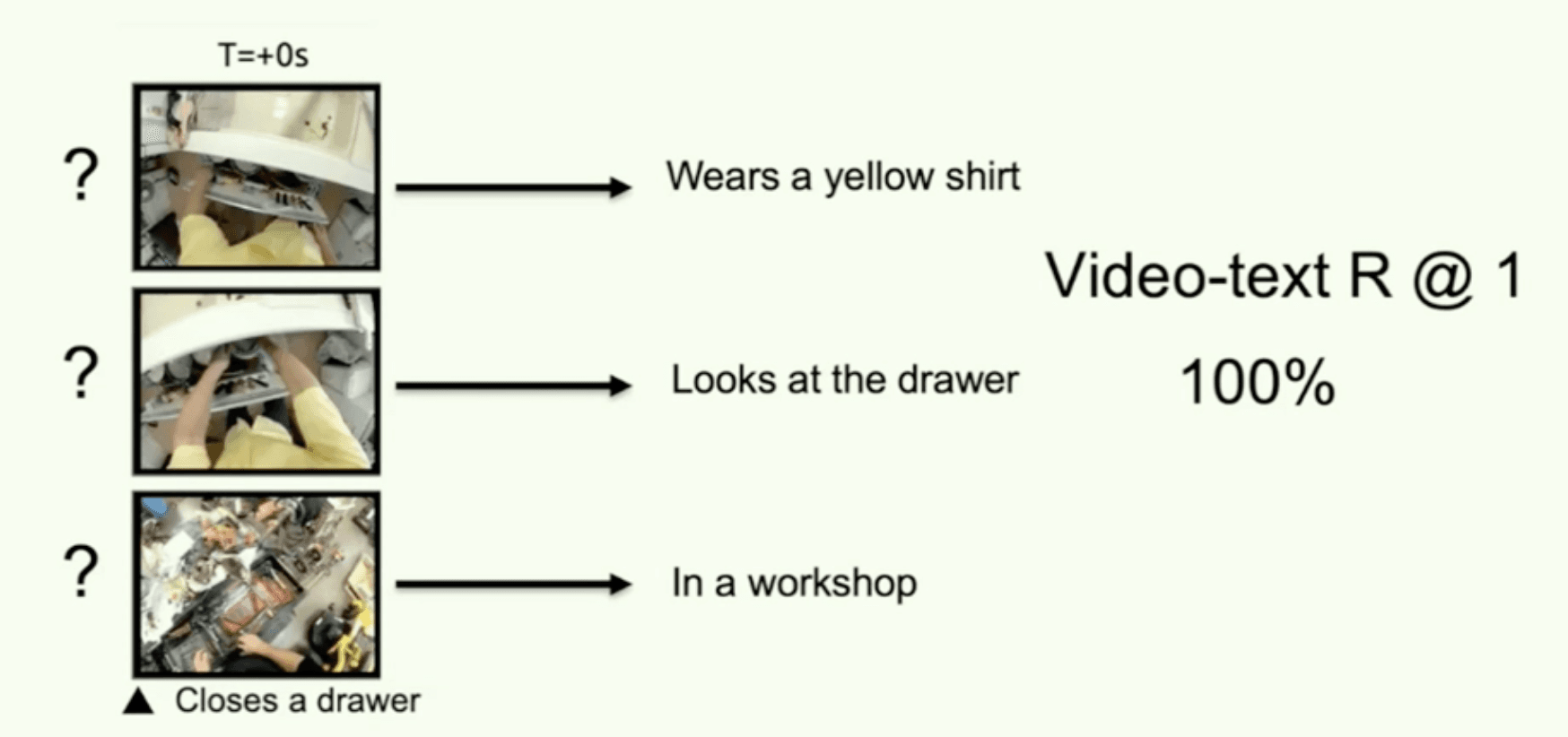
The method was evaluated on two domains: egocentric videos from Ego4D and timeloop movies. When applied to the LaViLa VCLM captioner, CDP showed significant improvements in caption uniqueness while maintaining semantic accuracy.
Domain Generalization Using Language
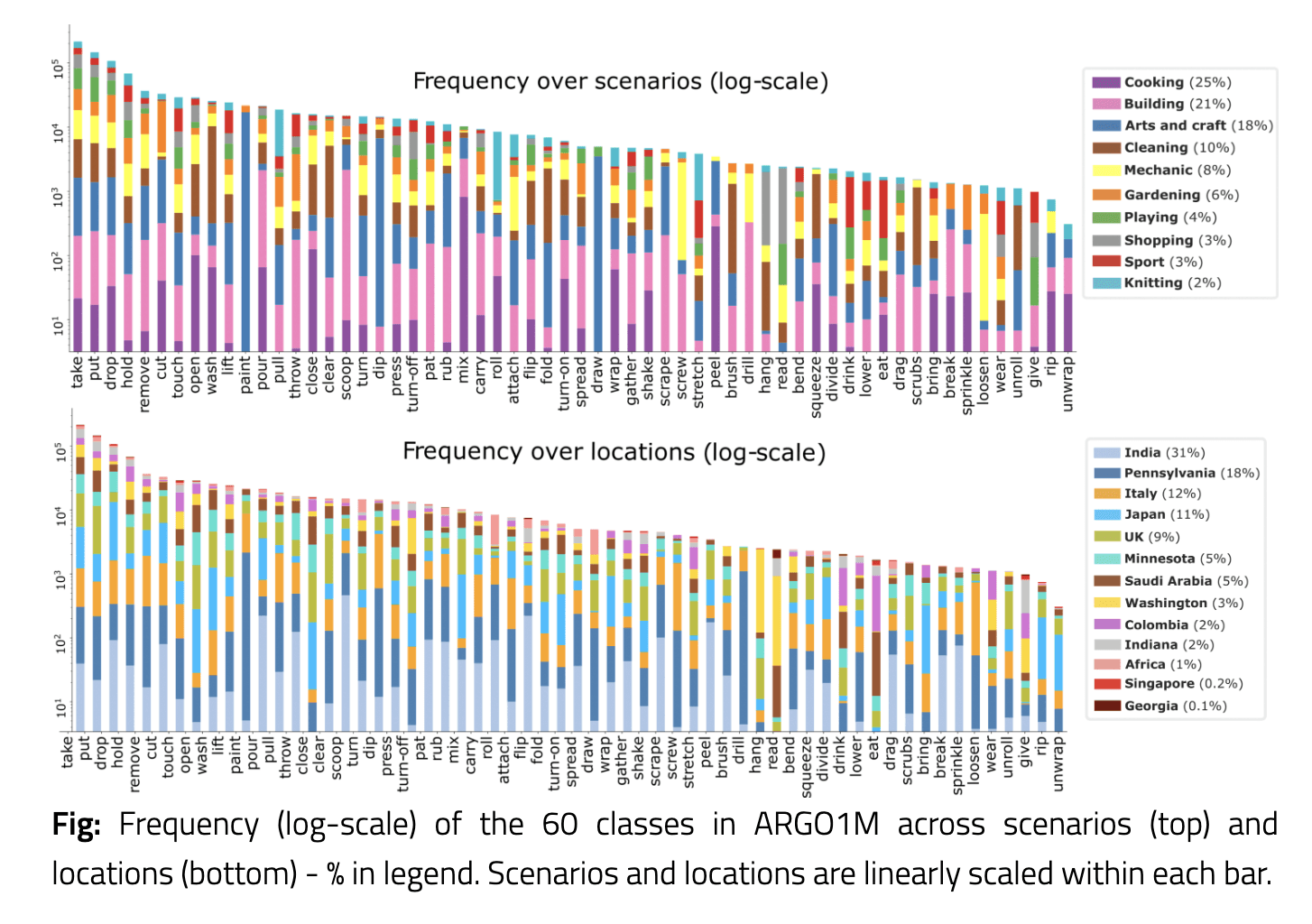
A major contribution is the ARGO1M dataset, the first of its kind designed specifically for Action Recognition Generalization across scenarios and locations. The dataset features:
1.05M action clips spanning 60 action classes
Recordings from 13 different locations
10-second clip duration standard
The accompanying paper "What can a cook in Italy teach a mechanic in India?" introduces Cross-Instance Reconstruction (CIR), which employs two key reconstructions:
Video-text association reconstruction using text narrations
Classification reconstruction trained for action class recognition
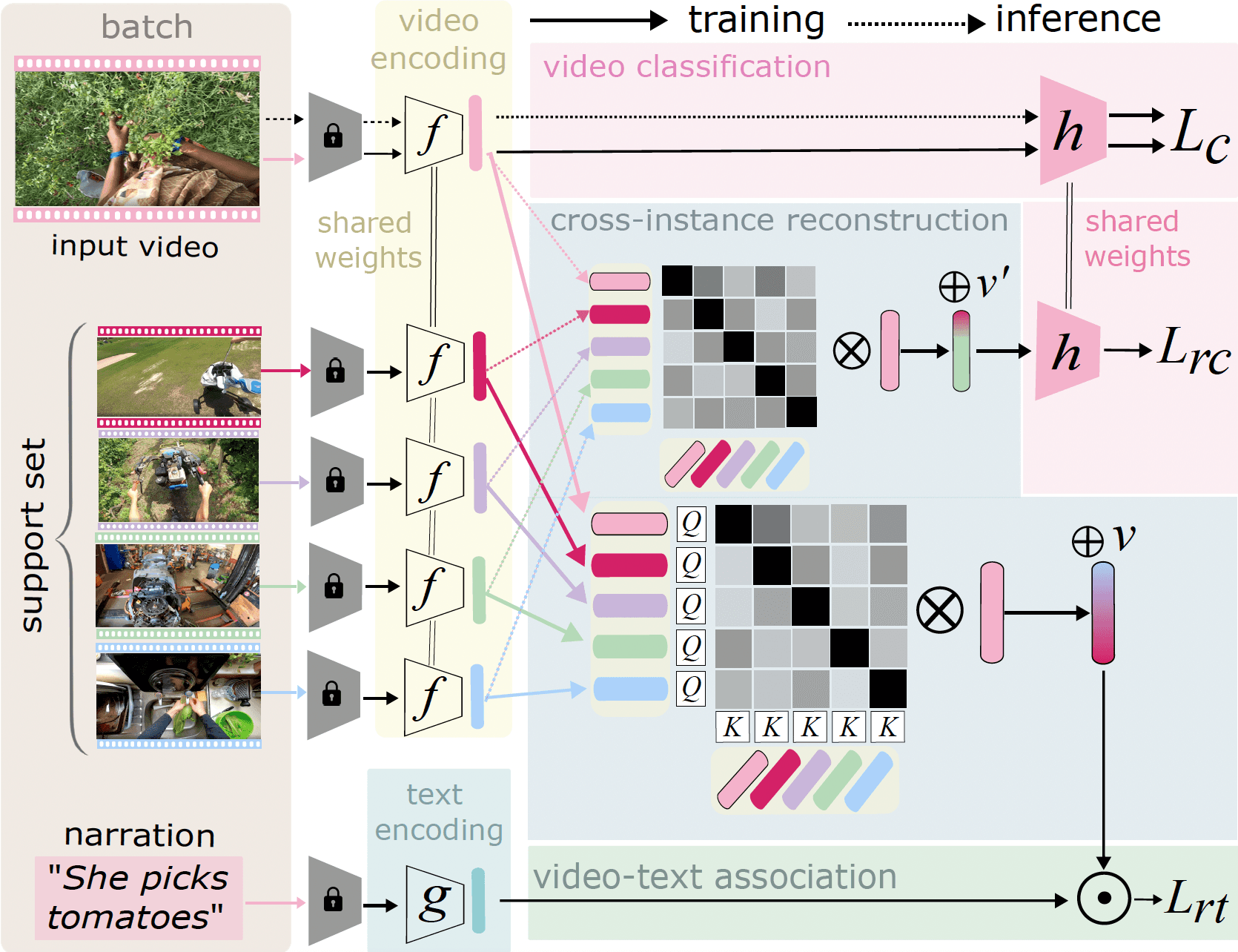
CIR represents actions as weighted combinations of actions from different scenarios and locations, enabling robust cross-domain generalization.
Hand-Object Interaction Referral
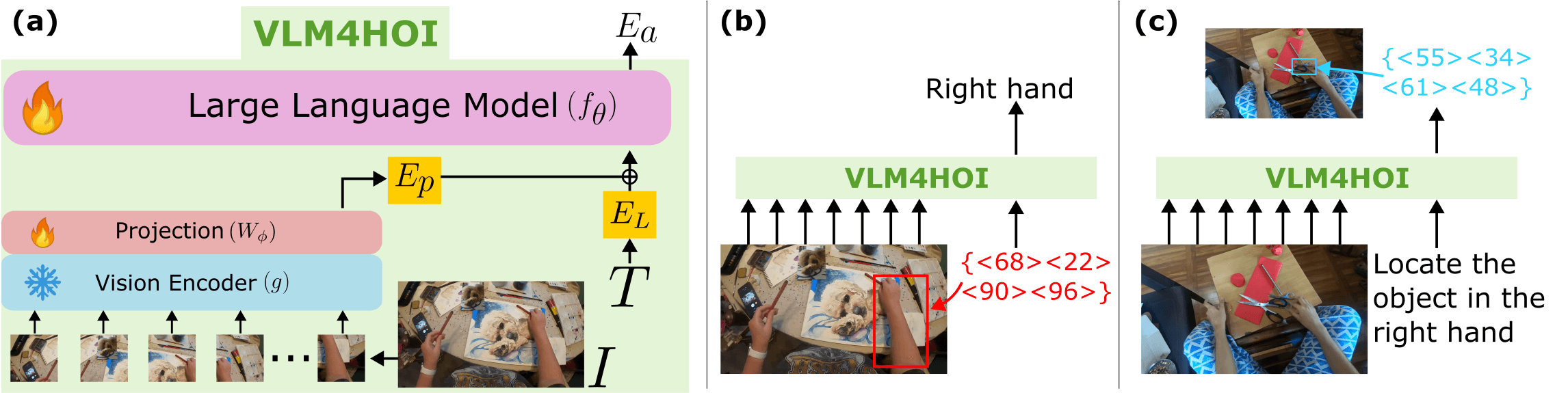
The HOI-Ref project introduces VLM4HOI, a sophisticated model for hand-object interaction referral in egocentric images. The model architecture includes:
A vision encoder (g) and projection layer (Wφ) for image processing
Language model embedding space integration
Capability to identify both hands and interacted objects
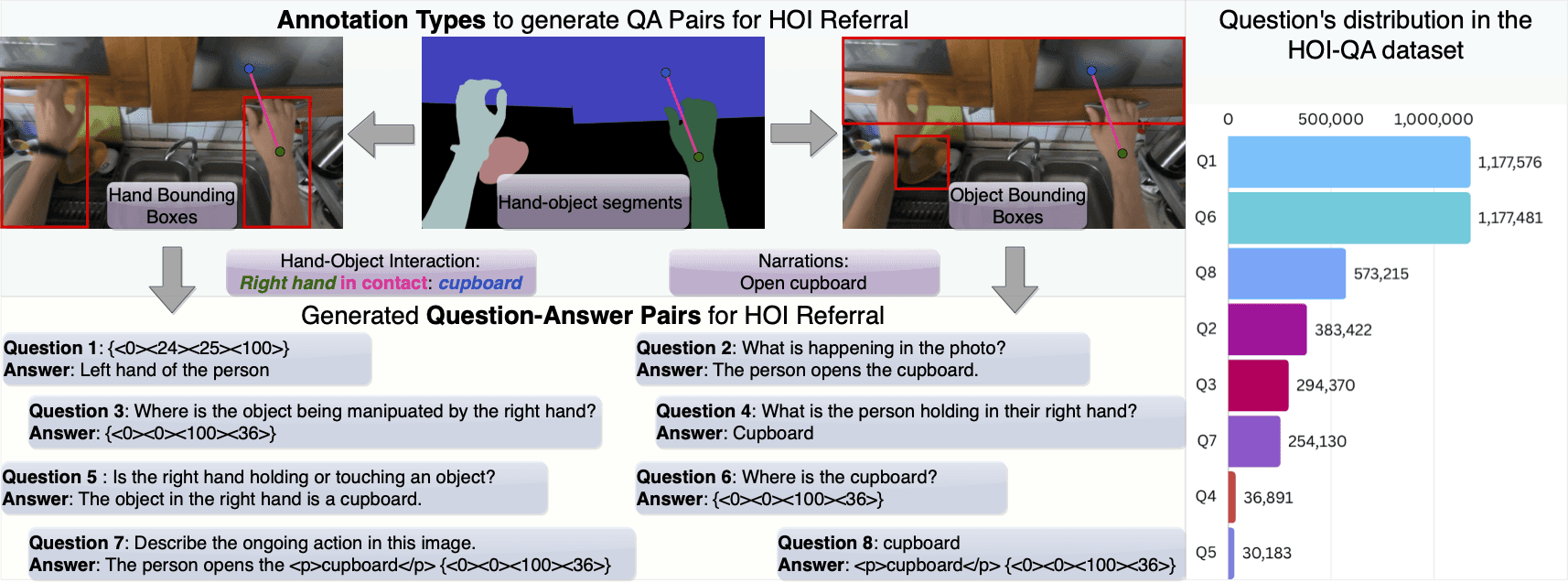
The researchers developed HOI-QA, a comprehensive dataset containing 3.9M question-answer pairs, focusing on:
Spatial referral and recognition of hands and objects
Understanding of hand-object interactions
Direct referral versus interaction referral capabilities
Audio-Visual Semantic Integration
This section presented two significant contributions to the field:
EPIC-SOUNDS Dataset
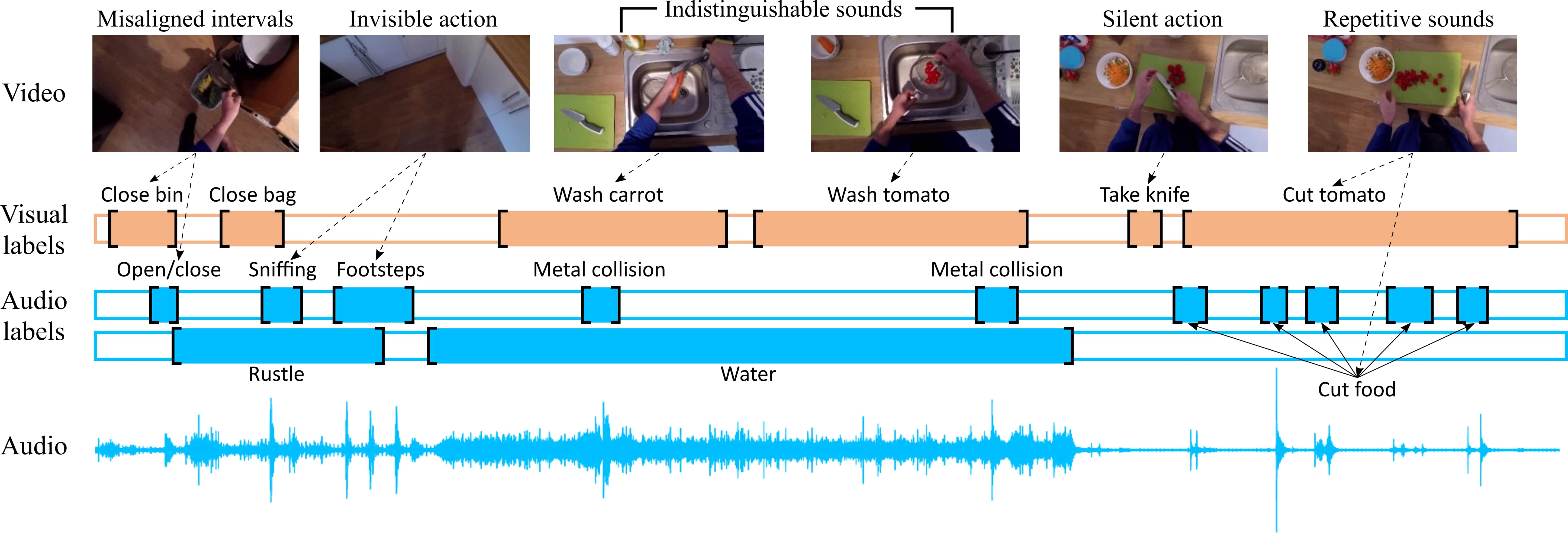
This large-scale dataset captures audio annotations within egocentric videos from EPIC-KITCHENS-100, featuring:
75.9k segments of audible events and actions
44 distinct action classes
Detailed material annotations for object collision sounds
Temporal labeling of distinguishable audio segments
Time Interval Machine (TIM)
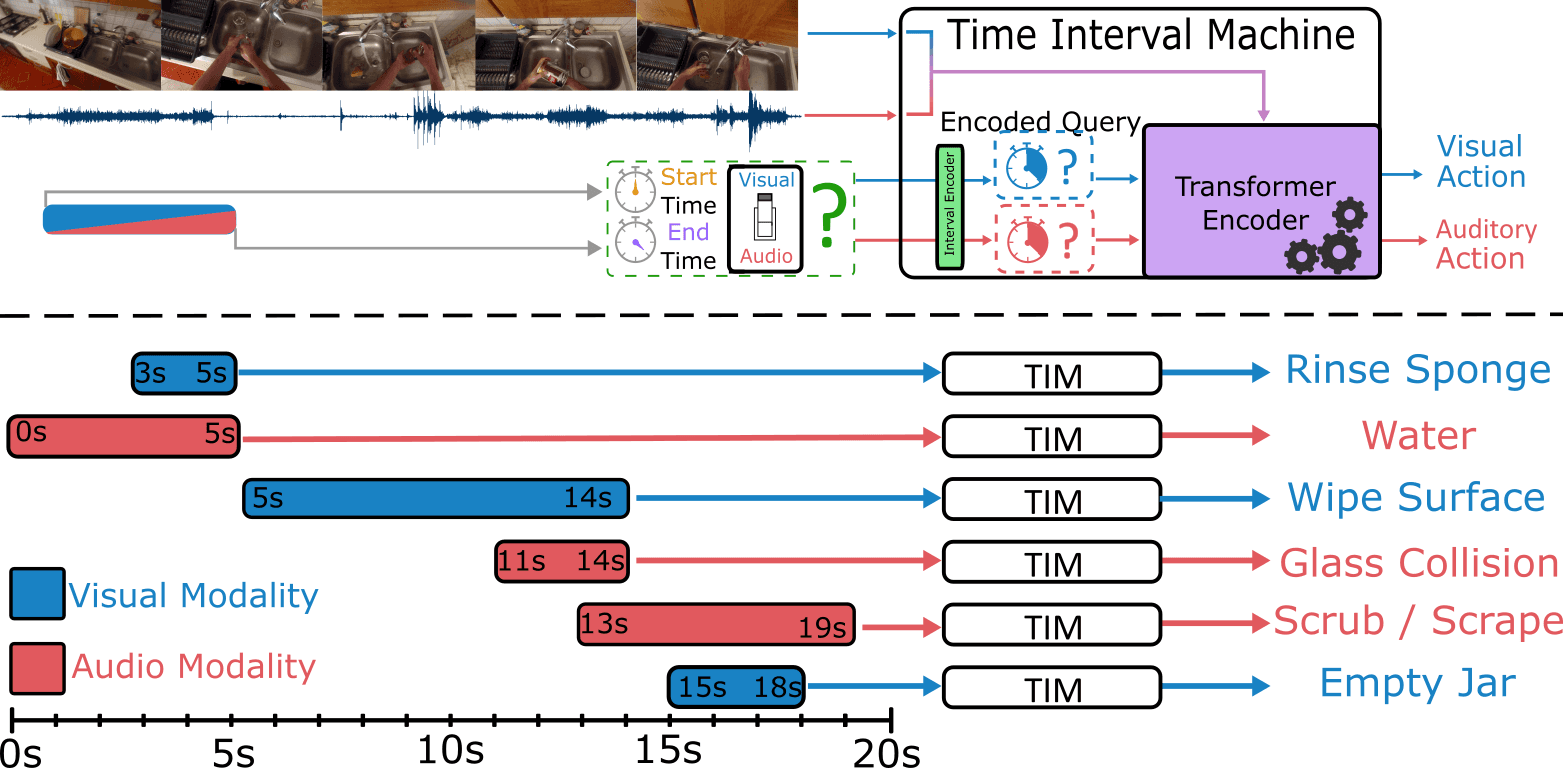
TIM represents a breakthrough in handling the different temporal extents of audio and visual events in long videos. Key features include:
A transformer encoder that processes modality-specific time intervals
Attention mechanisms for both interval-specific and surrounding context
State-of-the-art performance on multiple benchmarks
The model achieved remarkable results:
2.9% improvement in top-1 action recognition accuracy on EPIC-KITCHENS
Superior performance on Perception Test and AVE datasets
Successful adaptation for action detection using dense multi-scale interval queries
These advances collectively demonstrate significant progress in understanding and processing egocentric video content, particularly in handling the complex interplay between visual, textual, and audio modalities. The research from Dima’s group presents a comprehensive framework for addressing the challenges of multimodal integration in egocentric video understanding.
2 - Complex Video Understanding using Language and Video - Gedas Bertasius
Professor Gedas Bertasius's talk highlighted both the remarkable progress and current limitations of video-language models (VLMs) in complex video understanding tasks. While acknowledging the significant advances in video understanding due to LLMs and VLMs, he emphasized that modern VLMs still face fundamental challenges with complex video understanding tasks.
Addressing Long-Range Video Understanding
A key technical challenge identified is the struggle of existing models to localize relevant content in long video sequences. Bertasius introduced Structured State-Space Models (S4) as an efficient approach to model long-range dependencies in video (as seen in this work and this work). However, he noted that S4 models struggle with tasks requiring selective attention to specific parts of the input sequence, as their parameters remain constant for every input token.
BIMBA: Selective-Scan Compression
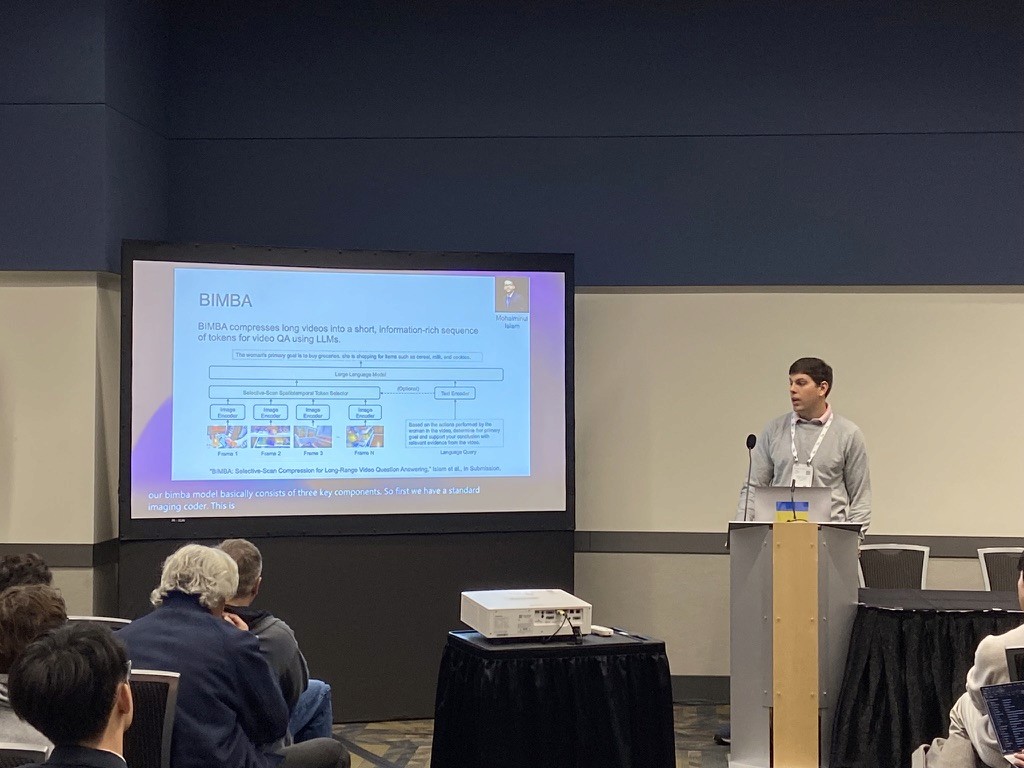
BIMBA addresses these limitations by introducing the selective scan algorithm (also known as Mamba) to choose which parts of the input should be stored in the hidden state in a data-dependent manner. The model features:
A spatiotemporal token selector using bidirectional selective-scan mechanism
Interleaved visual queries for precise content selection
Input-dependent parameters for adaptive processing
Efficient compression of long videos into information-rich token sequences
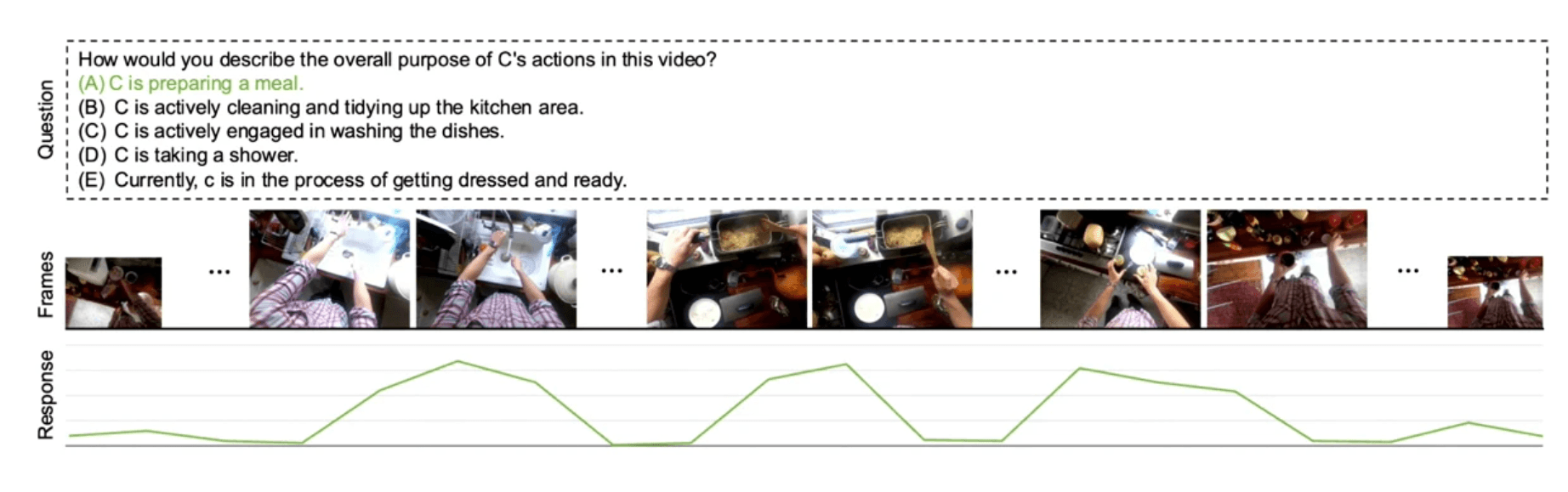
In evaluation on the EgoSchema benchmark, BIMBA demonstrated superior performance in identifying the most relevant parts of videos for question answering tasks, with qualitative results showing precise selection of video segments relevant to given queries.
LLoVi: LLM Framework for Long VideoQA
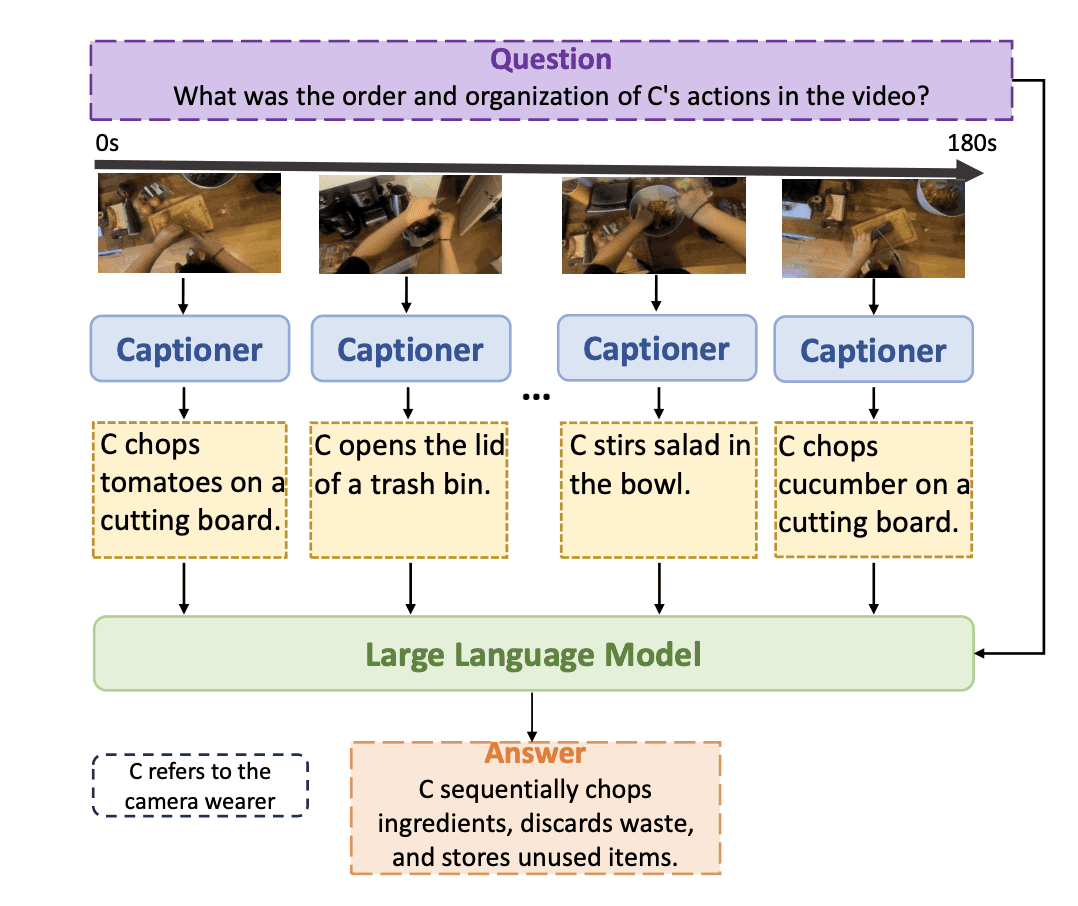
LLoVi introduces a more straightforward but highly effective approach to long-range video question answering through a two-stage process:
Stage 1: Video Processing
Samples short clips from long videos
Extracts captions for each clip
Maintains temporal ordering of information
Stage 2: Question Answering
Feeds temporally ordered captions into an LLM
Employs a novel two-round summarization prompt
Enhances complex question-answering capabilities
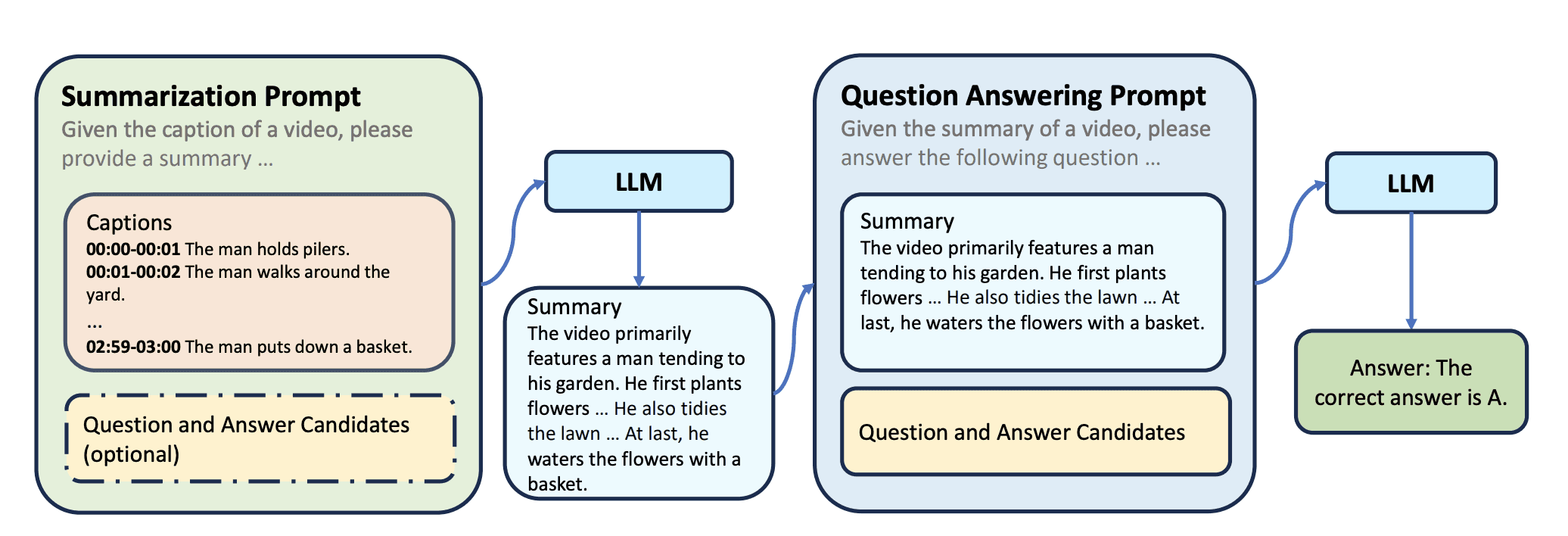
The evaluation results on EgoSchema demonstrated LLoVi's effectiveness in handling long-form video content, though Bertasius noted that the model uses 1750 times more parameters to model language than video, raising questions about efficiency and information preservation.
Beyond Language-Centric Approaches
Bertasius emphasized the limitations of representing videos purely through language, noting that captions and summaries dramatically reduce the rich information present in original video content.
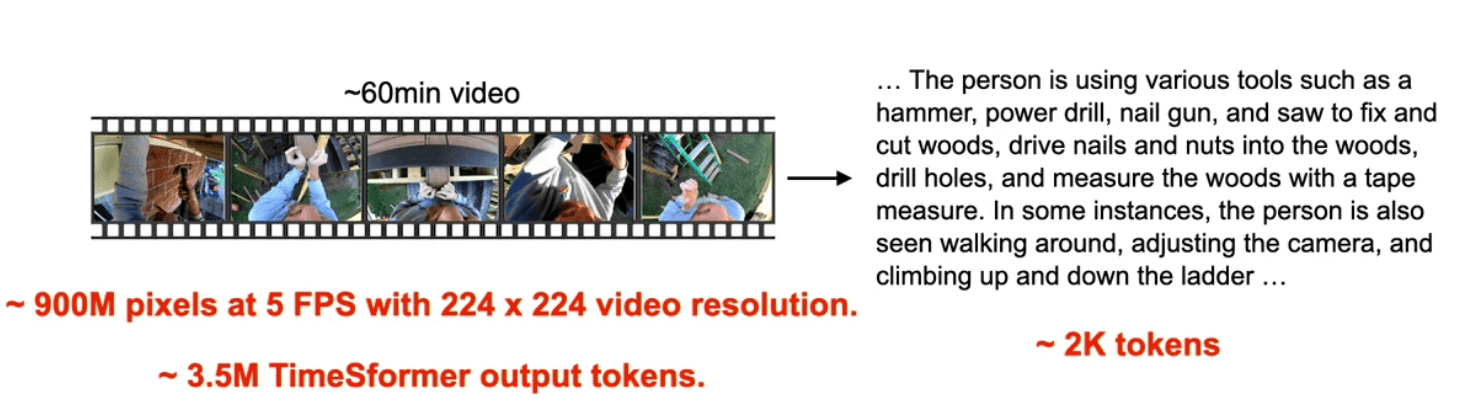
BASKET: Fine-Grained Skill Estimation Dataset
To address these limitations, the talk introduced BASKET, a comprehensive dataset for fine-grained skill estimation that focuses on visual understanding rather than language description. The dataset includes:
4,400+ hours of video content
32,232 players from 30+ countries across 4 continents
20 fine-grained basketball skills
8-10 minute highlight videos per player
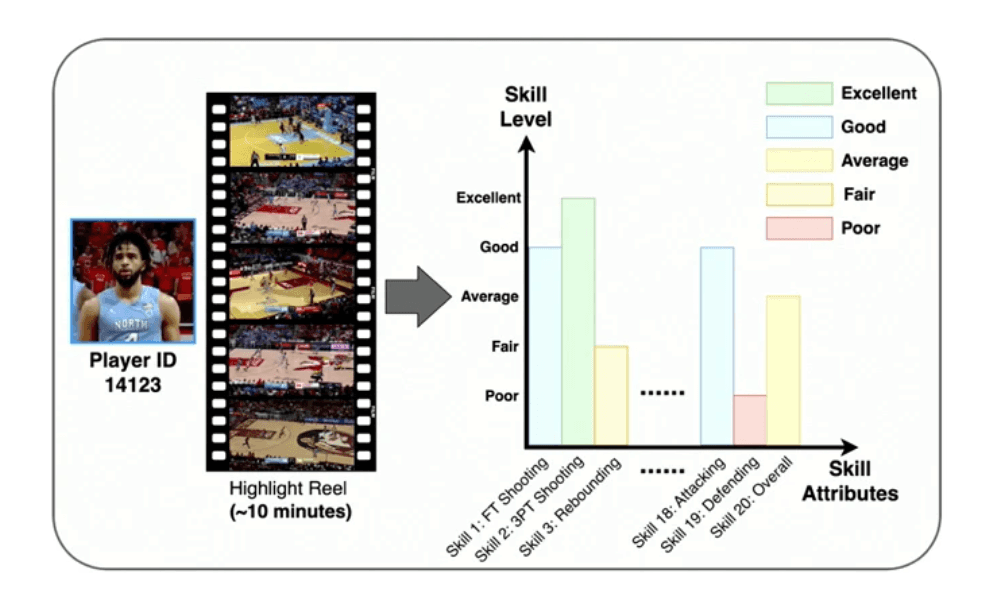
Basketball was selected as the focus sport due to several key advantages:
Global reach with 400M+ fans
High participant diversity
Abundant video footage availability
Complex fine-grained skills requiring temporal modeling
Similar player appearances necessitating fine-grained visual recognition
Performance Results:
Current state-of-the-art models struggle with fine-grained skill estimation
No model achieved more than 30% accuracy on the benchmark
Results highlight the significant gap between human and AI performance in complex video understanding tasks
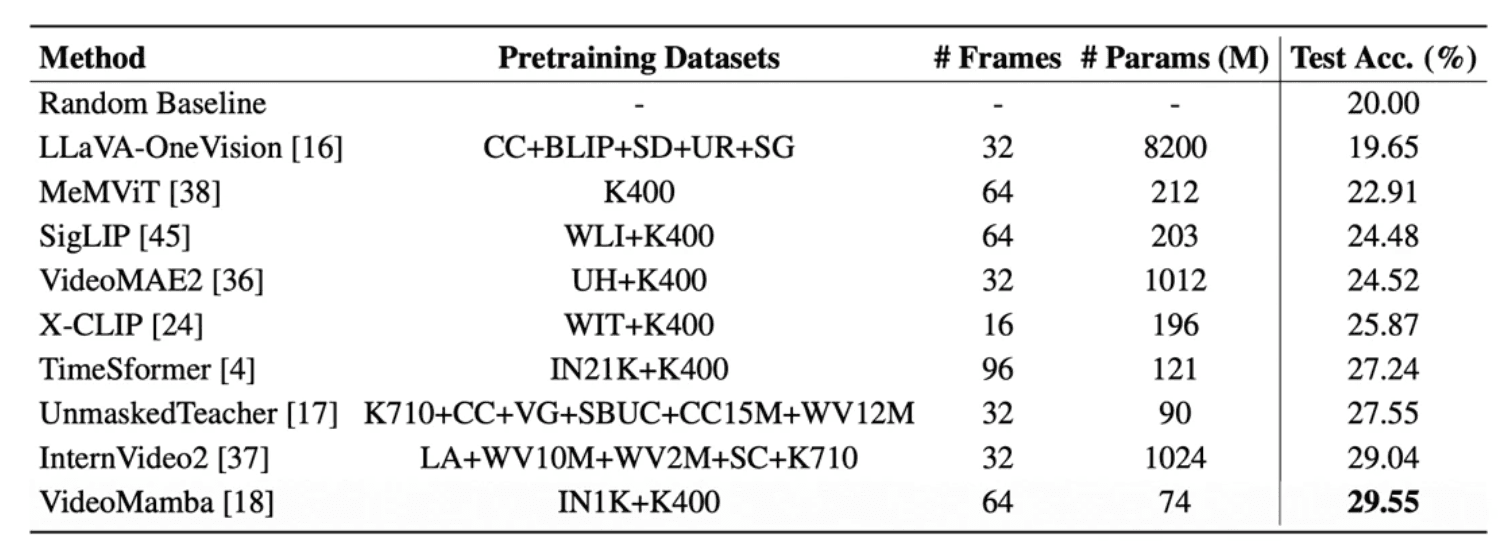
Key Takeaways and Future Directions
While LLMs have enabled remarkable progress in video understanding tasks, problems that are difficult to formulate using language don't benefit as much from language models or large-scale web video-text pre-training
The strong performance of LLMs and flaws in existing video-language benchmarks may mask underlying issues with video modeling
Current evaluation metrics may not fully capture the complexity of video understanding tasks
The field needs to develop more sophisticated approaches that can handle both language-based and purely visual understanding tasks
3 - How Intelligent Are Current Multimodal Video Models? - Yong Jae Lee
Professor Yong Jae Lee's talk challenged the conventional wisdom about the capabilities of current multimodal video models. While much of the research community has been focusing on understanding long-form videos, Lee emphasized a crucial point: understanding even short videos (less than 10 seconds) with counterfactual temporal information remains a significant challenge. This observation led to the development of two major contributions: Vinoground and Matryoshka Multimodal Models.
Vinoground: A New Benchmark for Temporal Reasoning
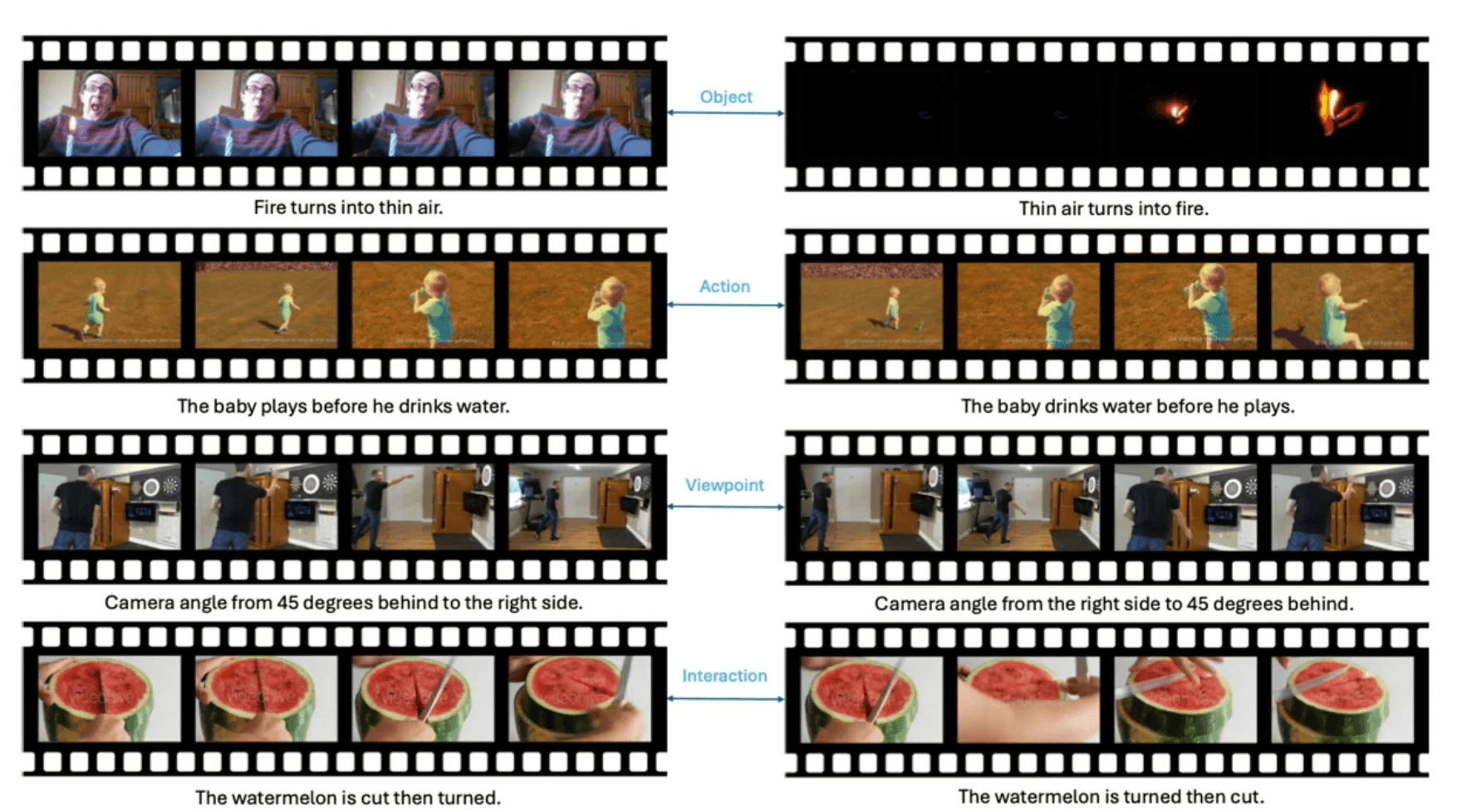
Inspired by Winoground, a challenging counterfactual benchmark for visio-linguistic compositional reasoning in images, Vinoground extends this concept to the video domain. The benchmark's name cleverly changes the 'W' to a 'V' for "video" while maintaining the focus on temporal counterfactuals as a unique element in video data.
The benchmark was designed with several crucial requirements to ensure robust evaluation of video understanding capabilities:
Videos must not contain single frame bias that could allow models to "cheat" by looking at individual frames
Tasks cannot be solved through textual cues alone, requiring true visual understanding
All videos must be natural, avoiding synthetic or artificially constructed scenarios
Complete video comprehension is necessary, with particular emphasis on event ordering
To make the benchmark especially challenging, each video pair comes with corresponding captions that use identical words but in different orders. For example, "a man waves at a woman before he talks to her" versus "a man talks to a woman before he waves at her." This design ensures that models must truly understand the temporal sequence of events rather than relying on simple pattern matching.
Technical Implementation
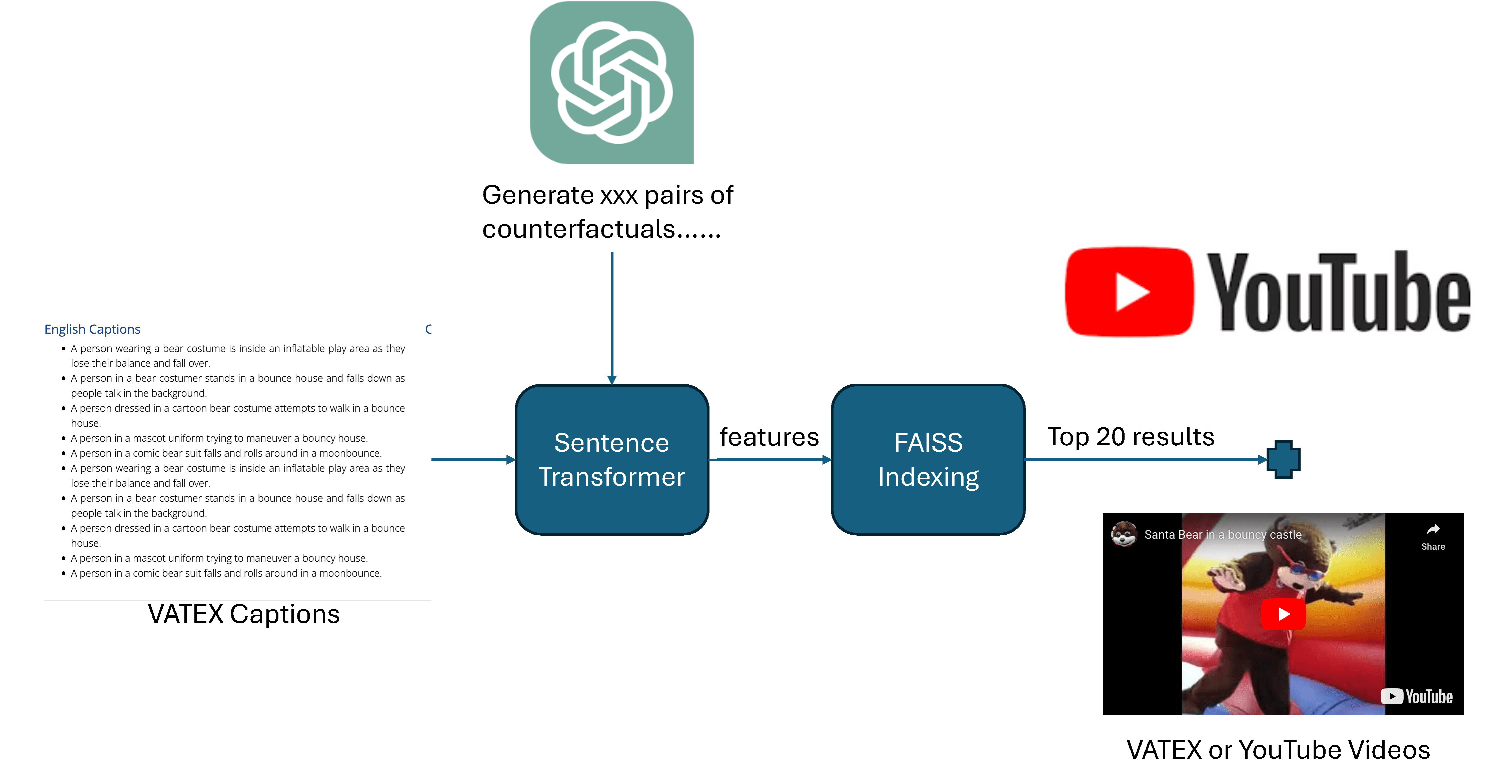
The data curation process involves a sophisticated pipeline where GPT-4 first generates counterfactual caption pair candidates. These candidates are then matched with appropriate videos using VATEX captions as an index, leveraging sentence transformers and the FAISS library for efficient similarity search. When direct matches aren't found in existing datasets, the team conducts YouTube searches using the captions to find suitable videos.
The benchmark comprises 500 carefully curated video-caption pairs, featuring:
Temporal differences in actions and objects (e.g., "the man eats then watches TV" vs. "the man watches TV then eats")
Object and viewpoint transformations (e.g., "water turning into ice" vs. "ice turning into water")
Spatial transformations that test understanding of physical relationships
Comprehensive Evaluation Framework
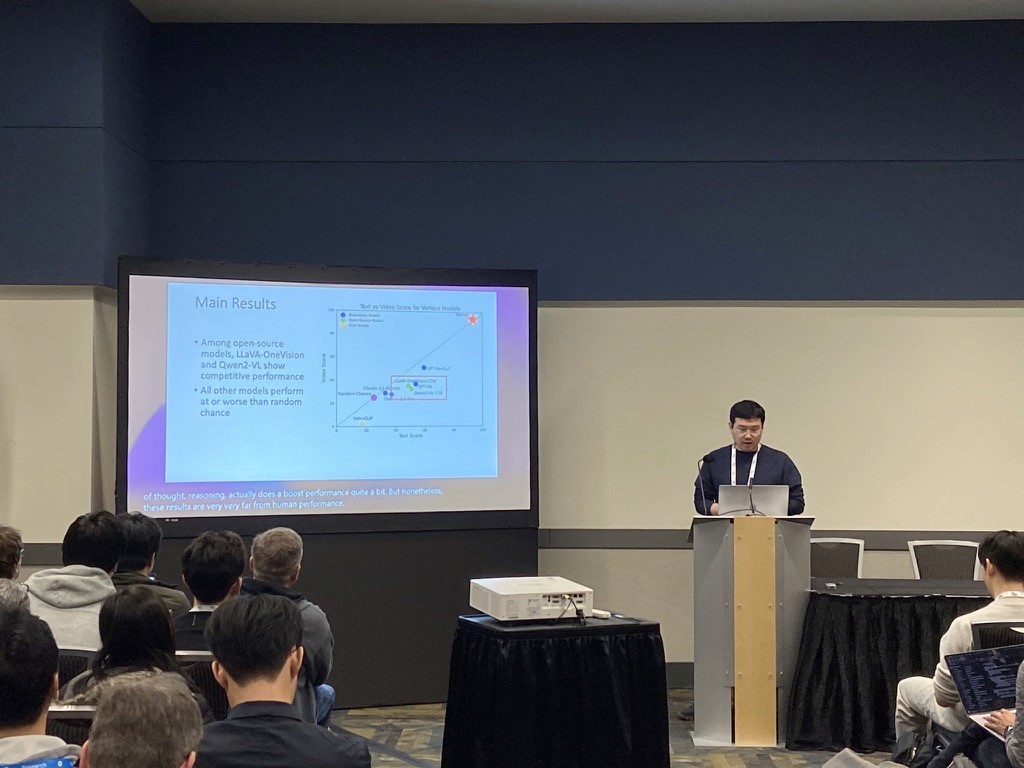
The evaluation framework employs three distinct metrics to provide a balanced assessment:
Text score: Measures the model's ability to understand linguistic differences
Video score: Evaluates visual comprehension capabilities
Group score: Assesses overall temporal understanding
Matryoshka Multimodal Models (M3)
The second major contribution addresses a fundamental bottleneck in current multimodal models: the overwhelming number of tokens, which not only makes Language-Vision Models (LMMs) inefficient but also dilutes their attention to relevant information. The Matryoshka Multimodal Models (M3) presents an elegant solution to this challenge.
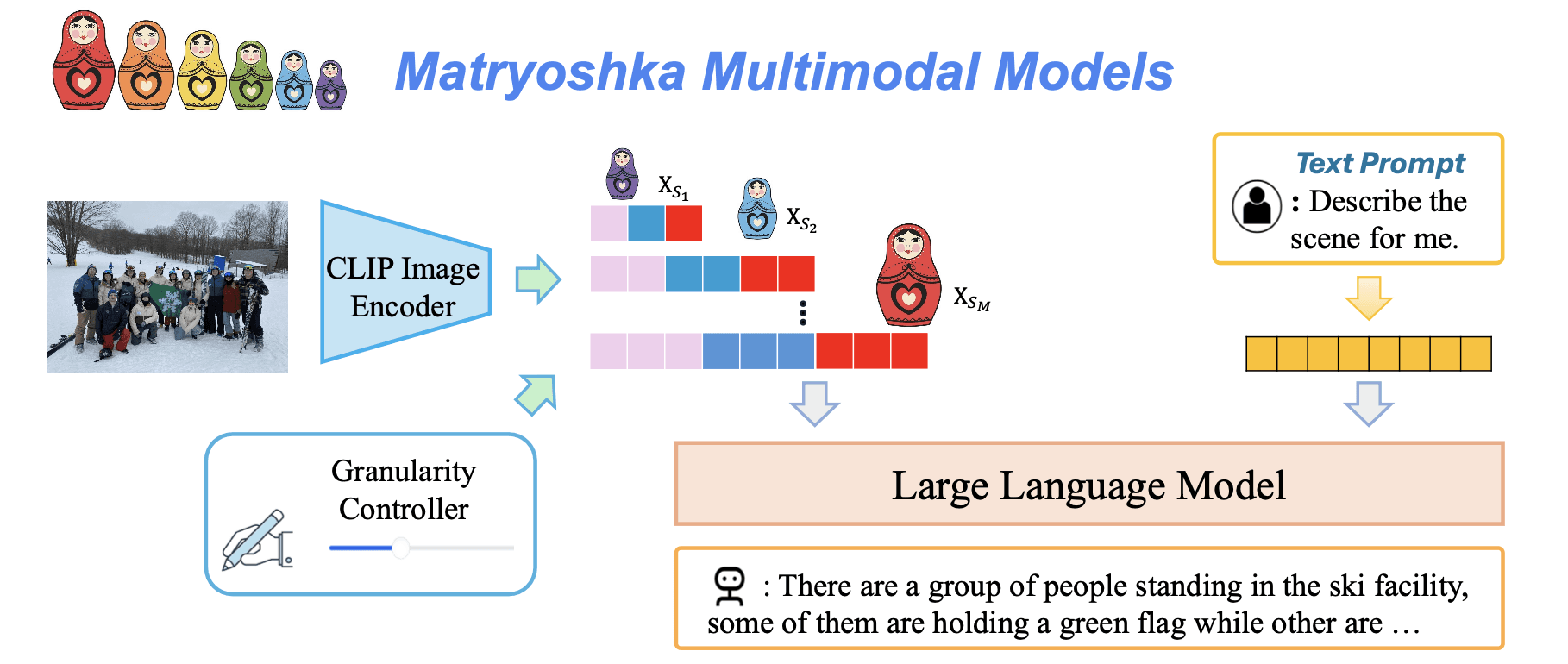
Technical Architecture
M3's training methodology is remarkably straightforward, taking the average of language generation loss across various visual token scales. The approach uses average pooling to obtain multi-granularity visual tokens, enabling a nested representation that proceeds from coarse to fine details.
Implementation Benefits
The implementation offers several significant advantages:
First, it maintains a simple design that uses vanilla LMM architecture and training data, requiring minimal code modifications and eliminating the need for a visual scale encoding module. Second, it provides unprecedented controllability, allowing researchers to explicitly adjust visual granularity during inference on a per-instance basis. Third, it serves as an analytical tool for understanding dataset requirements, revealing that many COCO-style benchmarks need only around 9 visual tokens to achieve performance comparable to using all 576 tokens.
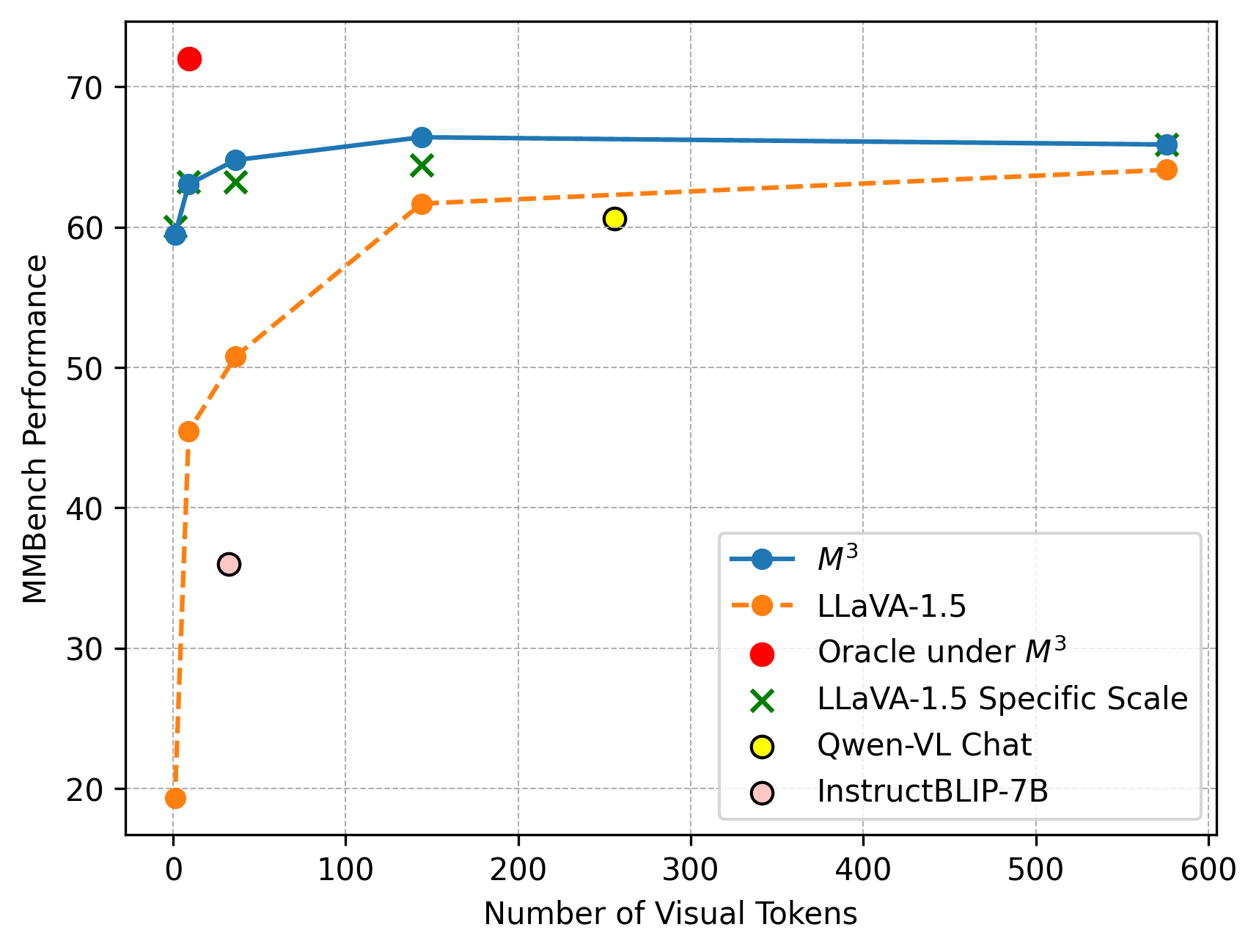
Empirical Results
Extensive testing on the MMBench revealed several crucial findings. The M3 variant of LLaVA-1.5-7B maintains or exceeds the performance of models trained on specific scales, while using significantly fewer resources. Particularly noteworthy is that M3 with just 9 visual tokens outperforms established baselines like InstructBLIP and Qwen-VL.
In video understanding tasks, full visual tokens often proved counterproductive. On four out of six benchmarks, using 720 or 180 tokens showed better performance than using the full token set, suggesting that excessive visual context might actually distract from accurate predictions. Most remarkably, for tasks like ActivityNet, IntentQA, and EgoSchema, using just 9 tokens per image grid (45 tokens total) performed within 1% accuracy of using the full 2880 tokens.
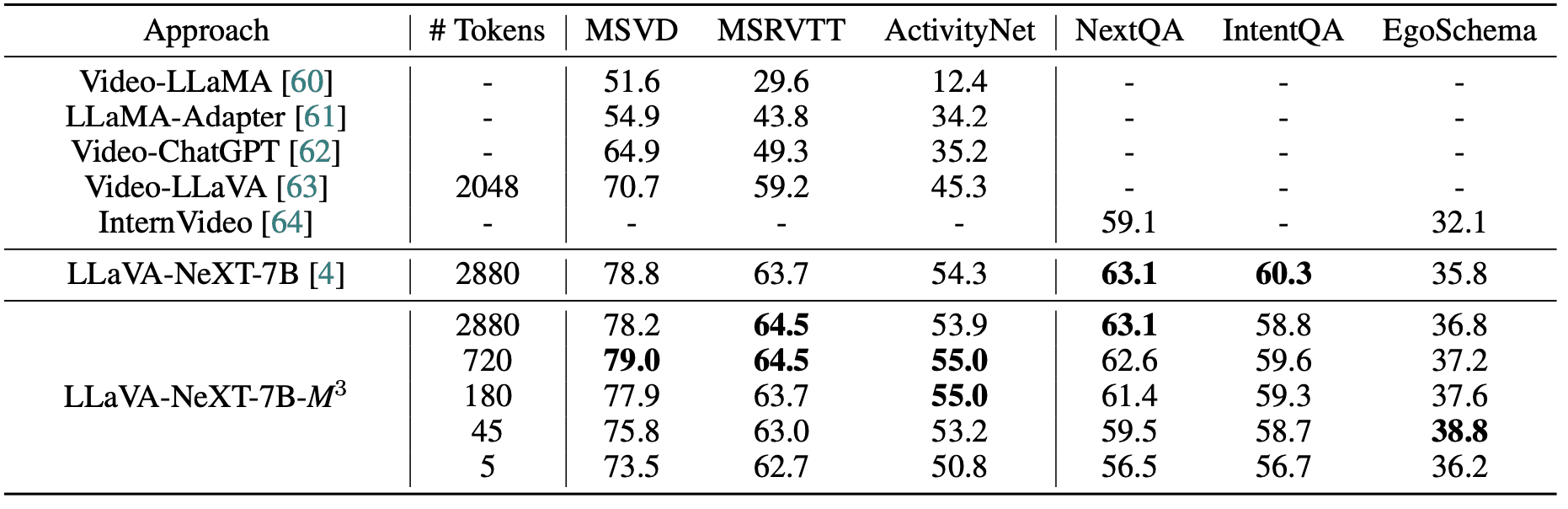
Broader Implications and Future Directions
The research presents several critical insights about the current state of video understanding models. While they show impressive capabilities in certain areas, they still fall significantly short of human-level intelligence. The Vinoground results particularly highlight how even short counterfactual temporal understanding remains challenging for current state-of-the-art models. Meanwhile, the M3 findings suggest that efficient processing of visual information might be more important than processing more visual tokens, pointing toward a promising direction for future model development.
4 - MovieGen: Foundation Model for Video Generation - Ishan Misra
Ishan Misra's presentation detailed Meta's MovieGen project, a groundbreaking initiative in text-to-video generation. The project addresses the fundamental challenge of generating videos from text input, with applications spanning creative content creation, video editing, image animation, simulation, and tools for both social media creators and Hollywood productions.
Evolution of Video Generation
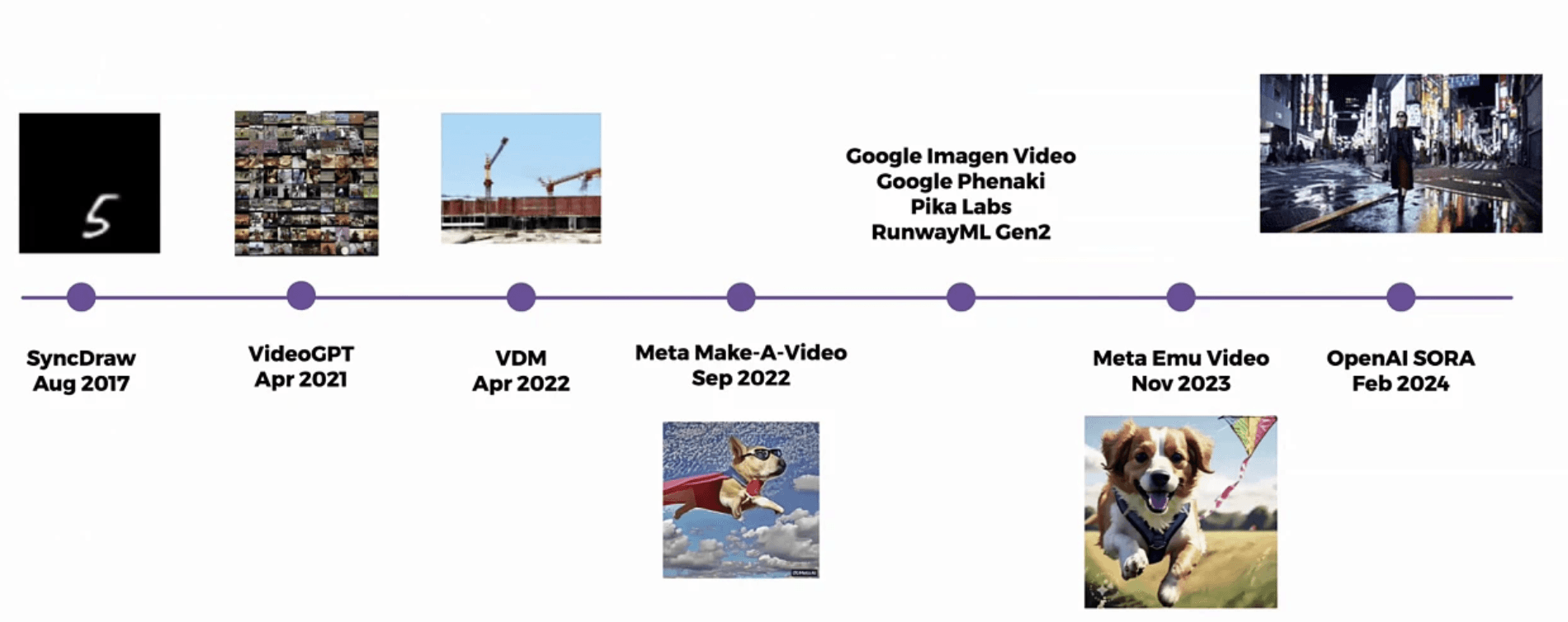
The presentation began with a comprehensive timeline of video generation models, highlighting the rapid progress in the field and setting the stage for MovieGen's contributions. This historical context emphasizes how the field has evolved from basic video synthesis to increasingly sophisticated generation capabilities.
Core Challenges in Video Generation
The field of video generation faces several fundamental challenges that MovieGen seeks to address. The first is the complex transformation from low-dimensional text input to high-dimensional video output, requiring sophisticated architectural solutions. Second, maintaining visual consistency and quality throughout generated videos demands robust temporal modeling. Third, the computational complexity of video generation necessitates efficient processing strategies and optimized architectures.
Technical Architecture and Innovations
1. Architectural Framework
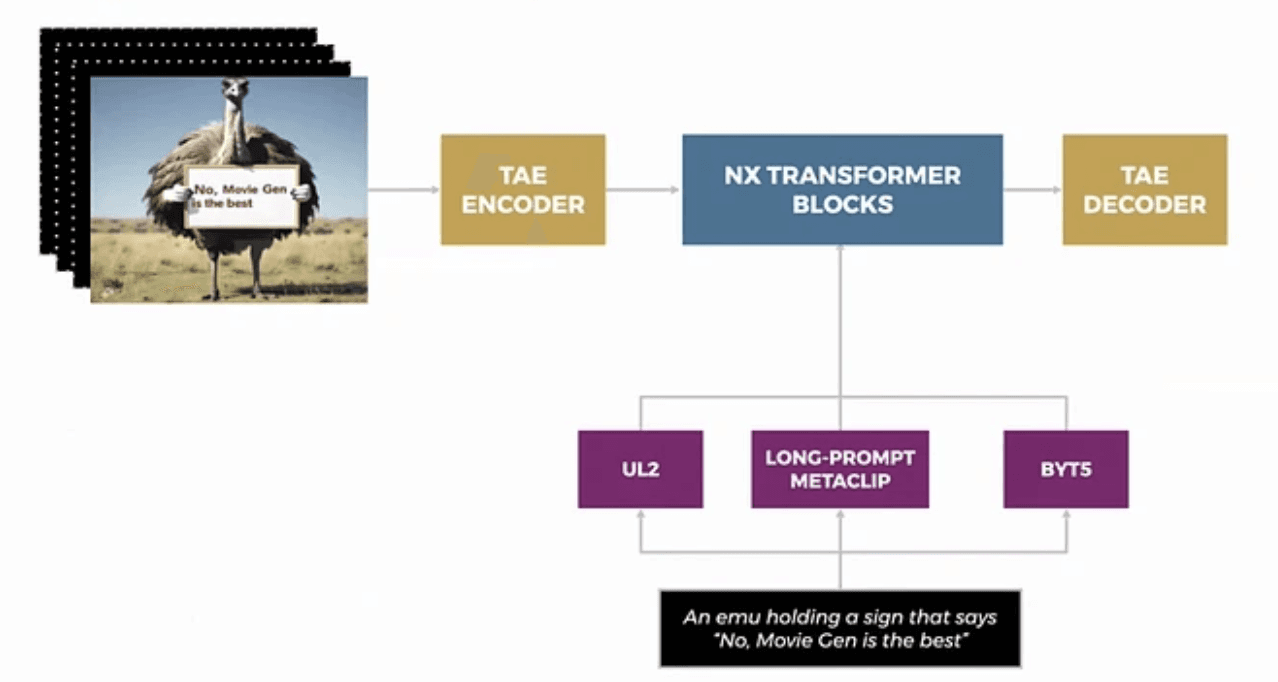
MovieGen's architecture represents a significant advancement in video generation, incorporating several innovative elements. The model employs enhanced text encodings that capture multiple aspects of input text prompts, enabling more nuanced understanding of generation requirements. The architecture is built on LLaMA-style Transformer blocks, which have been specifically adapted for video generation tasks, providing improved scalability and stability during training.
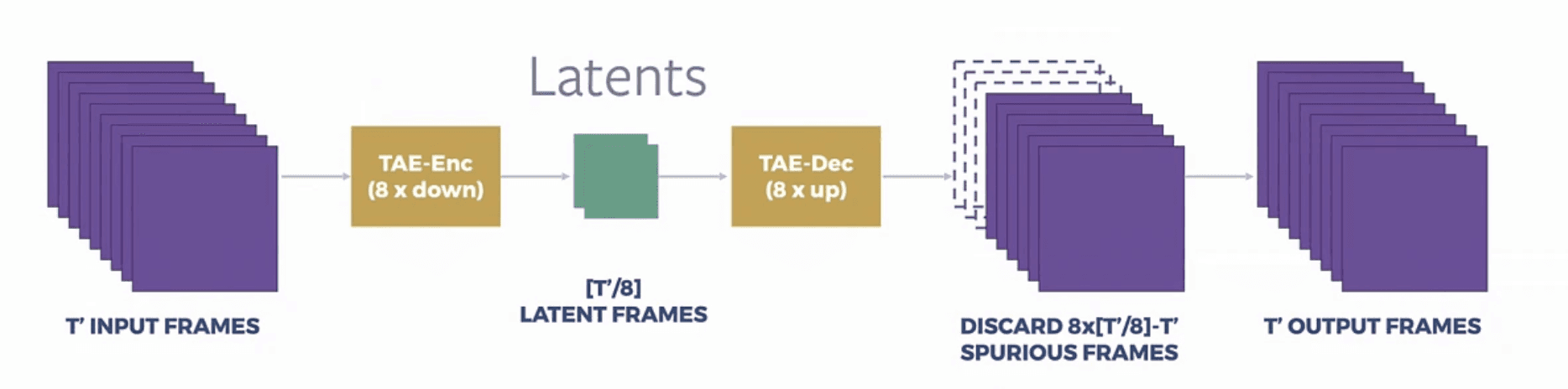
A key innovation is the model's approach to latent compression. MovieGen achieves an 8x reduction in video dimensions across height, width, and time dimensions, resulting in a remarkable 512x reduction in sequence length. This compression capability extends to both videos and images, allowing for variable-length sequence processing while maintaining generation quality.
2. Training Methodology
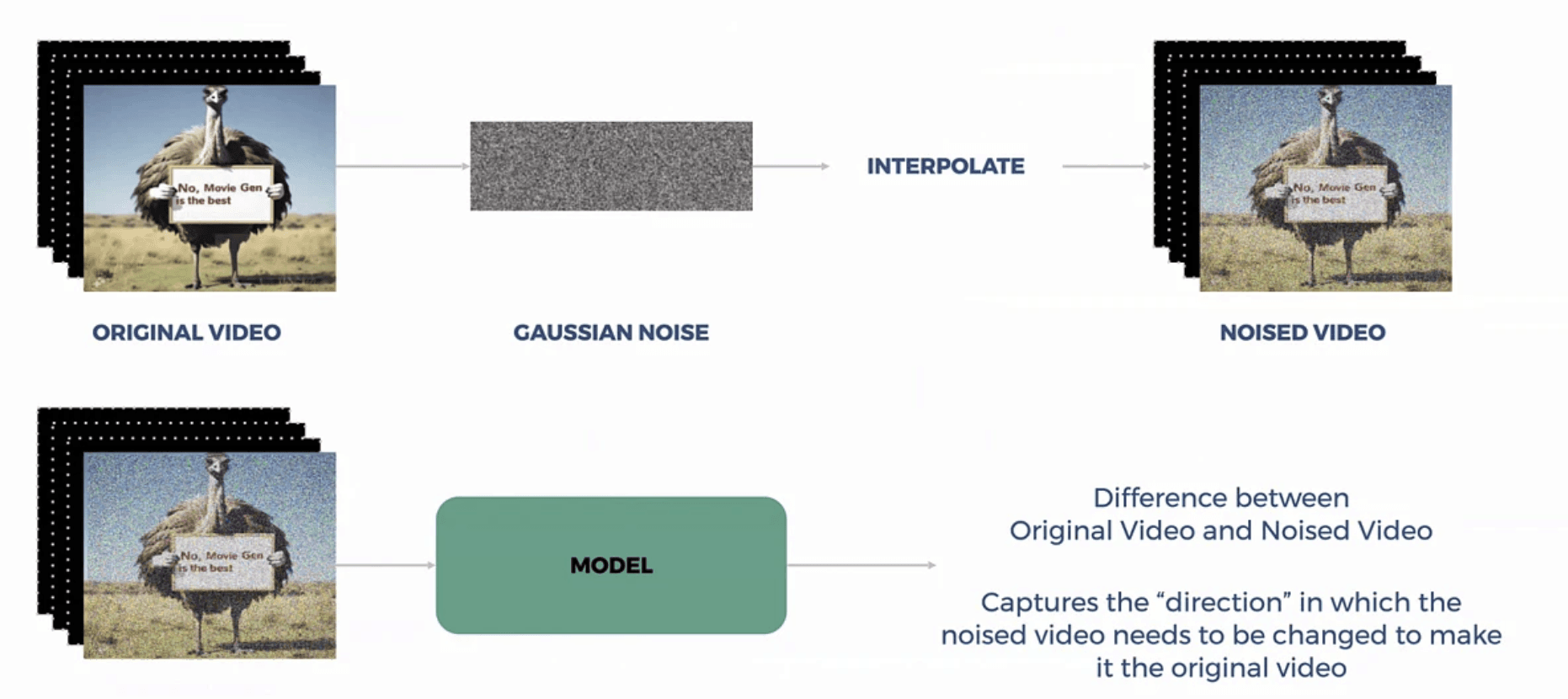
The training approach incorporates LLaMA-style blocks enhanced with diffusion Transformer (DiT) scale/shift modifications. This combination enables stable training at scale while allowing all parameters to be trained simultaneously on both images and videos, eliminating the need for frozen components. This end-to-end training approach contributes to the model's coherent understanding of both static and dynamic visual content.
3. Flow Matching Innovation
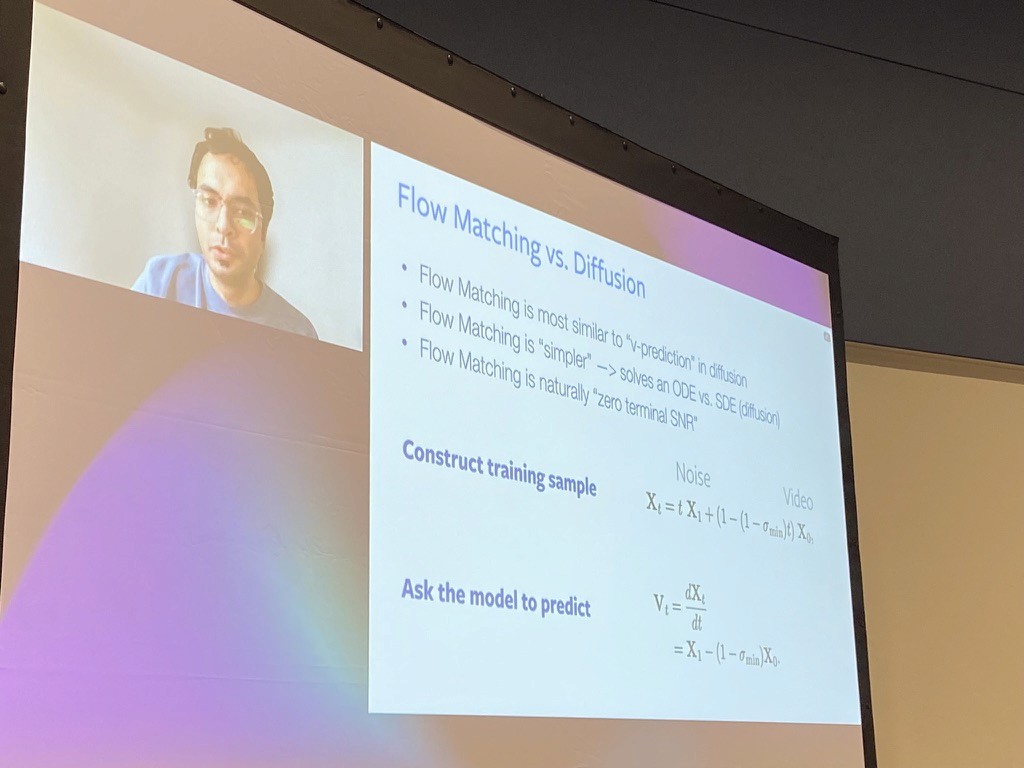
One of MovieGen's most significant technical innovations is its implementation of flow matching as an alternative to traditional diffusion approaches. This method is mathematically similar to "v-prediction" in diffusion but offers several crucial advantages. Instead of solving a stochastic differential equation (SDE) as in diffusion models, flow matching solves an ordinary differential equation (ODE), resulting in a simpler and more efficient process. The approach naturally achieves "zero terminal Signal-to-Noise ratio" characteristics, leading to both improved generation quality and faster inference speeds compared to diffusion-based methods.
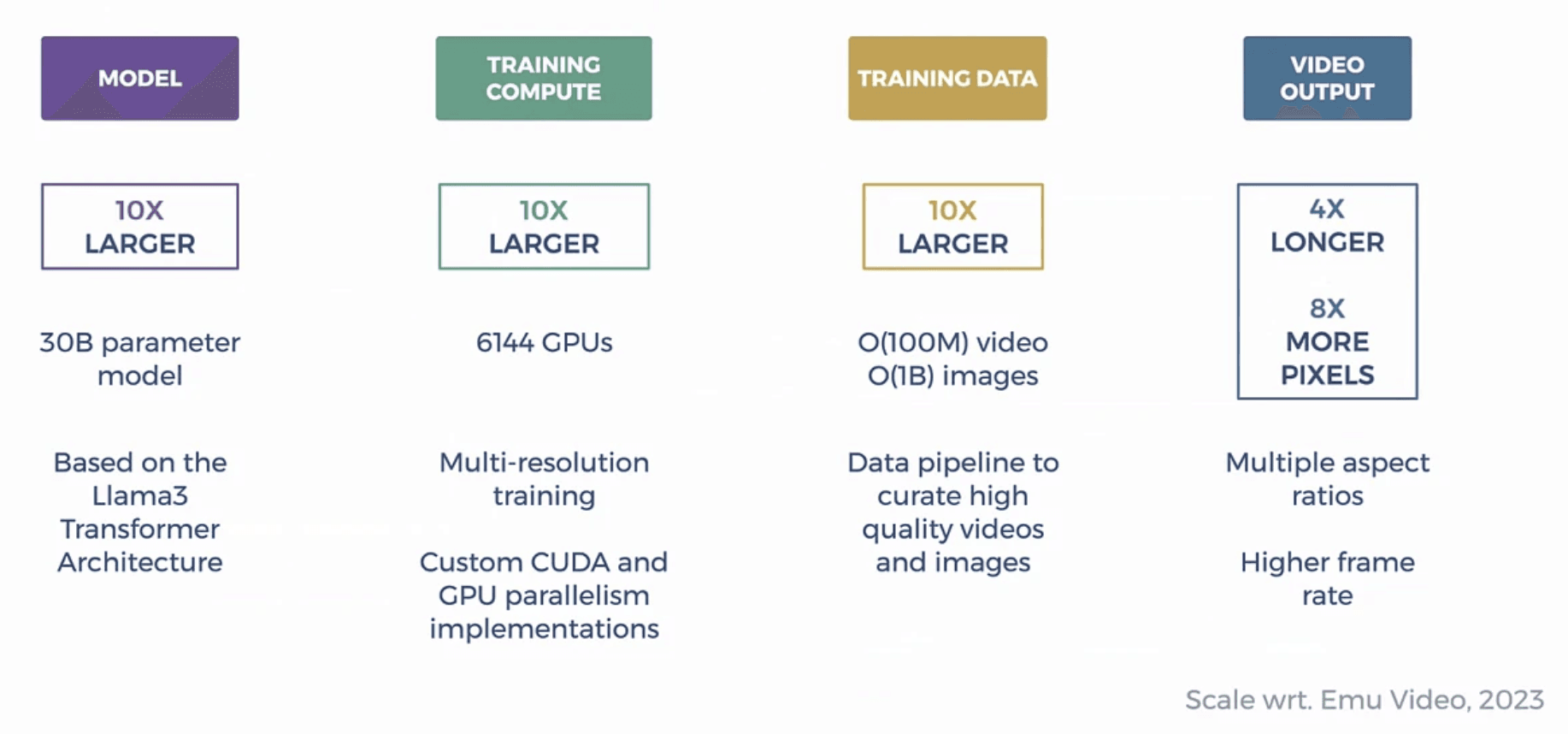
Training Data and Scaling
MovieGen's training methodology represents a significant scaling effort in both data and computation. The training dataset encompasses approximately 100 million videos and 1 billion images, with videos varying in length and frame rate to ensure robust generalization. A notable innovation is the use of LLaMA-3 for video caption generation, which substantially improves the model's ability to follow text prompts accurately.
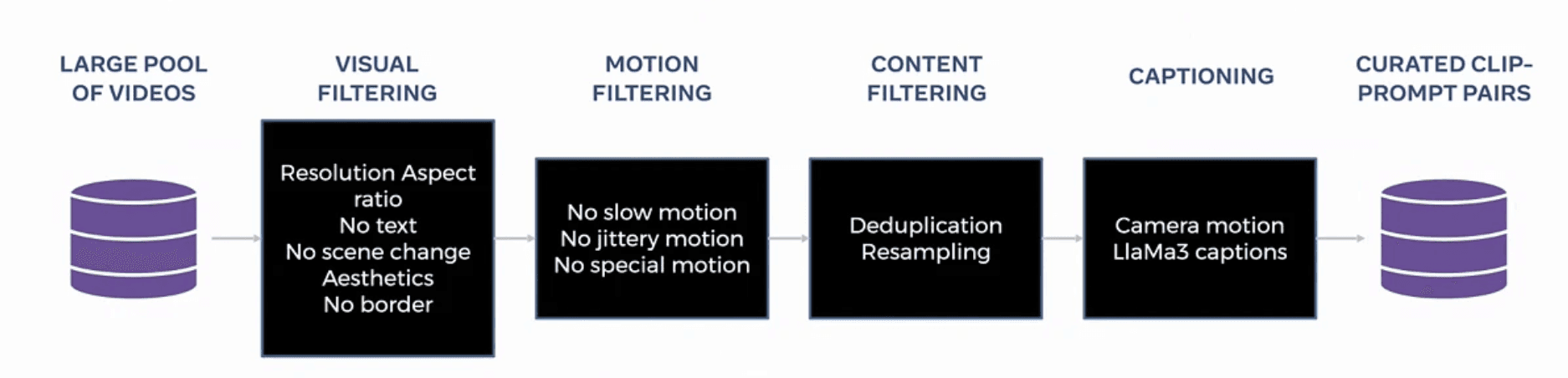
Comprehensive Evaluation Framework
The Meta team developed MovieGen Bench, a rigorous evaluation framework that surpasses previous benchmarks in both scale and comprehensiveness. This benchmark includes 1,000 non-cherry-picked videos, available publicly on GitHub, and offers three times more evaluation data than previous benchmarks. The evaluation methodology demonstrated a strong correlation between validation loss and human judgment, providing a reliable metric for model performance.
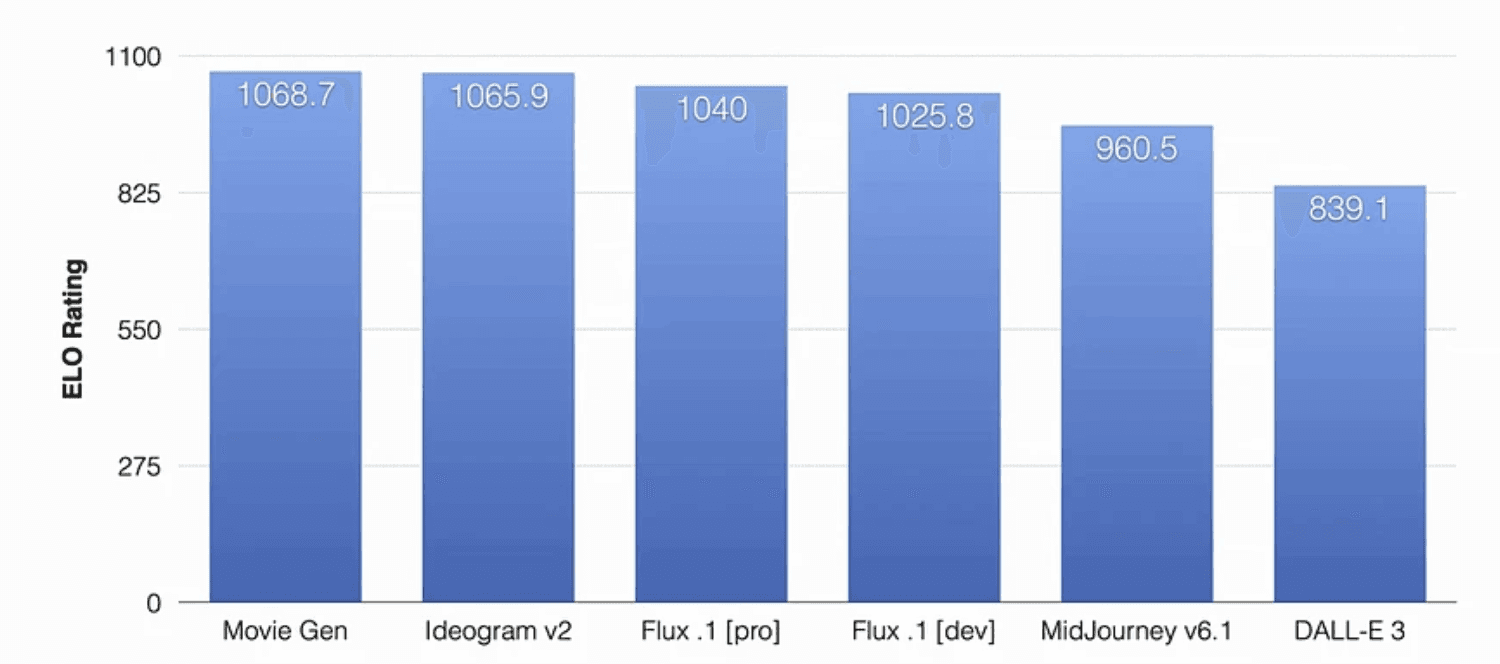
Performance and Future Directions
The evaluation results revealed impressive capabilities in several key areas. MovieGen showed competitive performance in both overall quality and realness metrics, with particularly strong results in text-to-image generation capabilities. The research uncovered important insights about scaling laws, notably that model performance has not yet reached saturation and that video generation scaling laws closely mirror those observed in LLaMA-3.
While MovieGen represents a significant advance in video generation capabilities, Ishan acknowledges important limitations and future challenges. Particularly, the extent of the model's ability to combine and generalize its understanding of the world remains an active area of investigation. This honest assessment of current limitations helps guide future research directions in the field of video generation.
5 - Towards Multimodal Agentic Models - Jianwei Yang
Jianwei Yang's presentation challenged the traditional focus on pure understanding in AI systems, arguing that interaction, not understanding alone, should be the ultimate goal. His comprehensive talk outlined a roadmap for developing multimodal AI agents capable of both understanding the past and acting for the future, with applications spanning web agents, robotics, and autonomous driving.
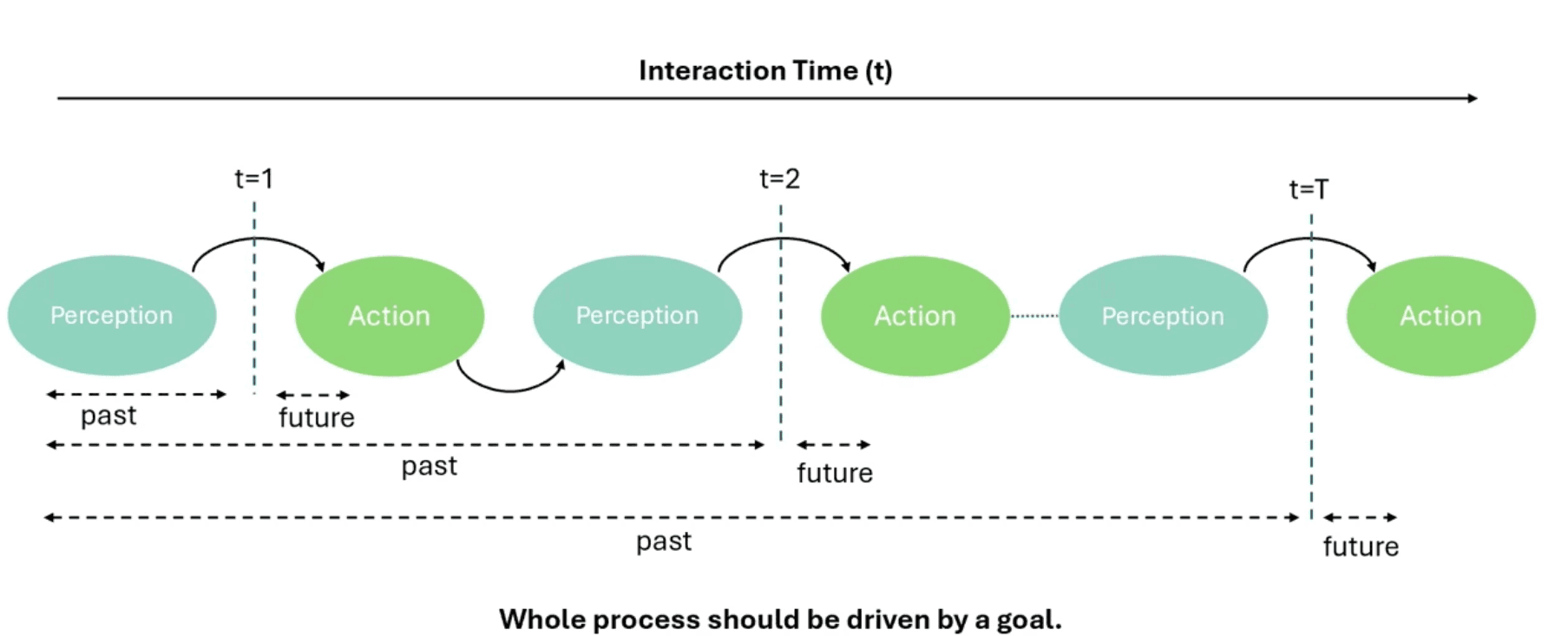
Understanding the Past: Enhanced Visual Perception
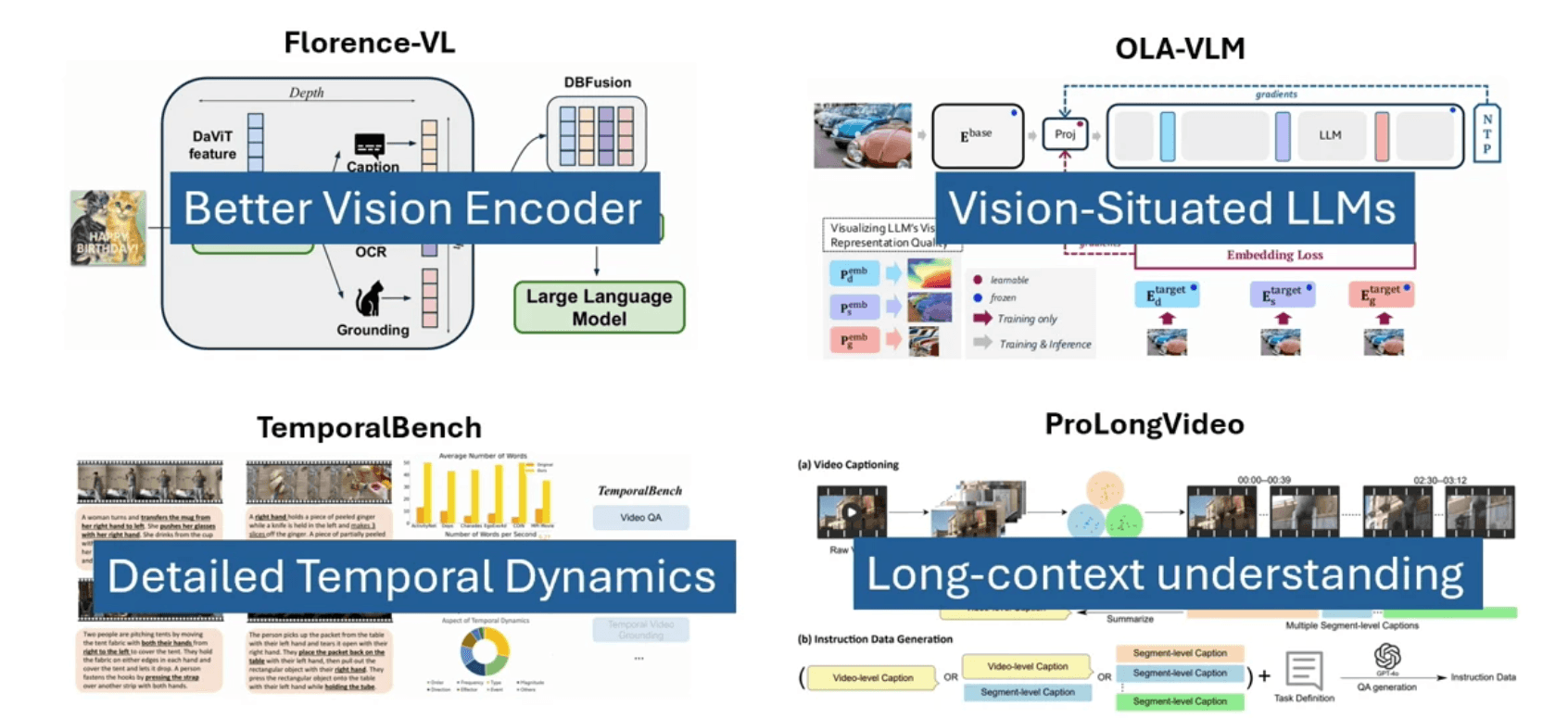
Florence-VL: Enhanced Vision-Language Models
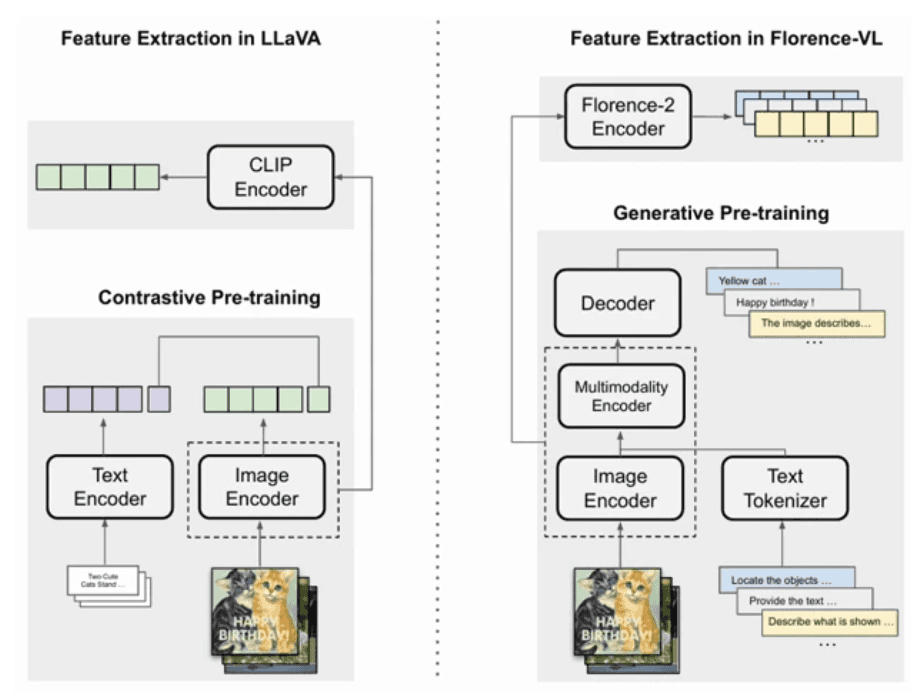
Florence-v2 represents a significant advancement in text-conditioned generative vision-language models. The model's architecture introduces a novel approach to visual perception through different prompts designed for various types of visual features. These features work complementarily, creating a richer overall representation of visual information. The model's effectiveness is measured through alignment cost between output features from the vision encoder and LLM encoder, providing a quantitative metric for evaluation.
OLA-VLM: Deepening Visual Understanding
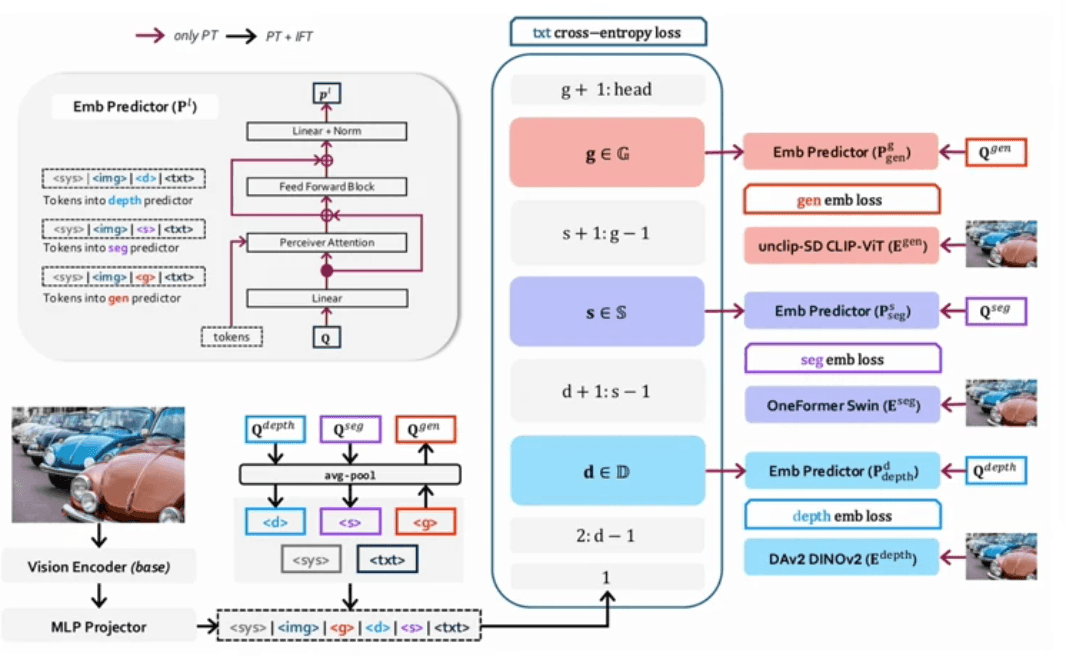
OLA-VLM pushes the boundaries of visual perception in multimodal LLMs by incorporating three distinct visual features for each image:
Depth features that capture spatial relationships
Segmentation features for object delineation
Unclip-SD features for enhanced visual representation
The research demonstrates that increasing text supervision leads to improved intermediate features for vision perception. A key innovation is the direct optimization of LLM features using "teacher" models, resulting in more robust visual understanding capabilities.
Fine-grained Temporal Dynamics
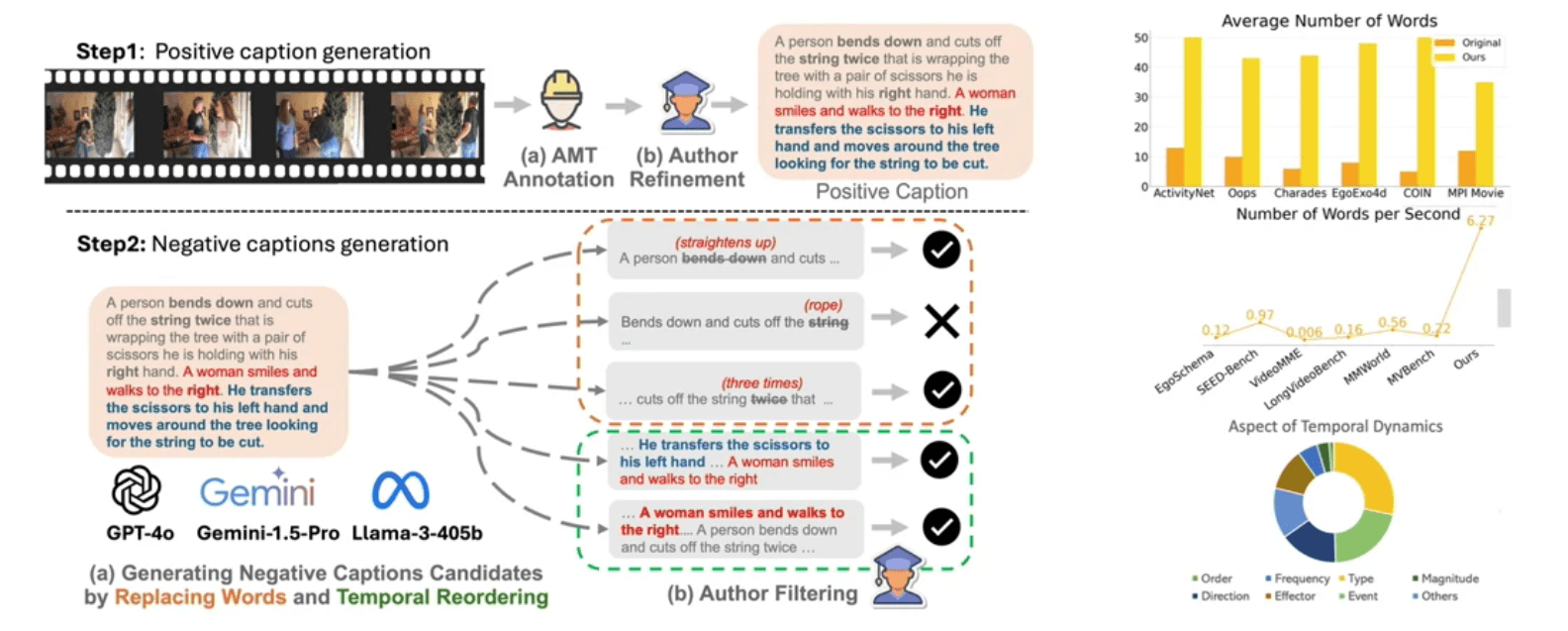
The TemporalBench project addresses a fundamental aspect of video understanding: videos contain not just static contents in individual frames but crucial fine-grained dynamics. This benchmark provides a comprehensive evaluation framework for assessing temporal understanding capabilities in multimodal video models.
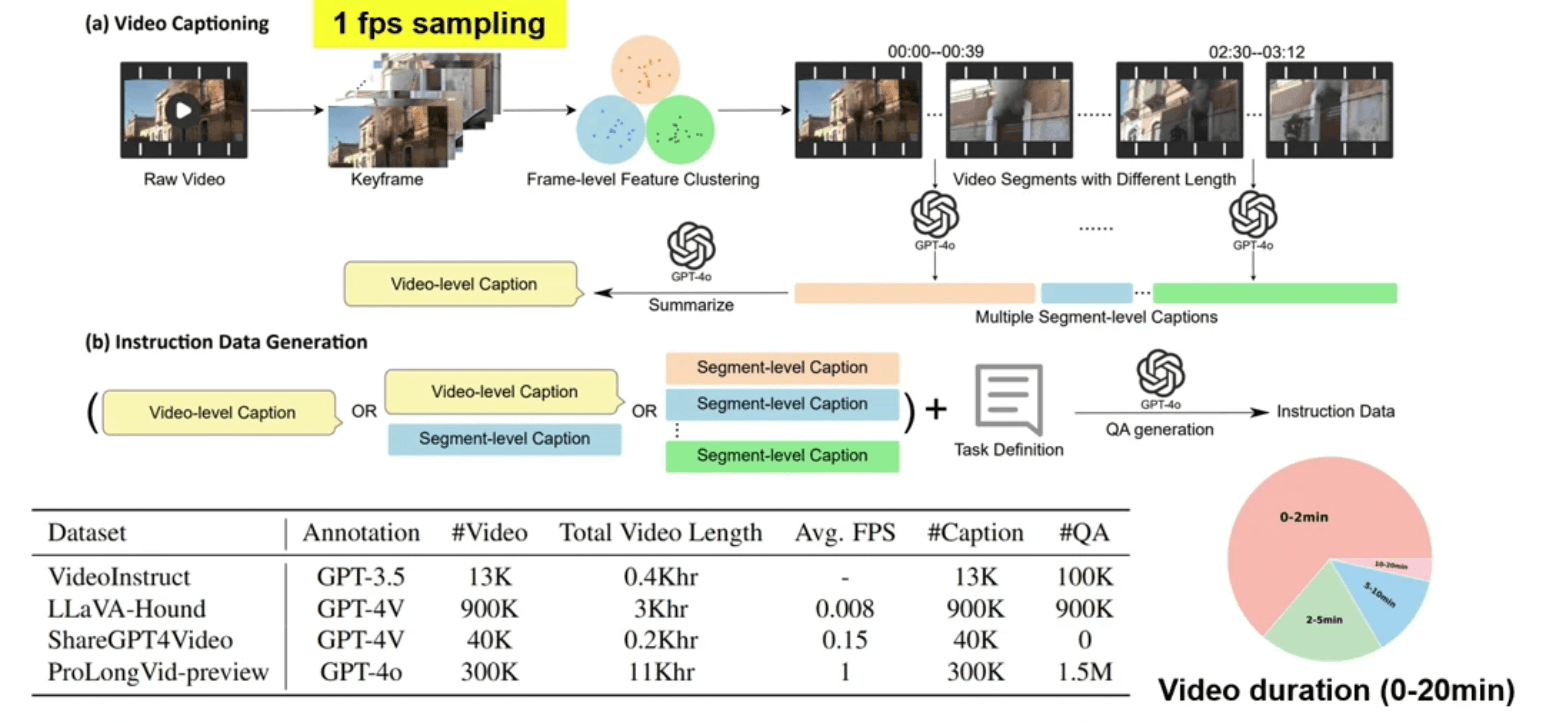
ProLongVid complements this work by focusing on two critical scaling dimensions:
Scaling video length during instruction data synthesis, enabling models to handle longer temporal sequences
Scaling context length during both training and inference, improving the model's ability to maintain coherent understanding across extended temporal spans
Acting for the Future: Multimodal Agentic Models
TraceVLA: Visual Trace Prompting
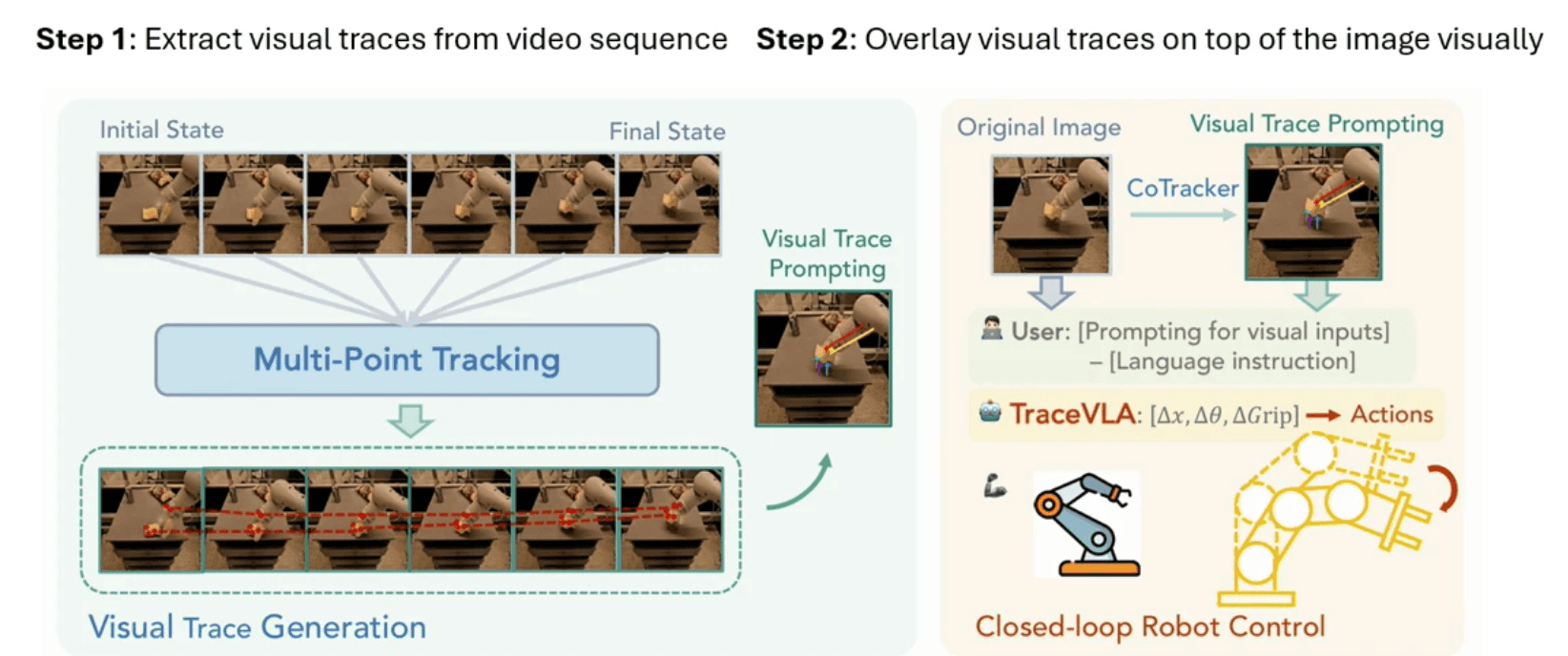
TraceVLA introduces a novel approach to enhancing spatial-temporal awareness for robotic policies. The core intuition behind this work is that better understanding of history facilitates better prediction of future actions. The system addresses several key challenges in multimodal processing:
Multi-image processing presents a significant challenge by increasing the number of visual tokens, while vision encoders struggle to capture subtle changes or motions across adjacent frames.
Text prompts, while easy to implement, fall short in capturing fine-grained movement information and spatial locations.
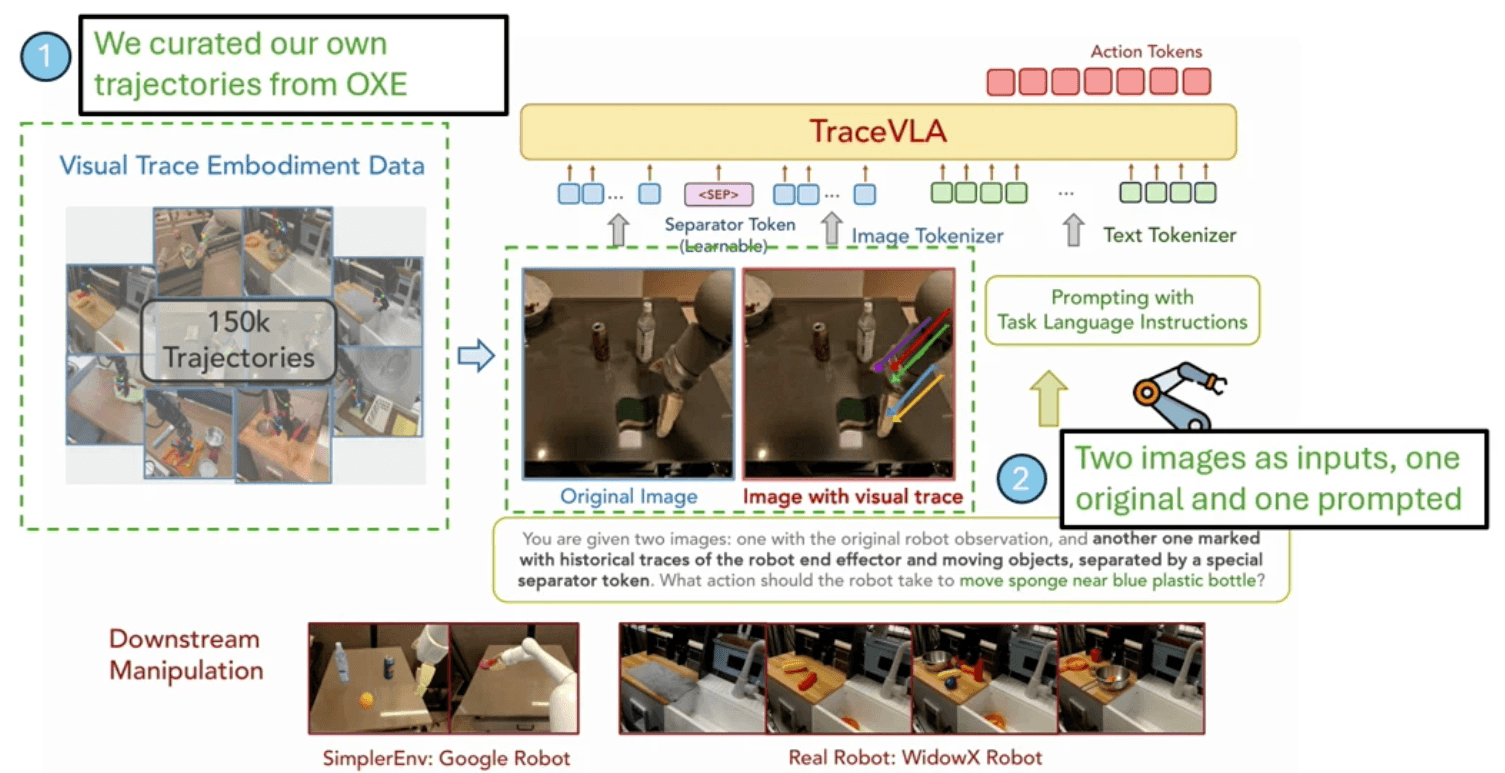
The innovation comes through visual prompts, which prove particularly effective through Set-of-Mark prompting, naturally building associations between current observations and historical context.
LAPA: Latent Action Pretraining
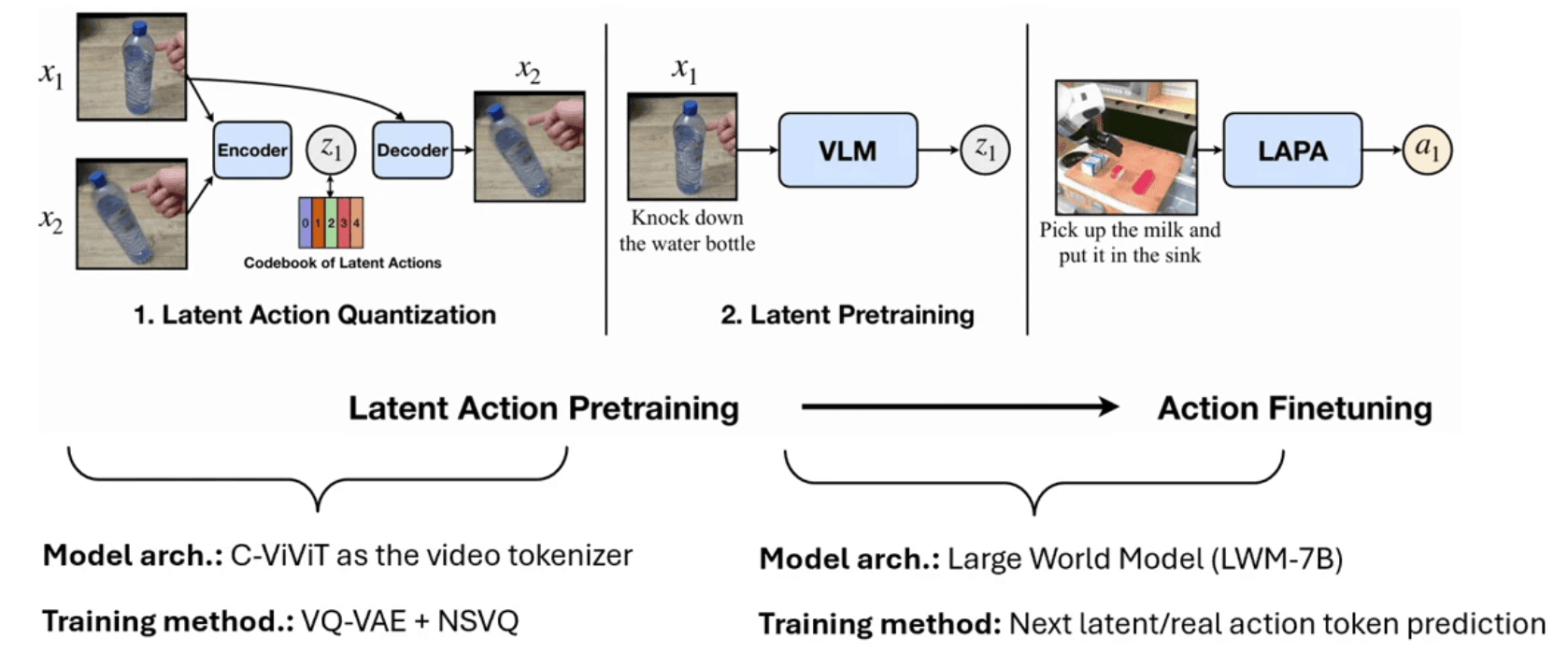
LAPA represents a breakthrough in leveraging unlabeled video data for robotics policy training. The system demonstrates impressive capabilities in learning from both robotics data without actions and human instructional videos. Using sthv2 as the human instructional video dataset, LAPA achieves significant improvements over models trained from scratch, though some gap remains compared to models pre-trained on robotics-specific data.
MAGMA: Foundation Model for Multimodal AI Agents
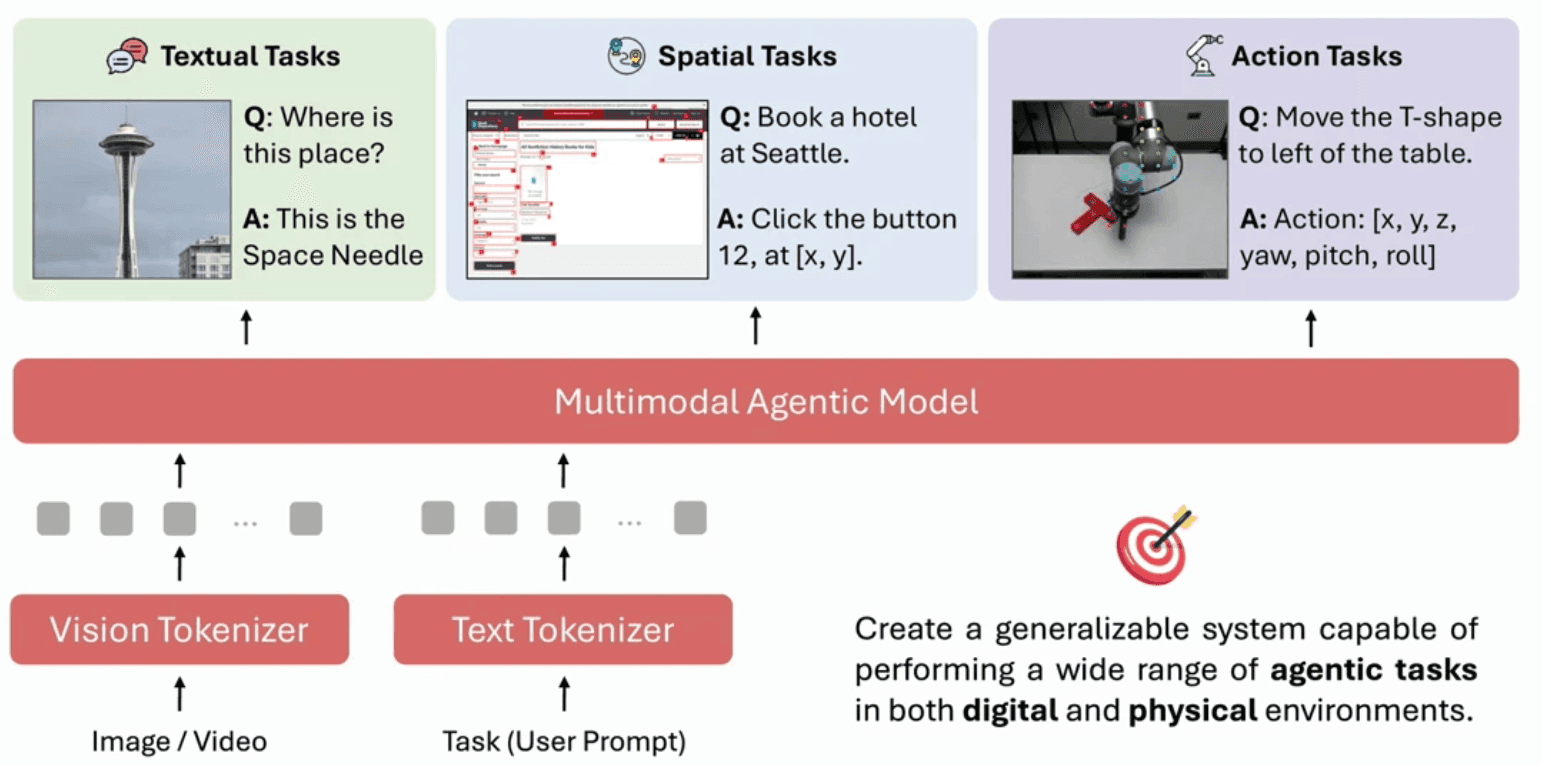
MAGMA introduces a comprehensive foundation model for multimodal AI agents, incorporating pre-training across a diverse range of images, videos, and robotics data. The model architecture is designed to facilitate both understanding and action, with particular emphasis on temporal motion supervision and action conversion. The pre-training tasks are carefully designed to develop emergent capabilities in robot trajectory planning, demonstrating effects of data scaling and task generalization.
Redefining Multimodal AI Agents
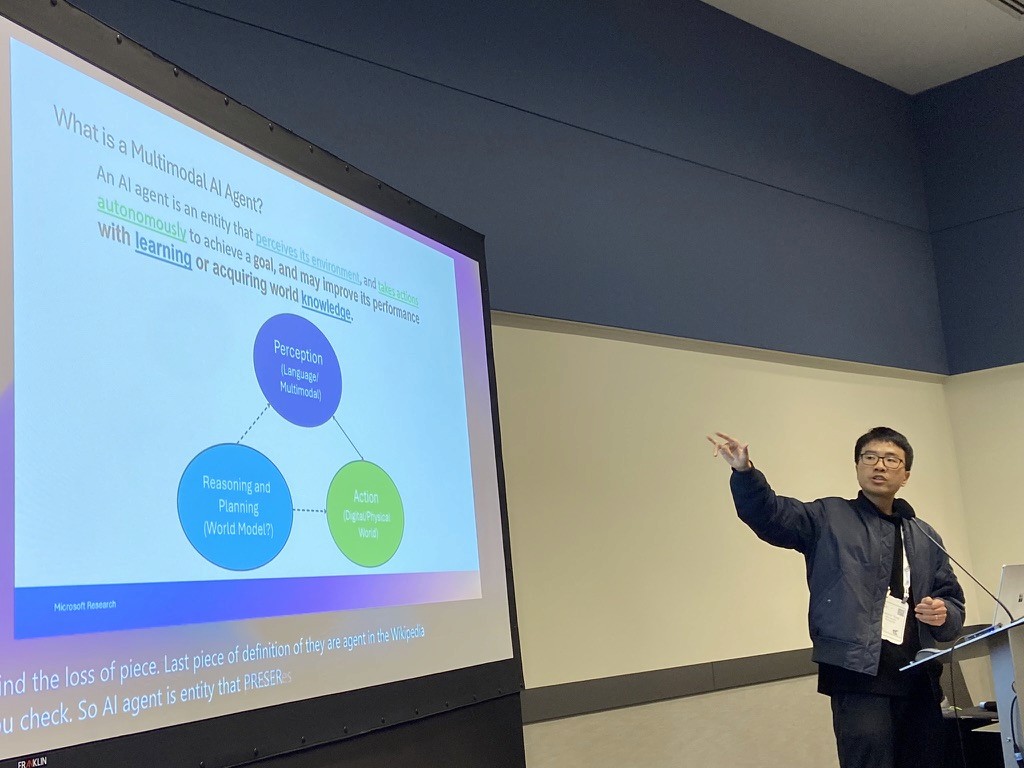
Yang concluded his talk by proposing an expanded definition of multimodal AI agents: "An AI agent is an entity that perceives its environment and takes actions autonomously to achieve a goal, and may improve its performance with learning or acquiring world knowledge." This definition emphasizes three critical aspects:
The transition from 2D to 3D perception for multimodal agents, acknowledging the importance of spatial understanding
The balance between model-free and model-based approaches in multimodal agent development
The crucial role of reasoning and planning in agent behavior
Future Directions and Challenges
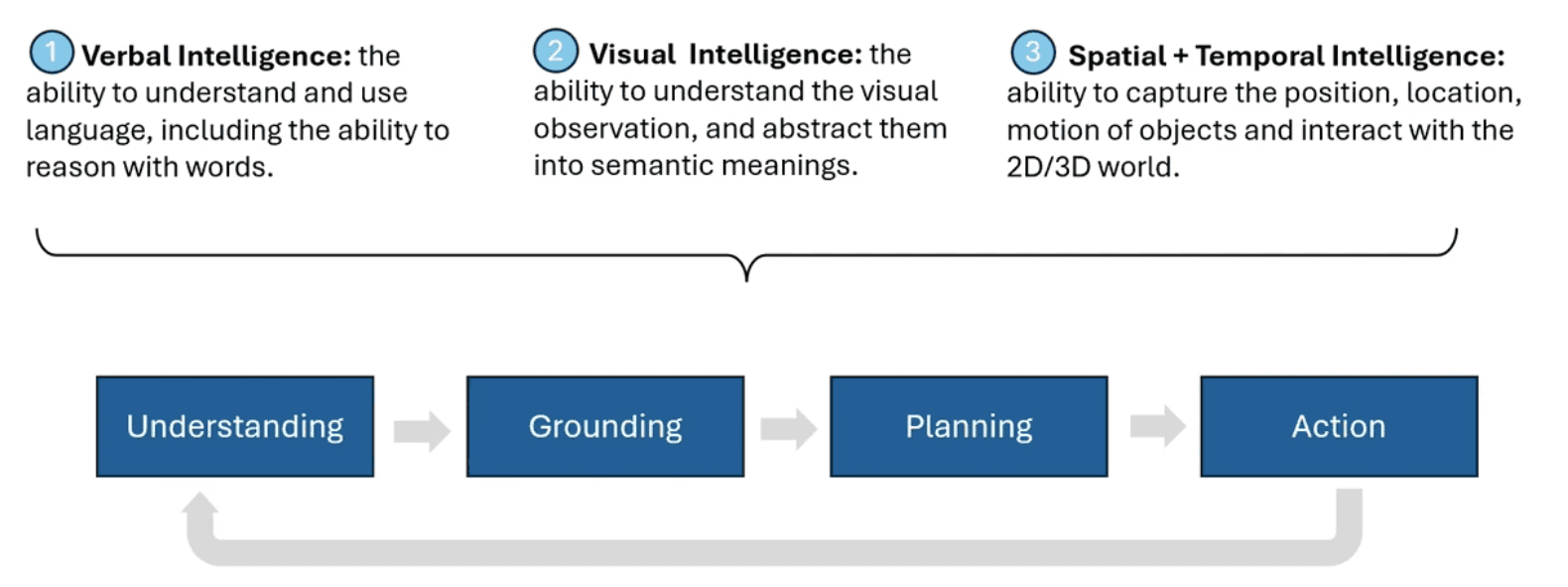
Yang emphasized that scaling remains a vital factor in advancing the field, particularly given the vast potential of video data and real-world interactions. He outlined several key directions for future research:
Multimodal Agentic Capabilities: Developing more sophisticated interaction abilities
Multimodal Reasoning: Enhancing the ability to make logical connections across modalities
Multimodal Understanding: Deepening comprehension of complex multimodal inputs
Multimodal Self-Awareness: Building systems with greater metacognitive capabilities
The presentation concluded with an important observation about current limitations: while visual understanding has improved significantly, it still falls short of human intelligence. Long-context video understanding, detail comprehension, and temporal dynamics remain challenging areas, particularly given the scarcity of high-quality video-text paired data despite the abundance of raw video content.
6 - From Video Generative Models to General World Models - Doyup Lee
Doyup Lee concluded our workshop exploring Runway’s journey from video generative models to general world models, centered around a fundamental question: "How can an intelligent machine understand the visual world?" His presentation delved deep into the nature of visual understanding and the role of imagination in AI systems, drawing from Runway's extensive experience in developing cutting-edge video generation models.
Understanding Visual Intelligence
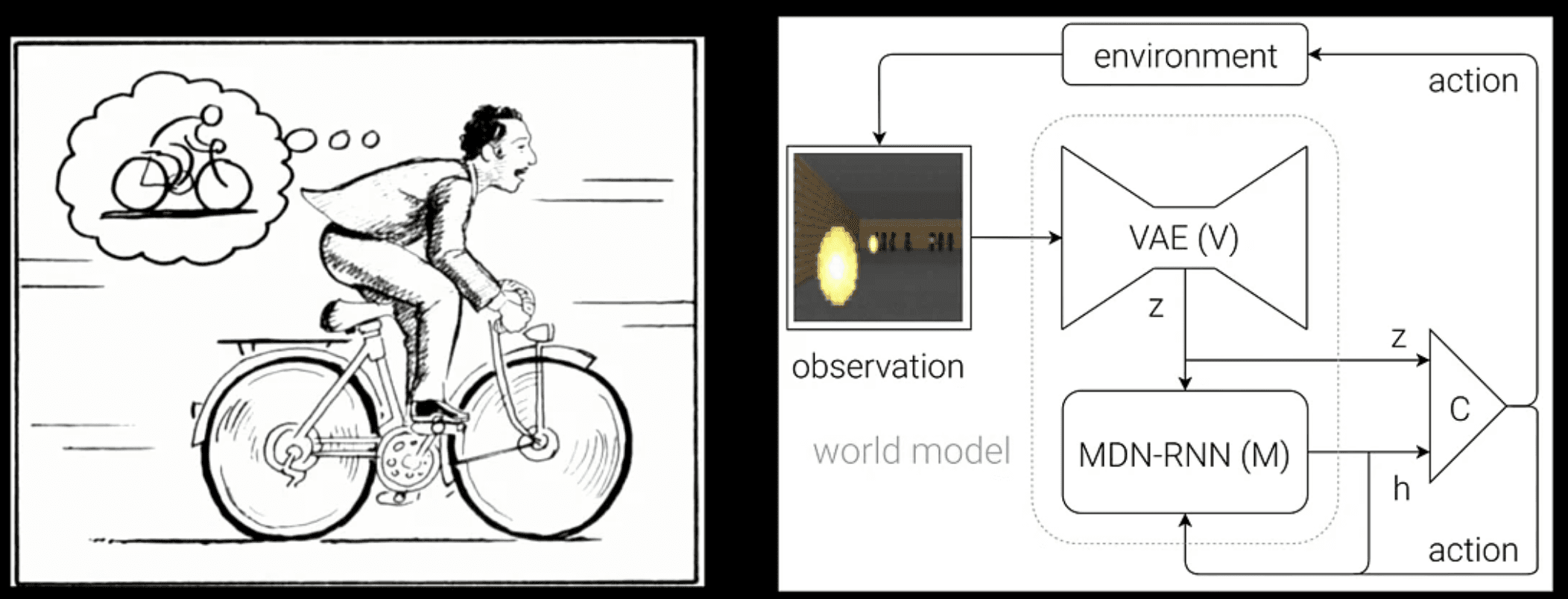
Lee began by examining what constitutes "visual understanding" through the lens of imagination and world modeling. He referenced the seminal work by Ha and Schmidhuber (2018) on World Models, which achieved impressive results in future predictions but remained confined to specific simulators or environments. Traditional visual recognition tasks - including image captioning, visual question answering, and semantic segmentation - have primarily focused on aligning visual information with human knowledge through text, but these approaches are inherently limited by the scope of visual data and world knowledge they can process.
The Platonic Representation Hypothesis
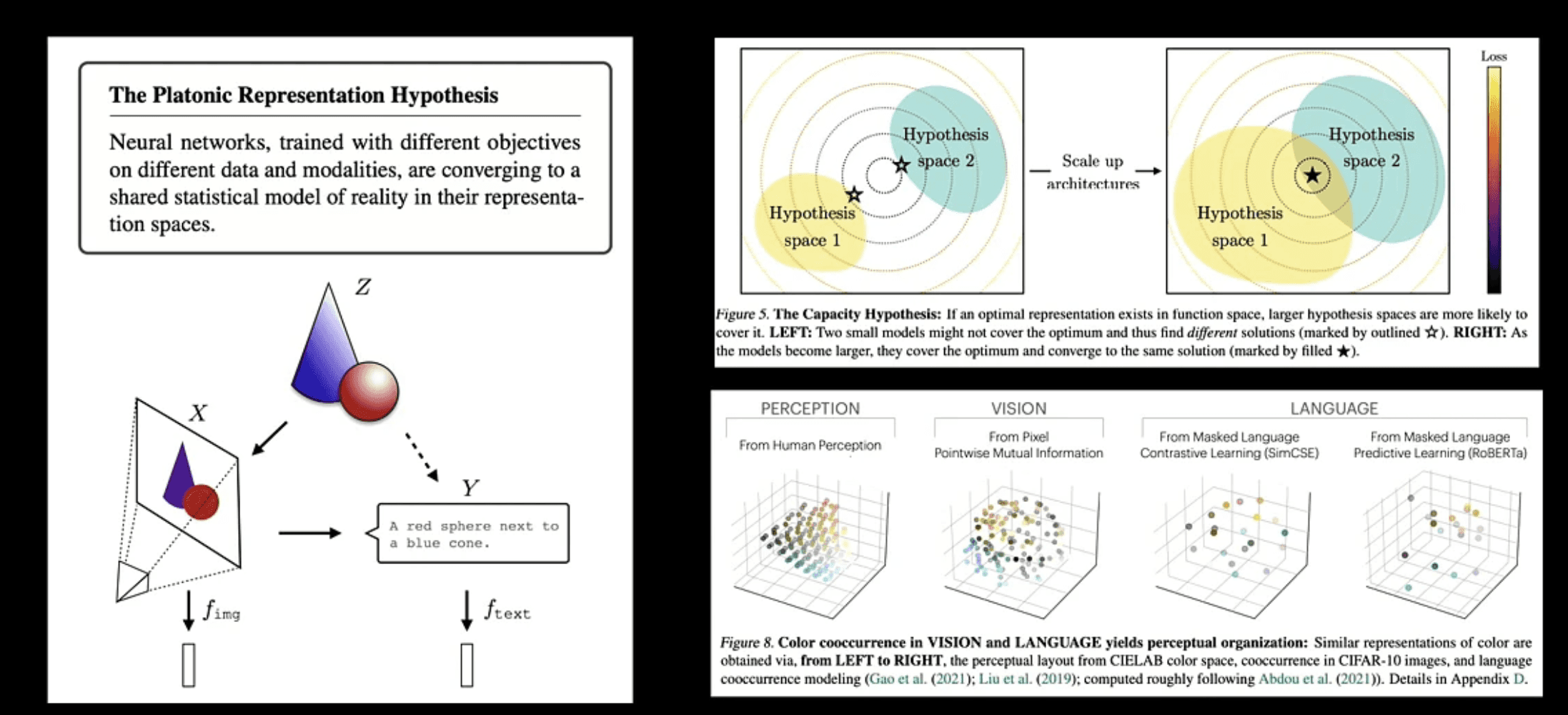
A cornerstone of Lee's theoretical framework is the Platonic Representation Hypothesis (Huh et al., ICML 2024), which identifies three essential components for visual understanding:
The ability to achieve high performance in future prediction tasks, demonstrating true comprehension of visual dynamics
Access to and effective utilization of large and diverse video datasets that capture the complexity of the visual world
Strong alignment with human knowledge, ensuring that the model's understanding corresponds to human concepts and expectations
The Five Essential Recipes for General World Models
1. The Bitter Lesson
Lee emphasized the importance of embracing the "bitter lesson" in AI development: that general methods leveraging computation and learning ultimately triumph over hand-crafted, specialized approaches. This principle guides the development of more flexible and scalable architectures.
2. High-quality and Large-scale Data
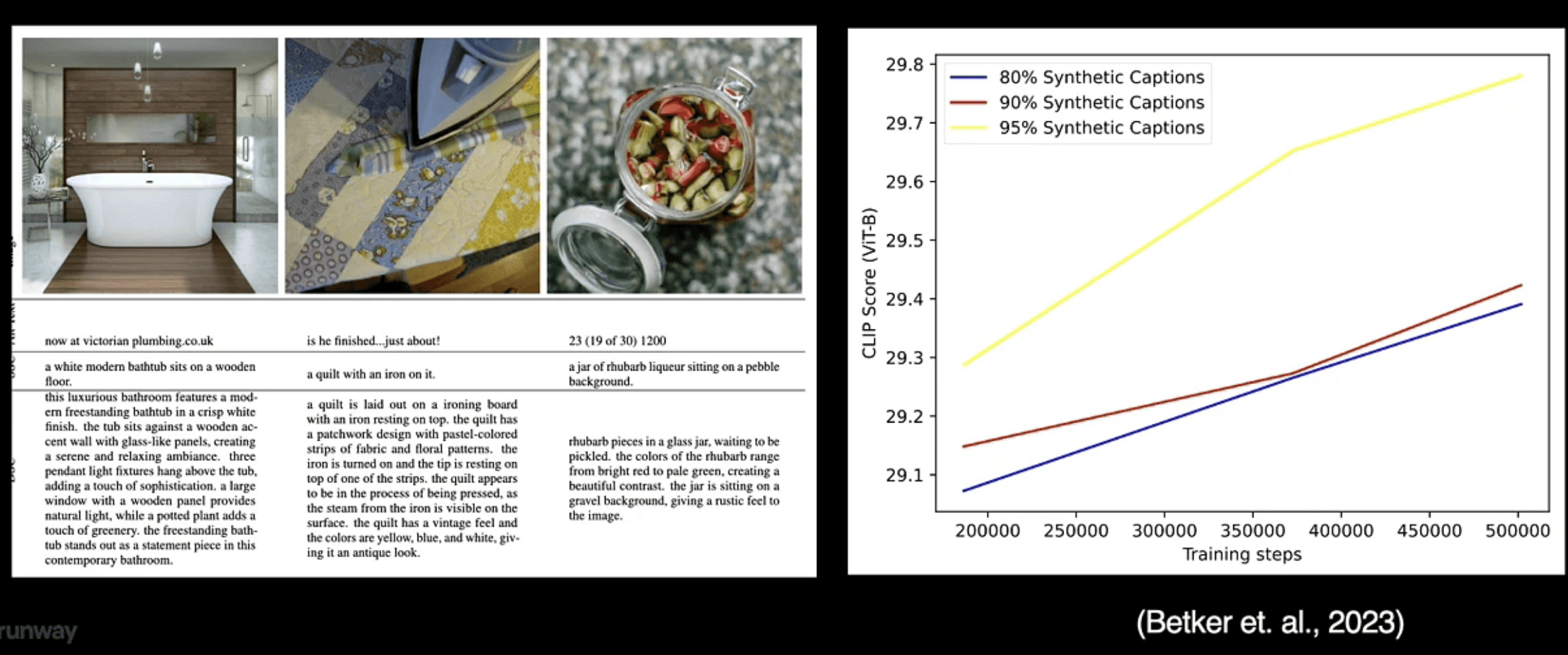
The presentation highlighted the critical role of data quality in model performance. Lee demonstrated how high-quality captions significantly improve both image and video models' capabilities. However, he noted that obtaining high-quality, large-scale video captions remains a significant challenge in the field, requiring innovative approaches to data collection and curation.
3. Scalable Neural Architecture
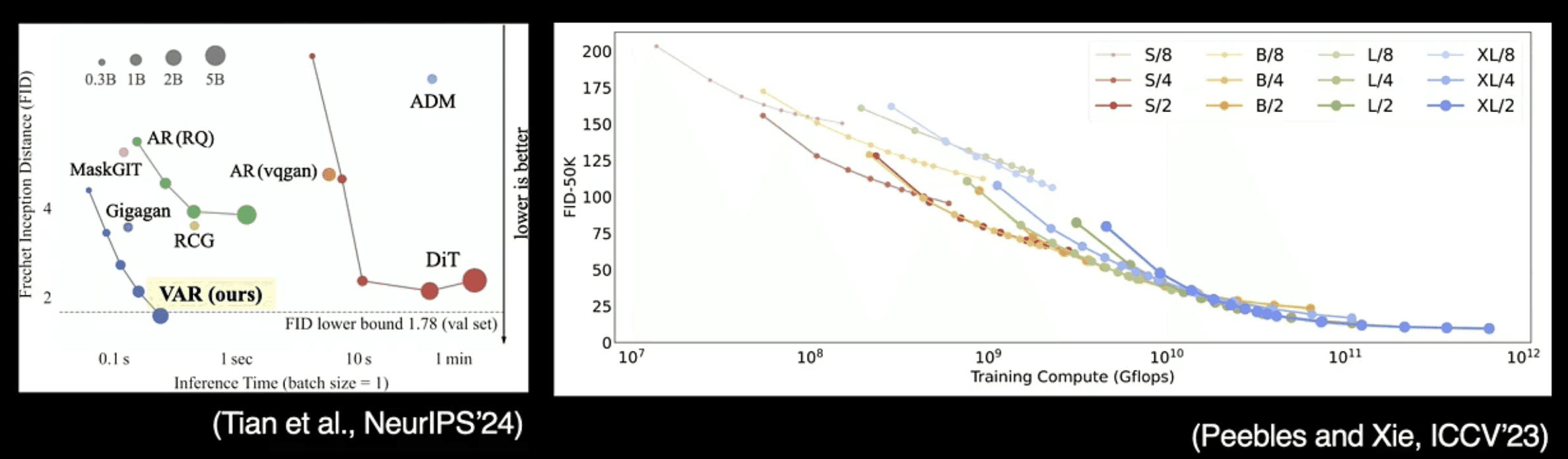
Lee detailed the importance of developing architectures that avoid relational inductive bias between data entities. The presentation included technical discussions of neural scaling laws, which provide an empirical framework for economic training with large compute resources. This approach enables more efficient utilization of computational resources while maintaining model performance.
4. Large-scale Compute Optimization
A critical technical component involves maximizing model FLOPS units (MFUs) during large-scale distributed training. Lee explained that MFU, defined as the ratio of observed throughput to theoretical maximum throughput, serves as a key metric for optimization. The approach encompasses various technical optimizations:
Implementation of low-precision computing techniques
Development of custom CUDA kernels for improved performance
Advanced parallelism strategies
Sophisticated cluster management systems
5. Cross-functional Team Integration
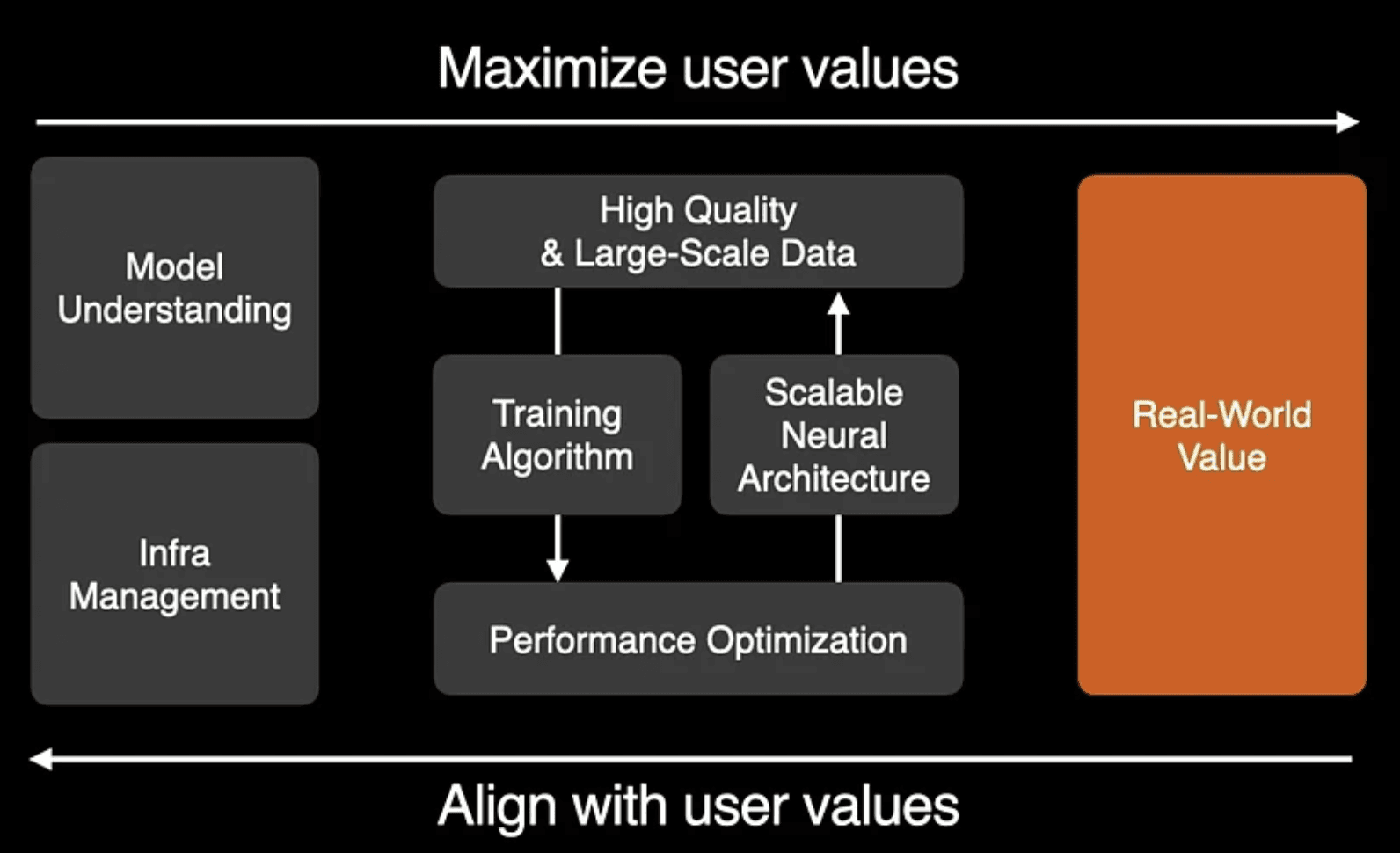
Lee emphasized that training large-scale models requires a systematic approach across functional teams. He presented a comprehensive framework for team collaboration, highlighting the importance of continuous feedback loops and cross-disciplinary expertise.
Runway's Advanced Video Generation Models
Gen-3 Alpha
Lee provided an in-depth look at Runway's Gen-3 Alpha model, which was released in June 2024. The model demonstrates remarkable capabilities in generating high-quality and diverse videos from natural language prompts. However, he acknowledged the ongoing challenge of fully understanding the model's ability to combine and generalize its world knowledge.
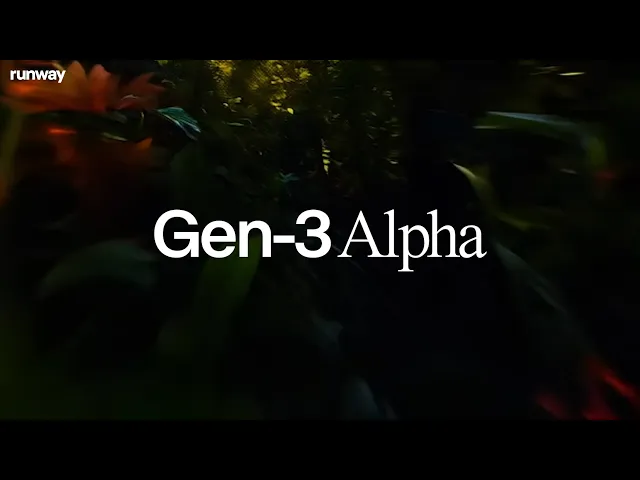
The Runway research team has conducted extensive research to understand the model's capabilities in several key areas:
Understanding and application of physical laws
Text rendering capabilities
Combinatorial generalization across visual concepts
Intuitive Control Mechanisms
Lee introduced several innovative approaches to controlling video generation:
Keyframe conditioning for precise stylistic control
Seamless integration with existing workflows through Generative VFX
Advanced camera control systems with 3D understanding
Fine-grained motion control through specialized brushes
Structural conditioning for video composition
Video out-painting capabilities for expanded creative control
Act-One: The Next Generation
The presentation included a demonstration of Act-One, representing the next evolution in video generation technology. This system builds upon the foundations of Gen-3 Alpha while incorporating more sophisticated control mechanisms and improved understanding of temporal dynamics.
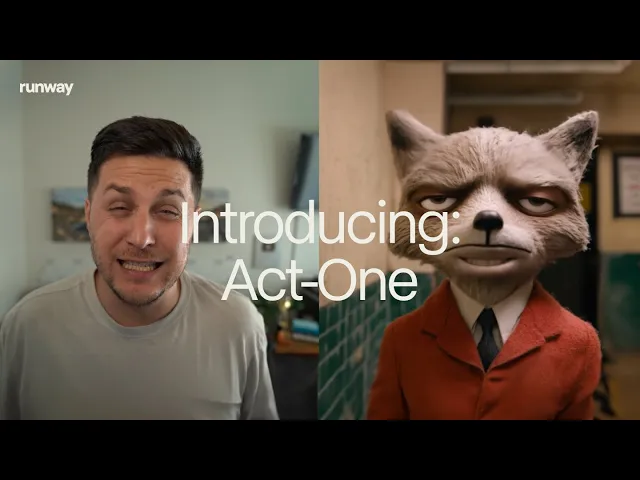
Future Directions and Challenges
Lee concluded by emphasizing that language and video serve as fundamental world descriptors: language providing the most abstract knowledge representation, and video offering the most scalable source of visual world representation. He stressed that scaling remains a crucial factor, with access to gigantic video datasets and the entire visual world for interaction.
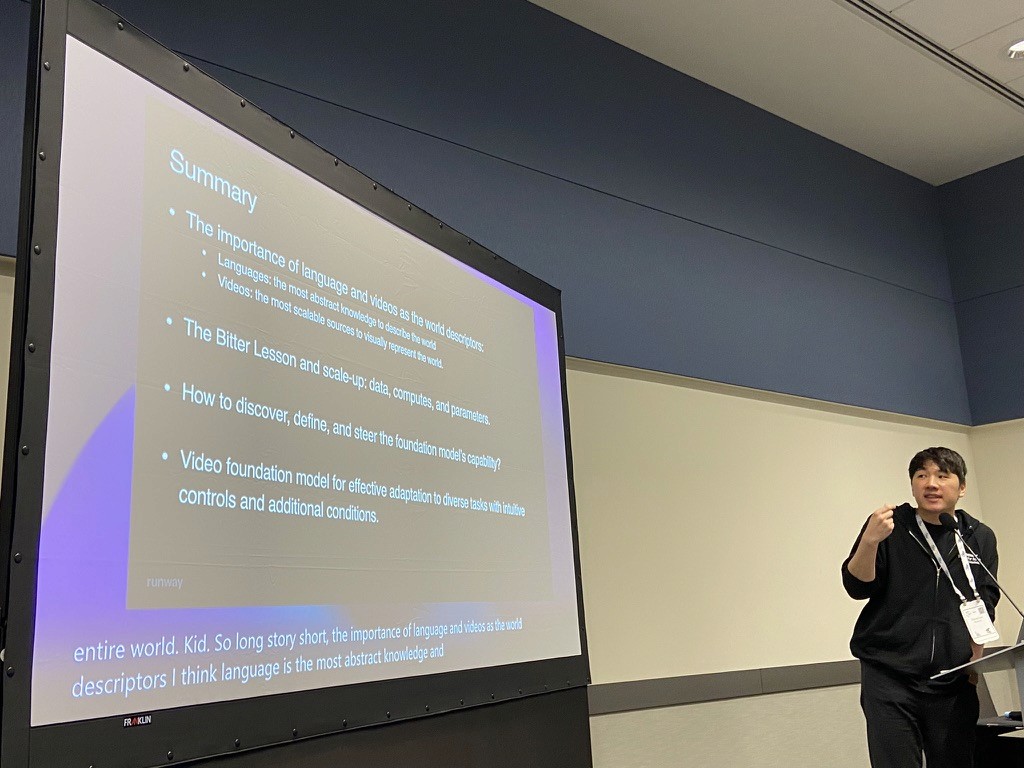
The presentation ended with a clear vision: the development of General World Models that understand the world at an unprecedented level. This goal requires continued innovation in:
Model architecture and scaling
Data collection and curation
Computational efficiency
Intuitive control mechanisms
Integration with existing creative workflows
The Future of Video-Language Models: A Vision Forward
The inaugural NeurIPS 2024 Video-Language Model Workshop has illuminated both the remarkable progress and compelling challenges in the field of video understanding. As an industry practitioner and organizer of this workshop, Twelve Labs recognizes the critical themes that emerged from our distinguished speakers' presentations.
Key Insights and Emerging Themes
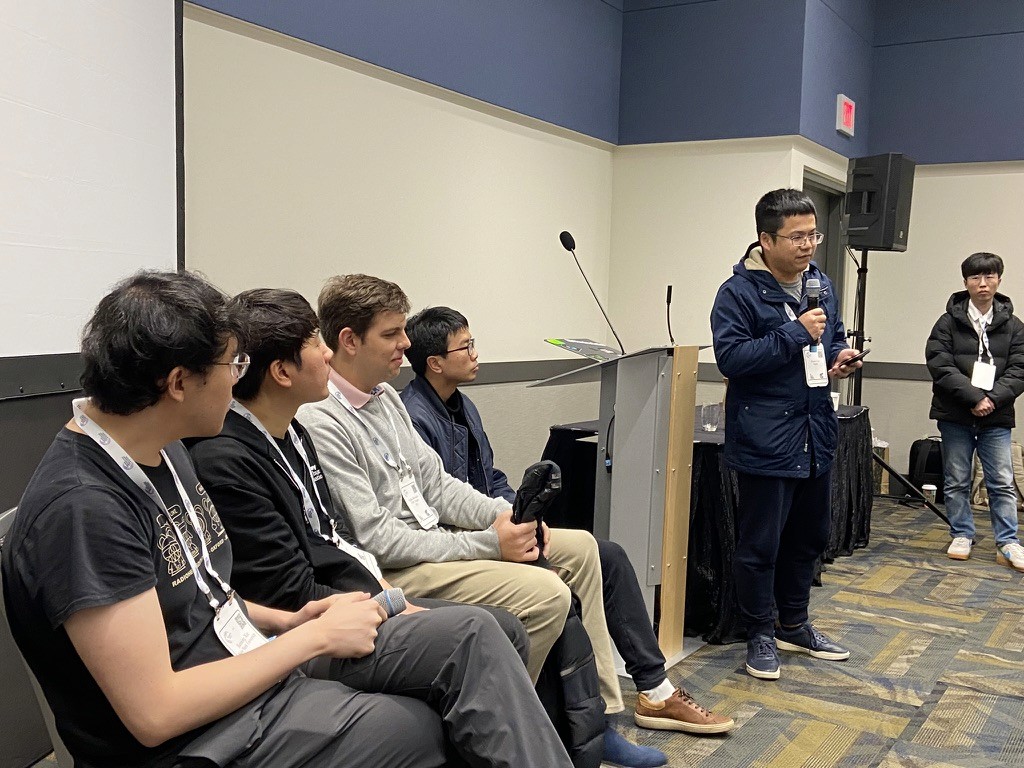
Several crucial themes resonated throughout the workshop. Professor Dima Damen highlighted the importance of understanding fine-grained temporal dynamics in egocentric videos, while Professor Gedas Bertasius emphasized the need to move beyond pure language-centric approaches in video understanding. Professor Yong Jae Lee's presentation revealed that even short-form video understanding remains challenging, particularly when dealing with counterfactual temporal information.
Jianwei Yang's vision of multimodal agentic models and Ishan Misra's insights into video generation demonstrated how the field is evolving beyond simple understanding tasks toward more sophisticated applications. Doyup Lee's exploration of general world models highlighted the potential for video-language models to develop comprehensive world understanding.
Industry Perspective and Path Forward
At Twelve Labs, these challenges resonate deeply with our experience developing video understanding technologies. Our journey with Marengo, our video embedding model, and Pegasus, our video-to-text model, has taught us firsthand about the complexities of multimodal integration, temporal understanding, and the critical importance of high-quality training data.
The workshop discussions validate our approach to tackling these challenges through:
Multi-vector embeddings that capture the multifaceted nature of video content
Sophisticated temporal modeling that addresses both short-term and long-term dependencies
Integration of multiple modalities including visual, audio, and textual information
Development of robust evaluation frameworks that go beyond traditional metrics
Vision for the Future
The future of video-language models is incredibly promising. As we move forward, we envision:
More sophisticated temporal understanding capabilities that can handle complex narratives and counterfactual reasoning
Better integration of world knowledge with visual understanding
More efficient architectures that can process longer videos while maintaining temporal coherence
Stronger bridges between academic research and industrial applications
This workshop marks an important milestone in bringing together researchers and practitioners to address these challenges collectively. As we continue to push the boundaries of what's possible in video understanding, we remain committed to fostering open collaboration and responsible development in this rapidly evolving field.
The path ahead is both challenging and exciting. By continuing to build on the insights shared at this workshop and maintaining strong collaboration between academia and industry, we can accelerate the development of more capable, efficient, and reliable video-language models that will transform how we perceive and interact with the world.
The inaugural NeurIPS 2024 Video-Language Model Workshop marked a pivotal moment in the rapidly evolving field of video understanding and generation. In his opening remarks, our CTO Aiden Lee highlighted the growing interest in video-language models across two key areas: video understanding (video-to-text) and video generation (text-to-video).
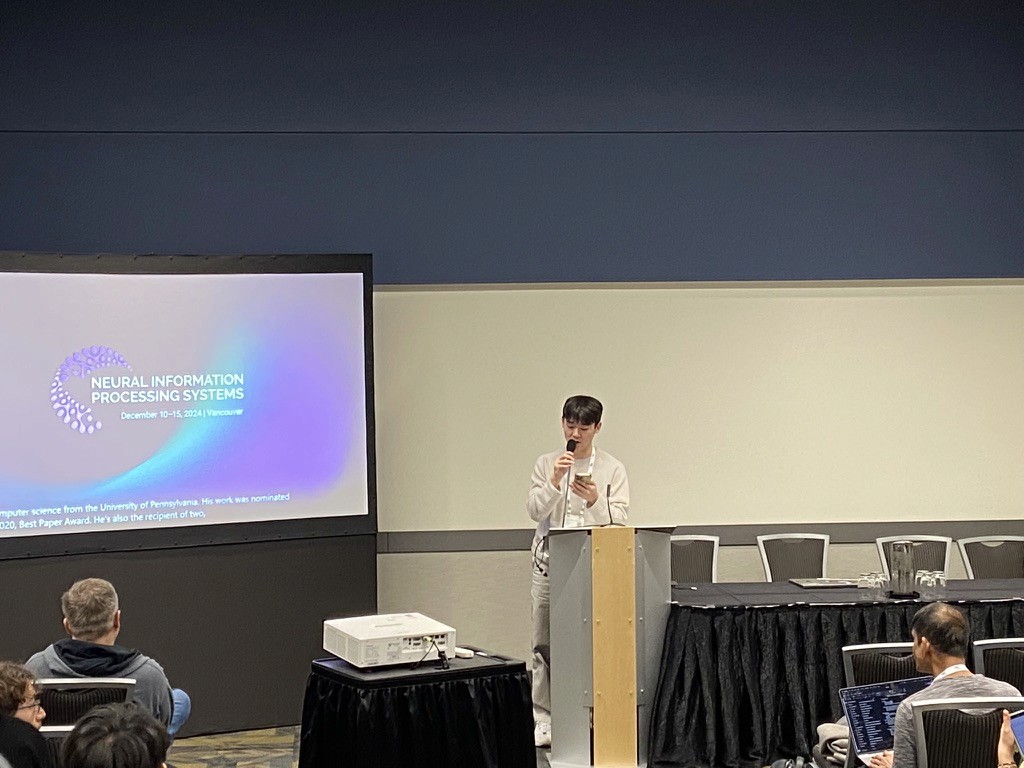
The applications of these technologies span diverse sectors, including content creation, advertising, healthcare, and robotics. Despite the exponential growth in vision-language model research, the field faces several critical challenges:
Data scarcity, particularly in high-quality video and text pairs
Scale and complexity issues in video storage and processing
Challenges in multimodal integration across visual, audio, and text components
Difficulties in benchmarking and evaluating video-language alignment
The workshop aimed to address these challenges by bringing together researchers to discuss current obstacles, foster open collaboration, and accelerate the development of video foundation models for real-world applications.
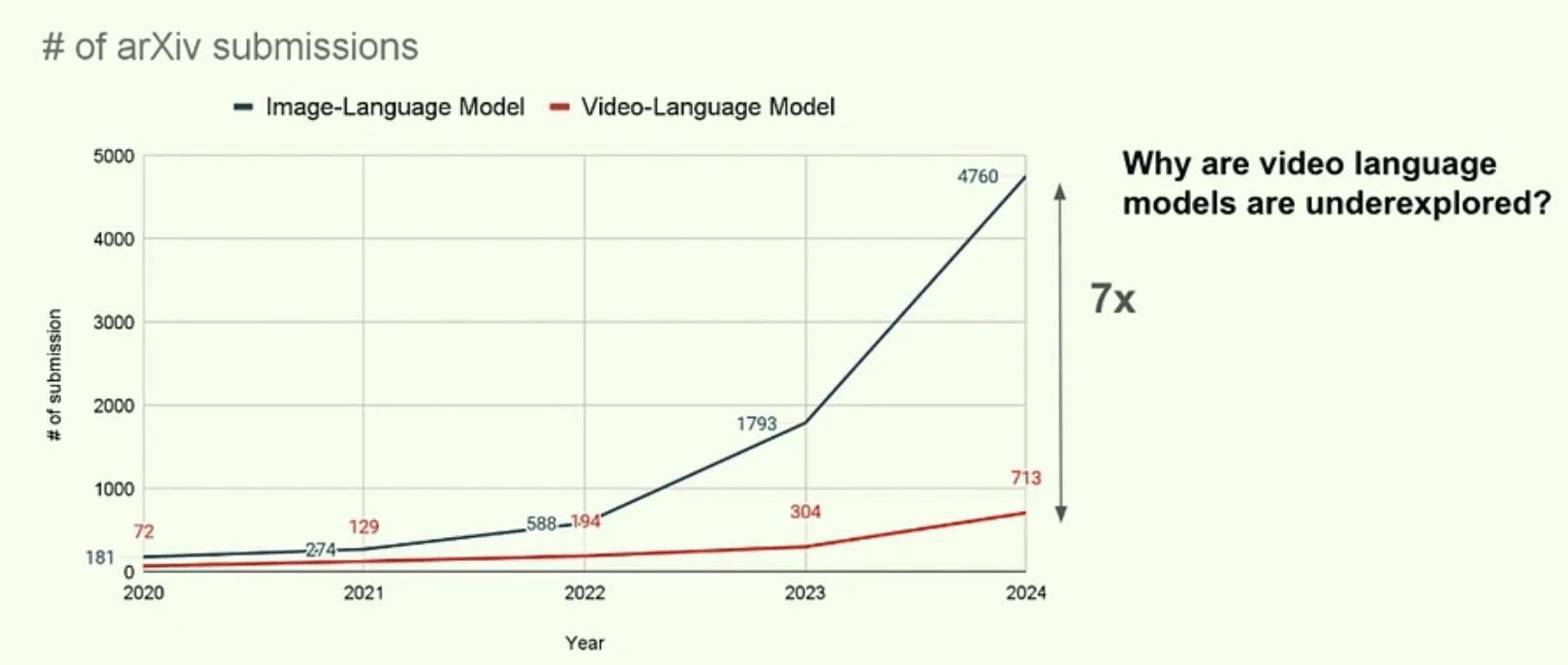
1 - Egocentric Video and Language Understanding - Dima Damen
Professor Dima Damen's comprehensive talk explored four cutting-edge aspects of egocentric video and language understanding. Her research draws from the EPIC-KITCHENS dataset and introduces several groundbreaking approaches.
Unique Captioning for Similar Actions
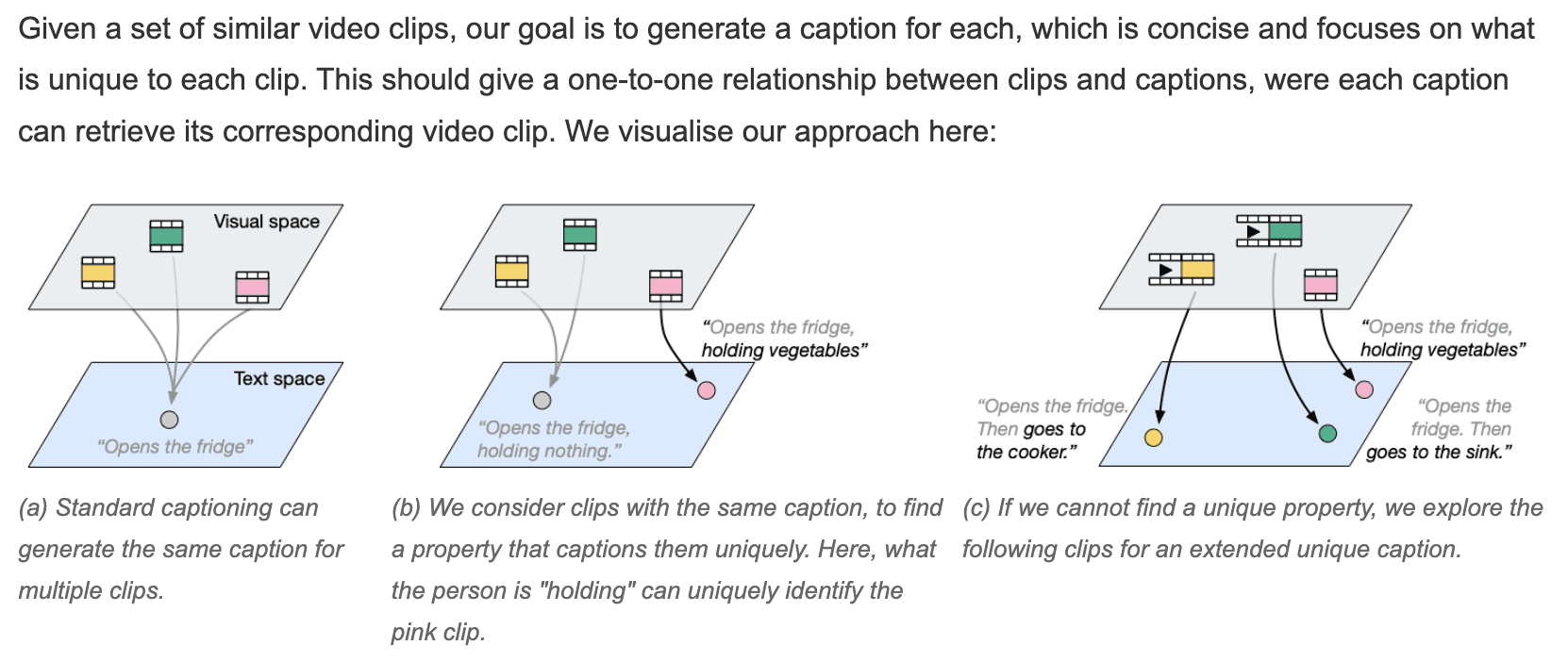
The paper "It's Just Another Day," which won the Best Paper Award at ACCV 2024, addresses a fundamental challenge in video captioning: the tendency of current methods to generate identical captions for similar clips when processing them independently.
The key insight behind this work is that while life is repetitive, we need distinct descriptions for similar actions. The researchers propose a novel approach called Captioning by Discriminative Prompting (CDP) that:
Considers clips jointly rather than in isolation
Utilizes a bank of discriminative prompts to generate unique captions
Evaluates through both video-to-text and text-to-video retrieval metrics
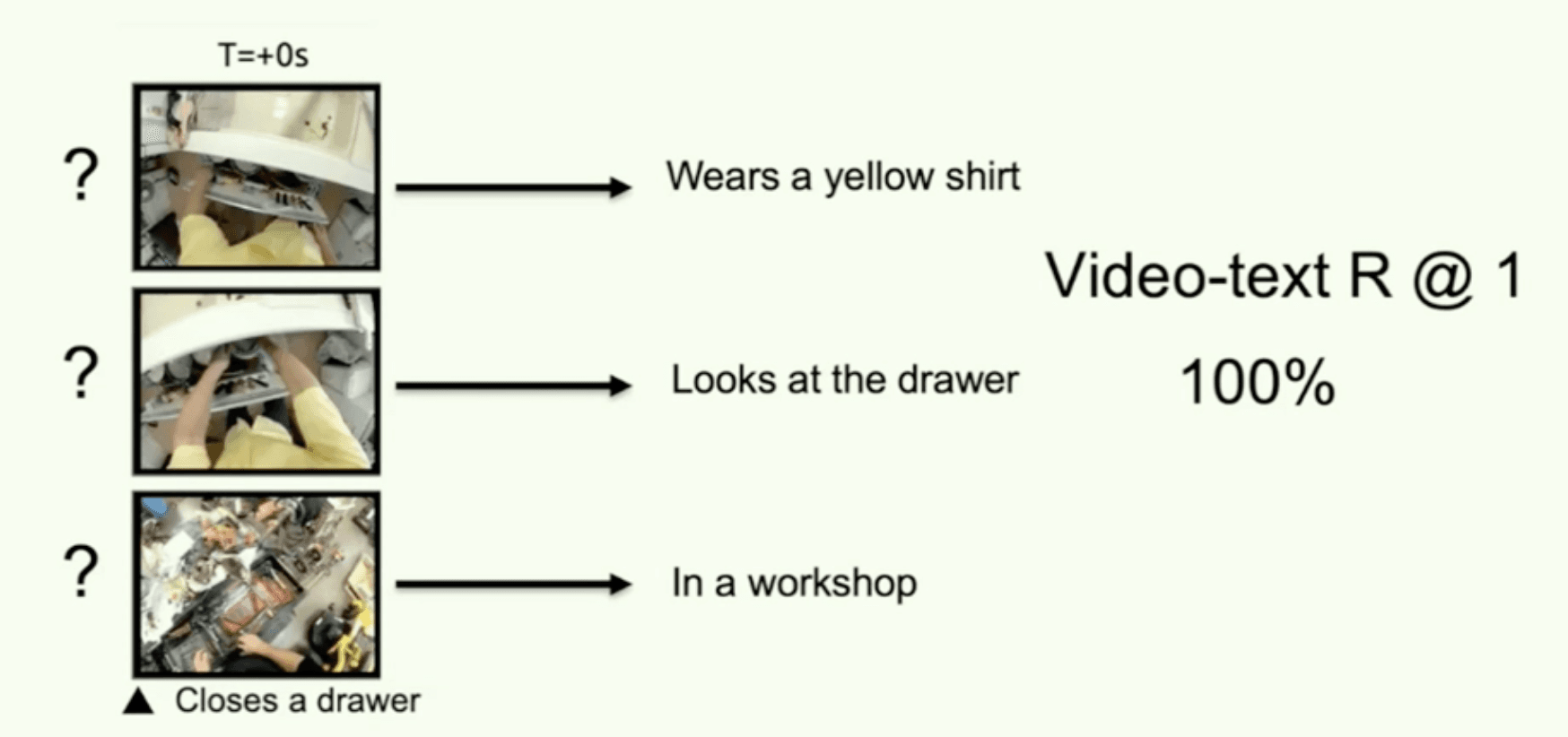
The method was evaluated on two domains: egocentric videos from Ego4D and timeloop movies. When applied to the LaViLa VCLM captioner, CDP showed significant improvements in caption uniqueness while maintaining semantic accuracy.
Domain Generalization Using Language
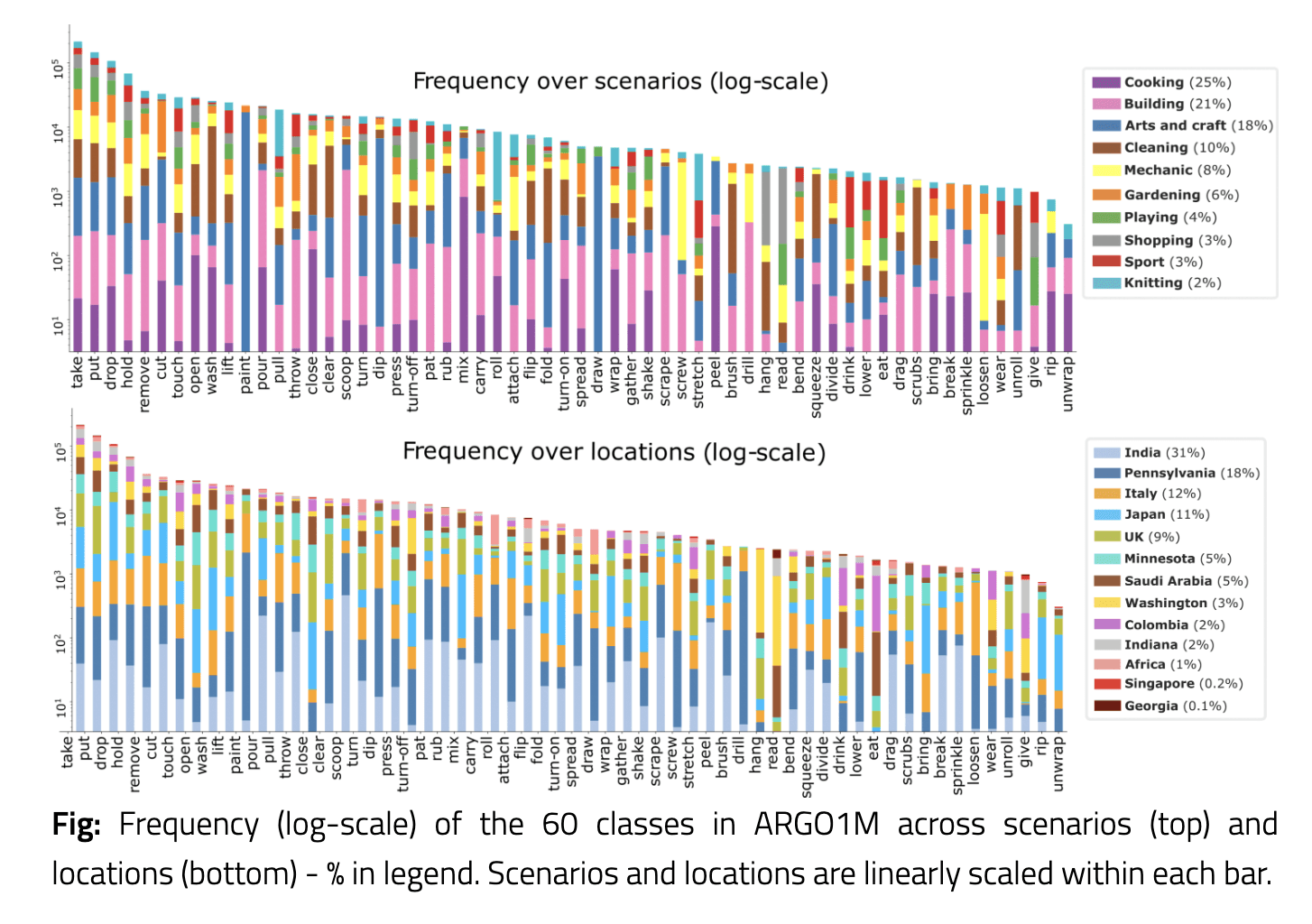
A major contribution is the ARGO1M dataset, the first of its kind designed specifically for Action Recognition Generalization across scenarios and locations. The dataset features:
1.05M action clips spanning 60 action classes
Recordings from 13 different locations
10-second clip duration standard
The accompanying paper "What can a cook in Italy teach a mechanic in India?" introduces Cross-Instance Reconstruction (CIR), which employs two key reconstructions:
Video-text association reconstruction using text narrations
Classification reconstruction trained for action class recognition
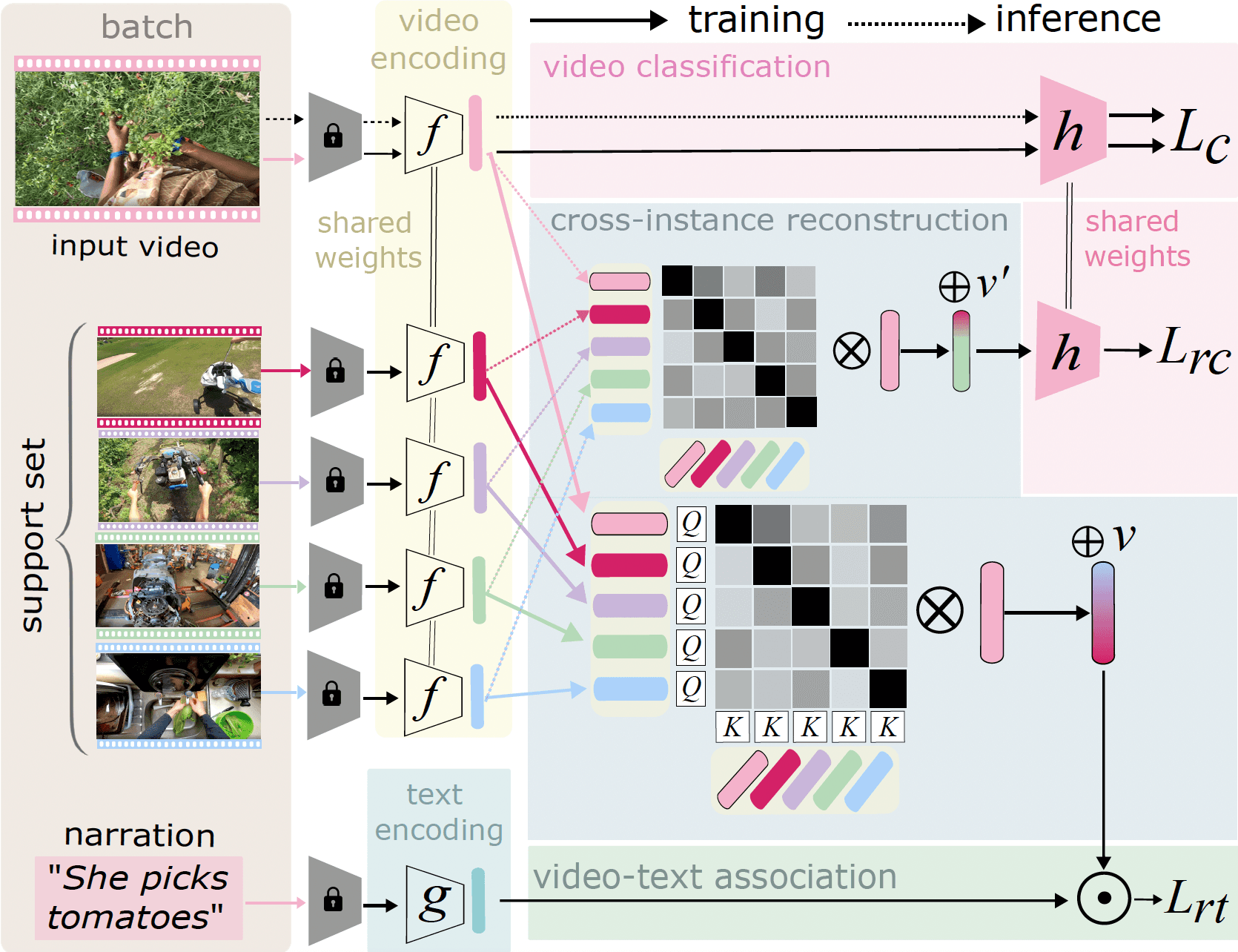
CIR represents actions as weighted combinations of actions from different scenarios and locations, enabling robust cross-domain generalization.
Hand-Object Interaction Referral
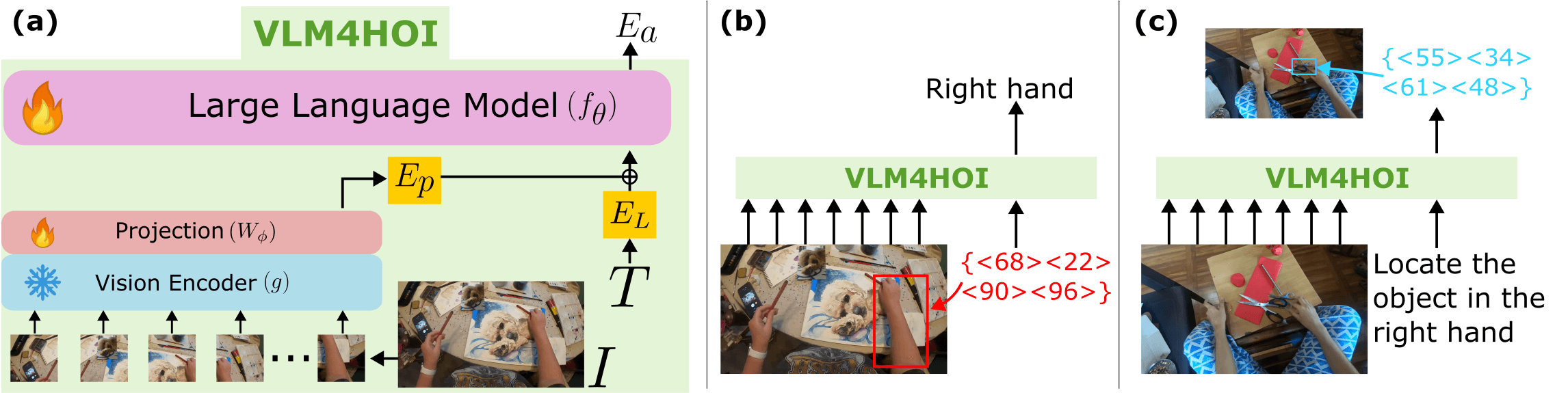
The HOI-Ref project introduces VLM4HOI, a sophisticated model for hand-object interaction referral in egocentric images. The model architecture includes:
A vision encoder (g) and projection layer (Wφ) for image processing
Language model embedding space integration
Capability to identify both hands and interacted objects
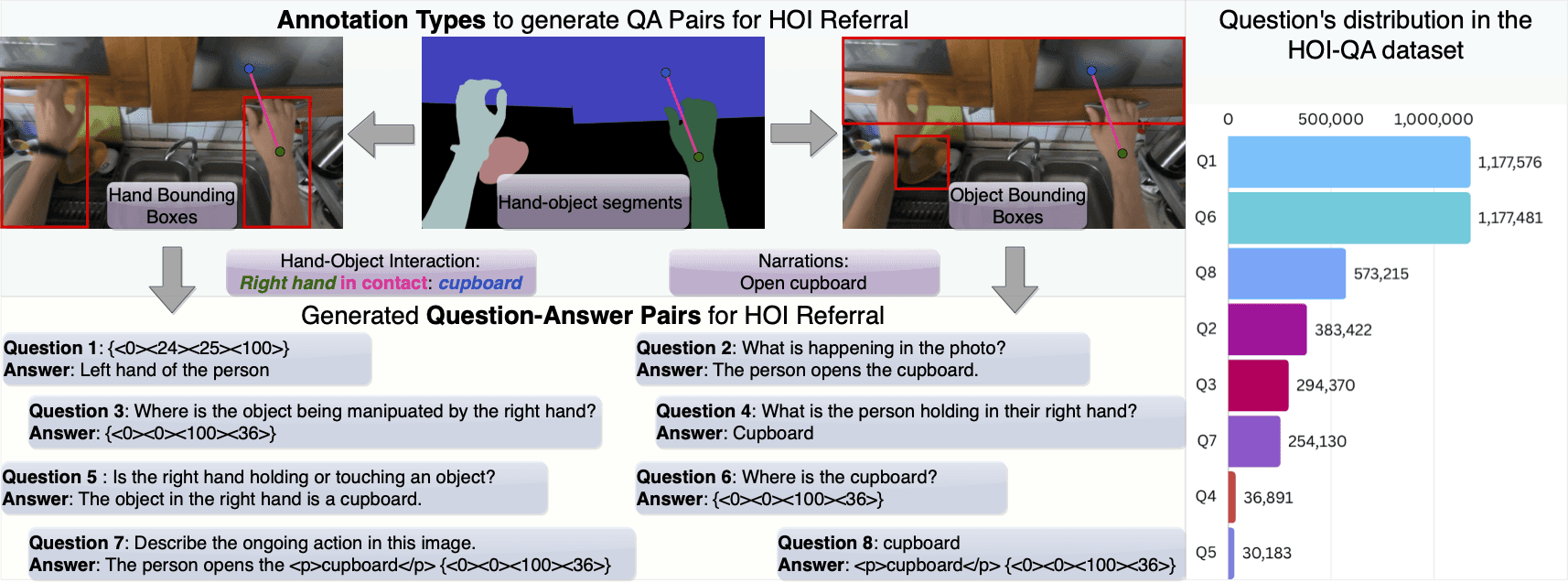
The researchers developed HOI-QA, a comprehensive dataset containing 3.9M question-answer pairs, focusing on:
Spatial referral and recognition of hands and objects
Understanding of hand-object interactions
Direct referral versus interaction referral capabilities
Audio-Visual Semantic Integration
This section presented two significant contributions to the field:
EPIC-SOUNDS Dataset
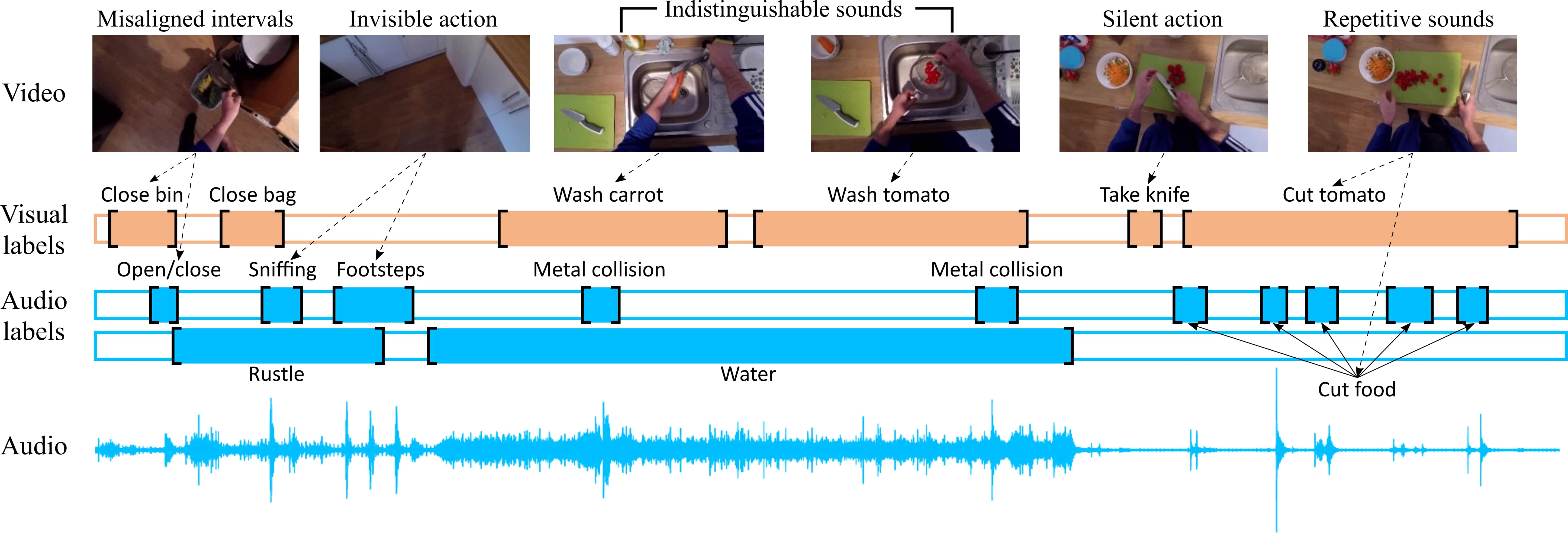
This large-scale dataset captures audio annotations within egocentric videos from EPIC-KITCHENS-100, featuring:
75.9k segments of audible events and actions
44 distinct action classes
Detailed material annotations for object collision sounds
Temporal labeling of distinguishable audio segments
Time Interval Machine (TIM)
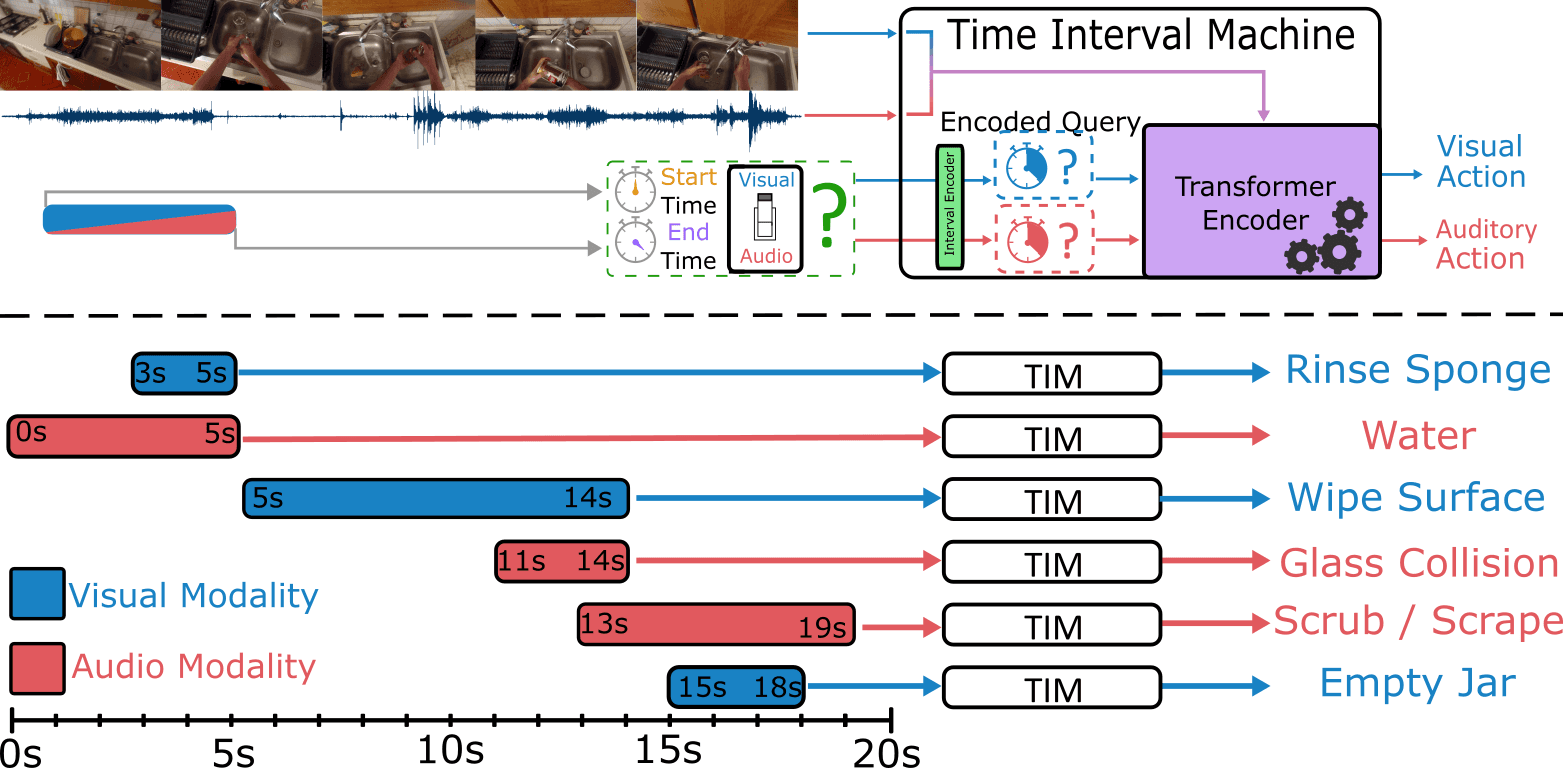
TIM represents a breakthrough in handling the different temporal extents of audio and visual events in long videos. Key features include:
A transformer encoder that processes modality-specific time intervals
Attention mechanisms for both interval-specific and surrounding context
State-of-the-art performance on multiple benchmarks
The model achieved remarkable results:
2.9% improvement in top-1 action recognition accuracy on EPIC-KITCHENS
Superior performance on Perception Test and AVE datasets
Successful adaptation for action detection using dense multi-scale interval queries
These advances collectively demonstrate significant progress in understanding and processing egocentric video content, particularly in handling the complex interplay between visual, textual, and audio modalities. The research from Dima’s group presents a comprehensive framework for addressing the challenges of multimodal integration in egocentric video understanding.
2 - Complex Video Understanding using Language and Video - Gedas Bertasius
Professor Gedas Bertasius's talk highlighted both the remarkable progress and current limitations of video-language models (VLMs) in complex video understanding tasks. While acknowledging the significant advances in video understanding due to LLMs and VLMs, he emphasized that modern VLMs still face fundamental challenges with complex video understanding tasks.
Addressing Long-Range Video Understanding
A key technical challenge identified is the struggle of existing models to localize relevant content in long video sequences. Bertasius introduced Structured State-Space Models (S4) as an efficient approach to model long-range dependencies in video (as seen in this work and this work). However, he noted that S4 models struggle with tasks requiring selective attention to specific parts of the input sequence, as their parameters remain constant for every input token.
BIMBA: Selective-Scan Compression
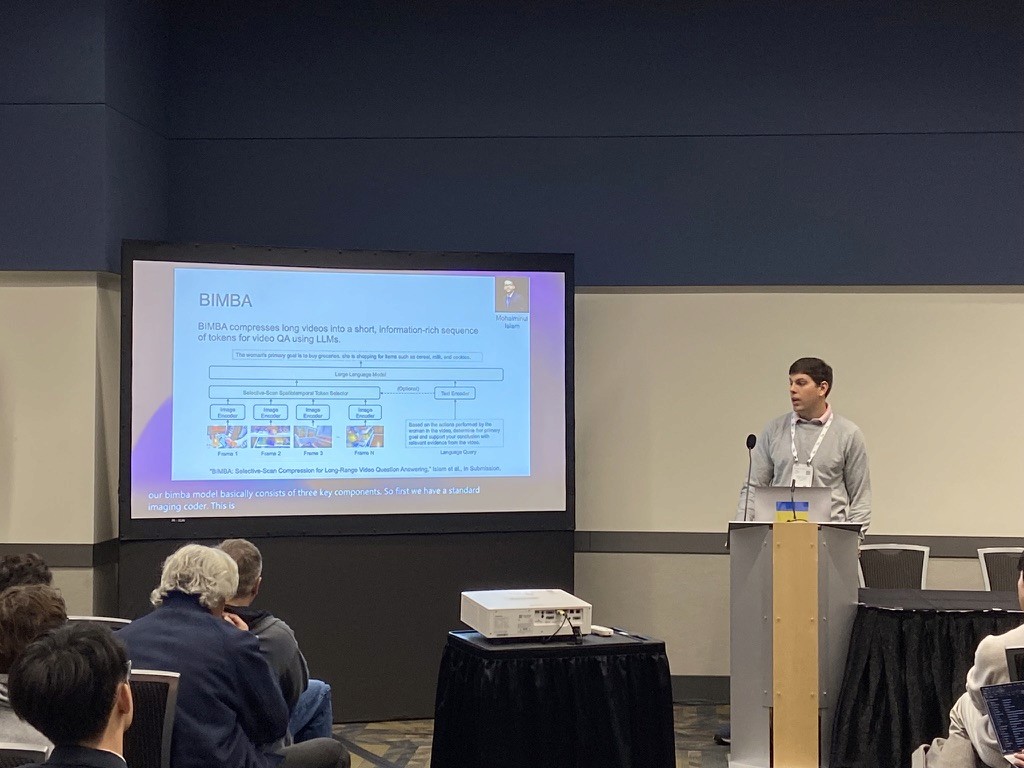
BIMBA addresses these limitations by introducing the selective scan algorithm (also known as Mamba) to choose which parts of the input should be stored in the hidden state in a data-dependent manner. The model features:
A spatiotemporal token selector using bidirectional selective-scan mechanism
Interleaved visual queries for precise content selection
Input-dependent parameters for adaptive processing
Efficient compression of long videos into information-rich token sequences
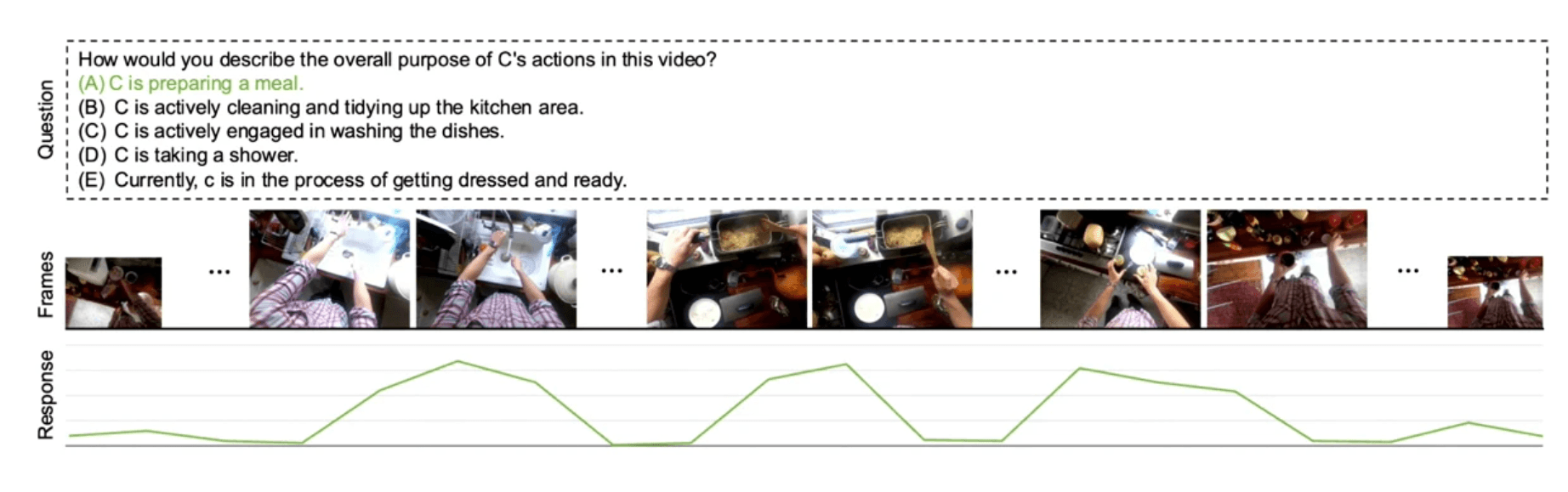
In evaluation on the EgoSchema benchmark, BIMBA demonstrated superior performance in identifying the most relevant parts of videos for question answering tasks, with qualitative results showing precise selection of video segments relevant to given queries.
LLoVi: LLM Framework for Long VideoQA
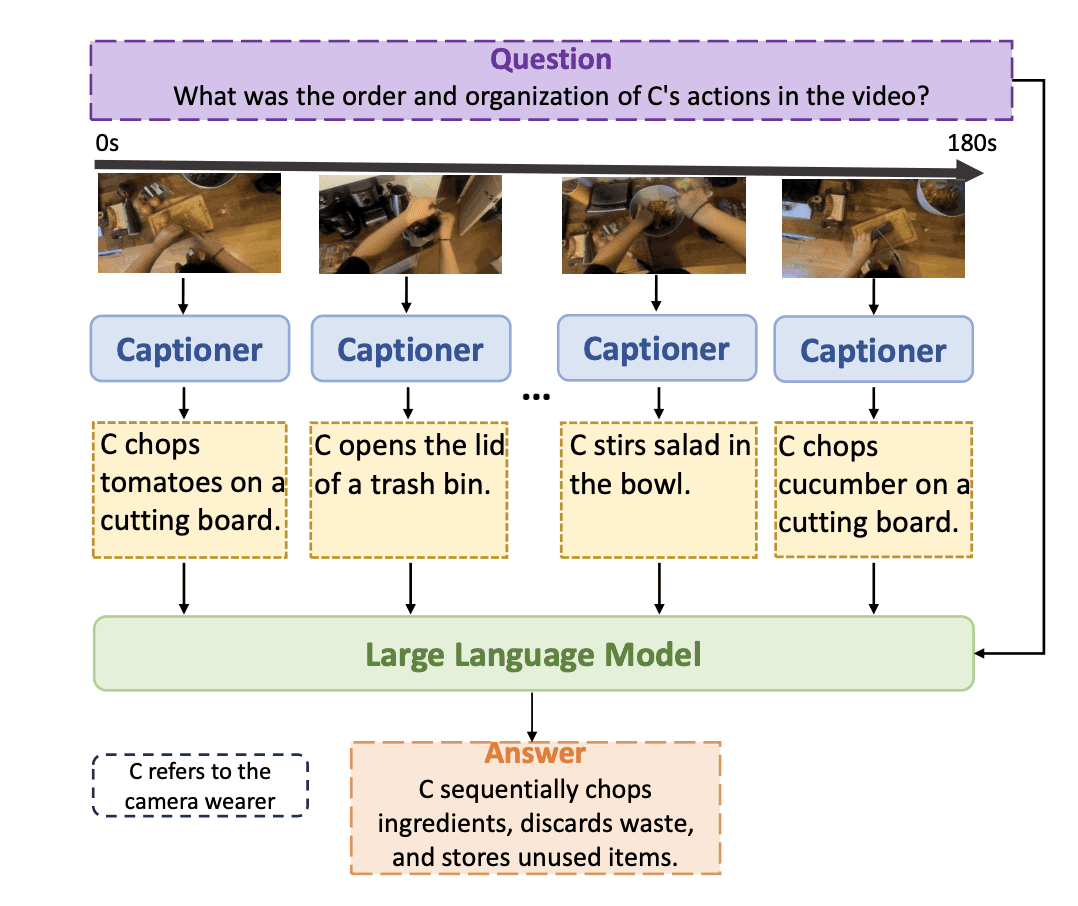
LLoVi introduces a more straightforward but highly effective approach to long-range video question answering through a two-stage process:
Stage 1: Video Processing
Samples short clips from long videos
Extracts captions for each clip
Maintains temporal ordering of information
Stage 2: Question Answering
Feeds temporally ordered captions into an LLM
Employs a novel two-round summarization prompt
Enhances complex question-answering capabilities
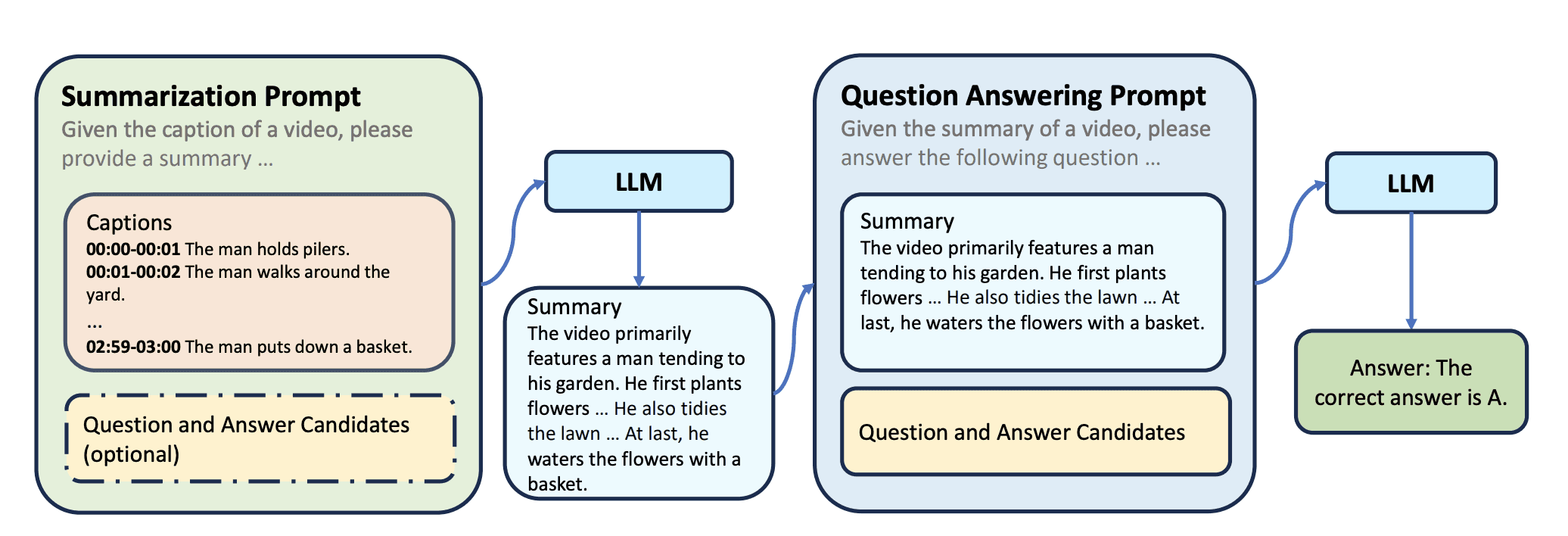
The evaluation results on EgoSchema demonstrated LLoVi's effectiveness in handling long-form video content, though Bertasius noted that the model uses 1750 times more parameters to model language than video, raising questions about efficiency and information preservation.
Beyond Language-Centric Approaches
Bertasius emphasized the limitations of representing videos purely through language, noting that captions and summaries dramatically reduce the rich information present in original video content.
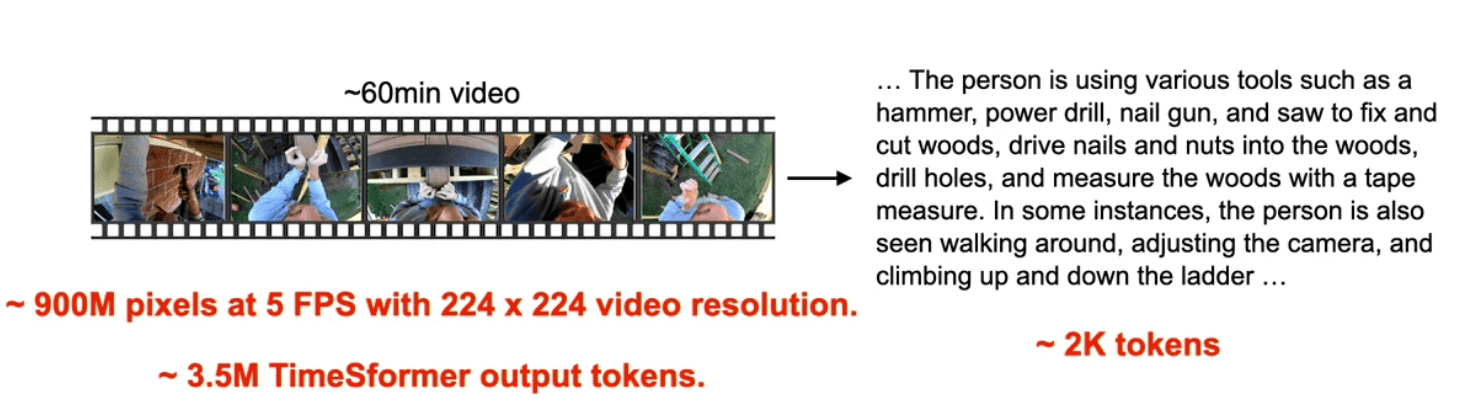
BASKET: Fine-Grained Skill Estimation Dataset
To address these limitations, the talk introduced BASKET, a comprehensive dataset for fine-grained skill estimation that focuses on visual understanding rather than language description. The dataset includes:
4,400+ hours of video content
32,232 players from 30+ countries across 4 continents
20 fine-grained basketball skills
8-10 minute highlight videos per player
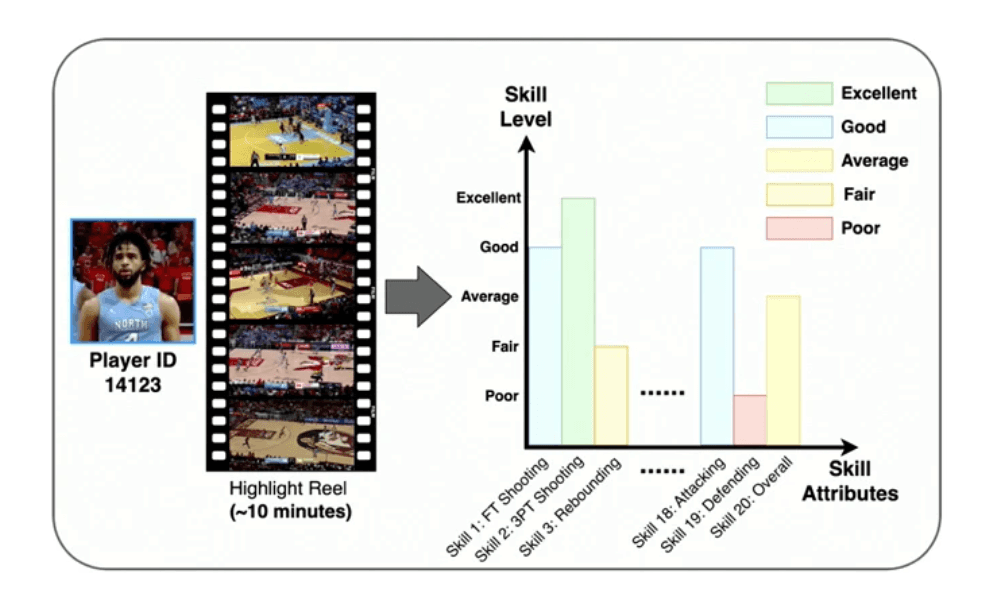
Basketball was selected as the focus sport due to several key advantages:
Global reach with 400M+ fans
High participant diversity
Abundant video footage availability
Complex fine-grained skills requiring temporal modeling
Similar player appearances necessitating fine-grained visual recognition
Performance Results:
Current state-of-the-art models struggle with fine-grained skill estimation
No model achieved more than 30% accuracy on the benchmark
Results highlight the significant gap between human and AI performance in complex video understanding tasks
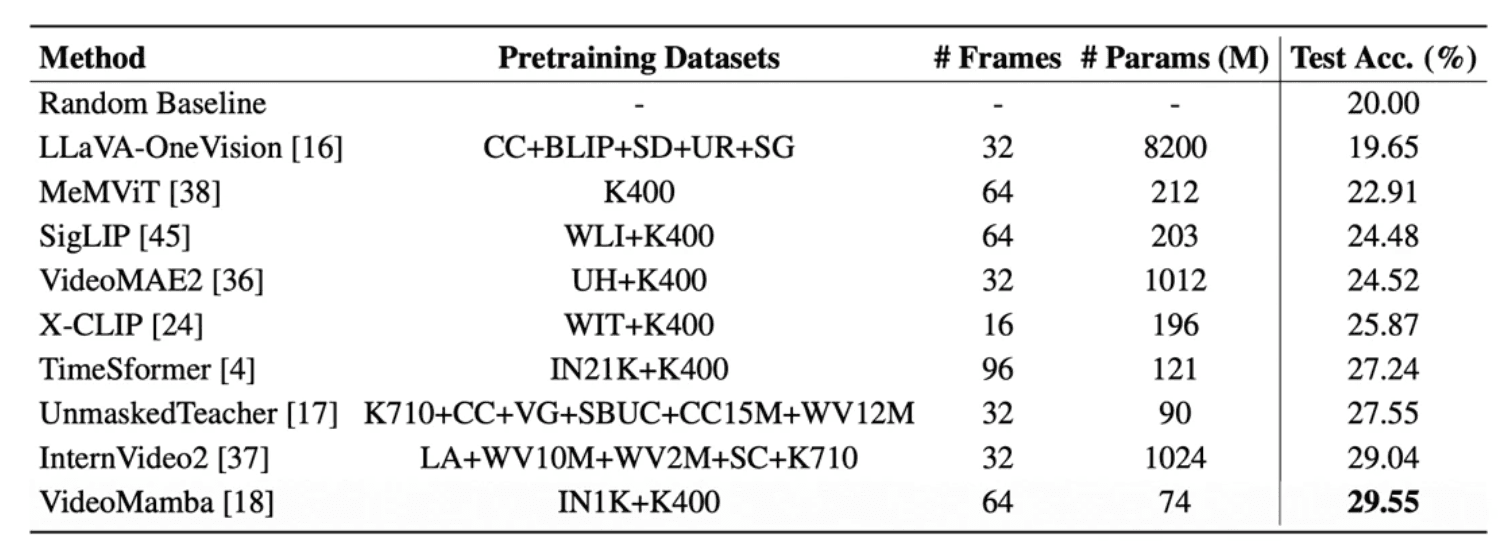
Key Takeaways and Future Directions
While LLMs have enabled remarkable progress in video understanding tasks, problems that are difficult to formulate using language don't benefit as much from language models or large-scale web video-text pre-training
The strong performance of LLMs and flaws in existing video-language benchmarks may mask underlying issues with video modeling
Current evaluation metrics may not fully capture the complexity of video understanding tasks
The field needs to develop more sophisticated approaches that can handle both language-based and purely visual understanding tasks
3 - How Intelligent Are Current Multimodal Video Models? - Yong Jae Lee
Professor Yong Jae Lee's talk challenged the conventional wisdom about the capabilities of current multimodal video models. While much of the research community has been focusing on understanding long-form videos, Lee emphasized a crucial point: understanding even short videos (less than 10 seconds) with counterfactual temporal information remains a significant challenge. This observation led to the development of two major contributions: Vinoground and Matryoshka Multimodal Models.
Vinoground: A New Benchmark for Temporal Reasoning
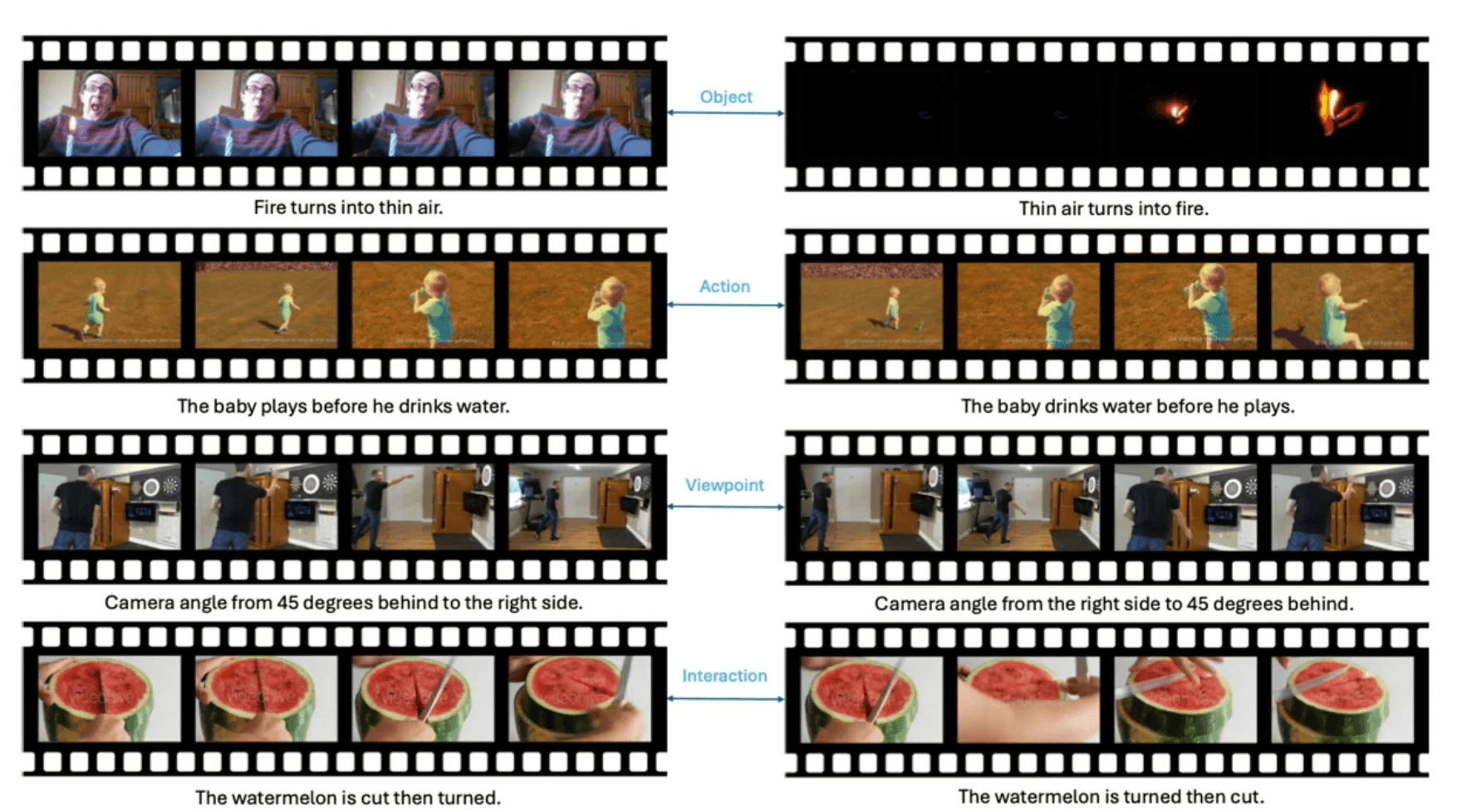
Inspired by Winoground, a challenging counterfactual benchmark for visio-linguistic compositional reasoning in images, Vinoground extends this concept to the video domain. The benchmark's name cleverly changes the 'W' to a 'V' for "video" while maintaining the focus on temporal counterfactuals as a unique element in video data.
The benchmark was designed with several crucial requirements to ensure robust evaluation of video understanding capabilities:
Videos must not contain single frame bias that could allow models to "cheat" by looking at individual frames
Tasks cannot be solved through textual cues alone, requiring true visual understanding
All videos must be natural, avoiding synthetic or artificially constructed scenarios
Complete video comprehension is necessary, with particular emphasis on event ordering
To make the benchmark especially challenging, each video pair comes with corresponding captions that use identical words but in different orders. For example, "a man waves at a woman before he talks to her" versus "a man talks to a woman before he waves at her." This design ensures that models must truly understand the temporal sequence of events rather than relying on simple pattern matching.
Technical Implementation
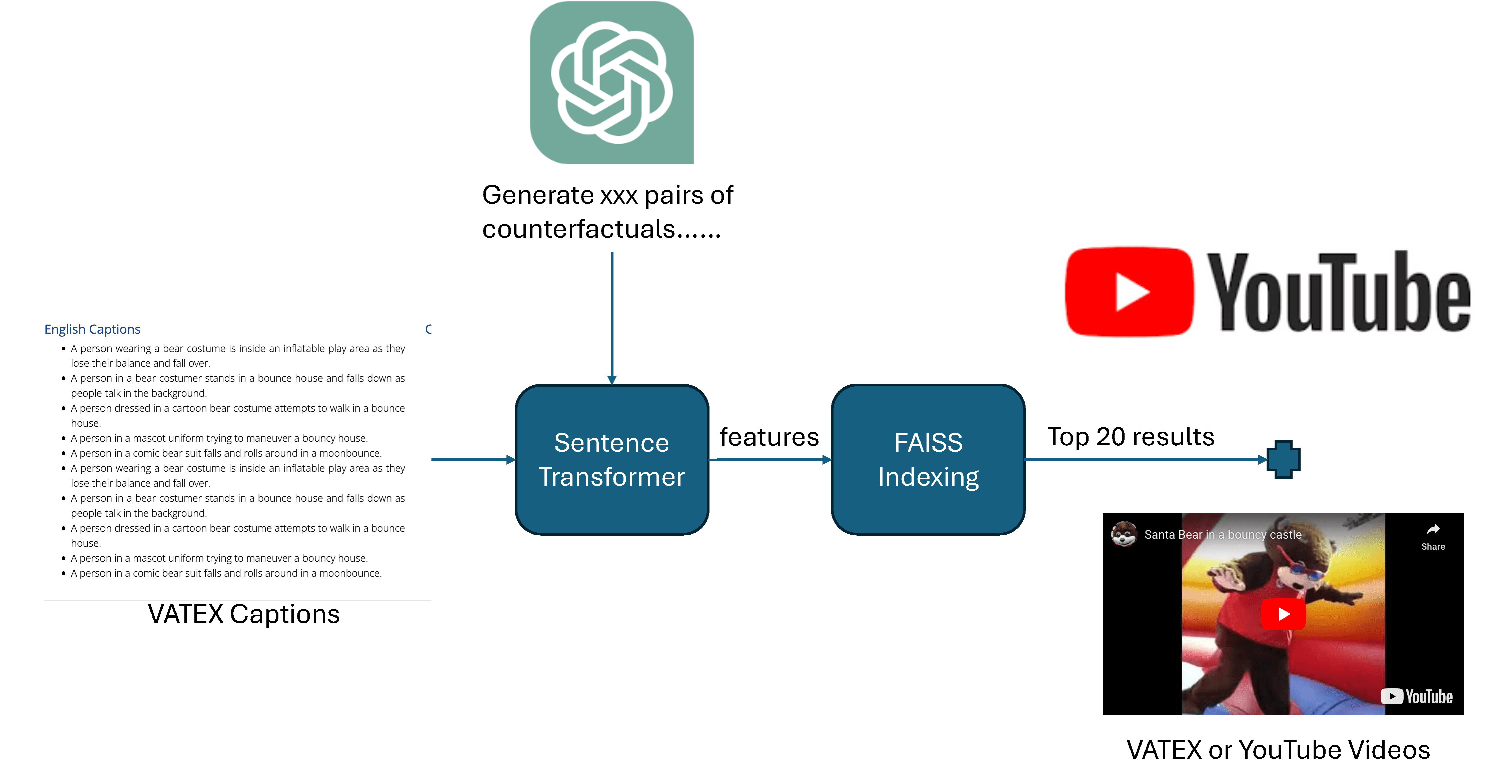
The data curation process involves a sophisticated pipeline where GPT-4 first generates counterfactual caption pair candidates. These candidates are then matched with appropriate videos using VATEX captions as an index, leveraging sentence transformers and the FAISS library for efficient similarity search. When direct matches aren't found in existing datasets, the team conducts YouTube searches using the captions to find suitable videos.
The benchmark comprises 500 carefully curated video-caption pairs, featuring:
Temporal differences in actions and objects (e.g., "the man eats then watches TV" vs. "the man watches TV then eats")
Object and viewpoint transformations (e.g., "water turning into ice" vs. "ice turning into water")
Spatial transformations that test understanding of physical relationships
Comprehensive Evaluation Framework
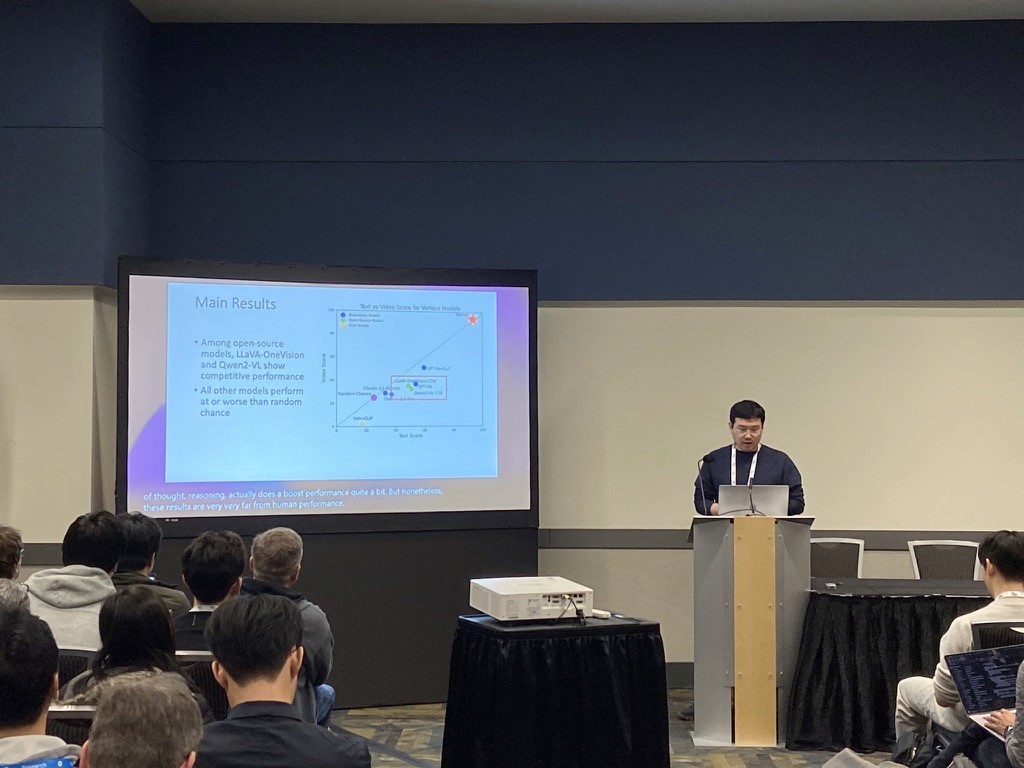
The evaluation framework employs three distinct metrics to provide a balanced assessment:
Text score: Measures the model's ability to understand linguistic differences
Video score: Evaluates visual comprehension capabilities
Group score: Assesses overall temporal understanding
Matryoshka Multimodal Models (M3)
The second major contribution addresses a fundamental bottleneck in current multimodal models: the overwhelming number of tokens, which not only makes Language-Vision Models (LMMs) inefficient but also dilutes their attention to relevant information. The Matryoshka Multimodal Models (M3) presents an elegant solution to this challenge.
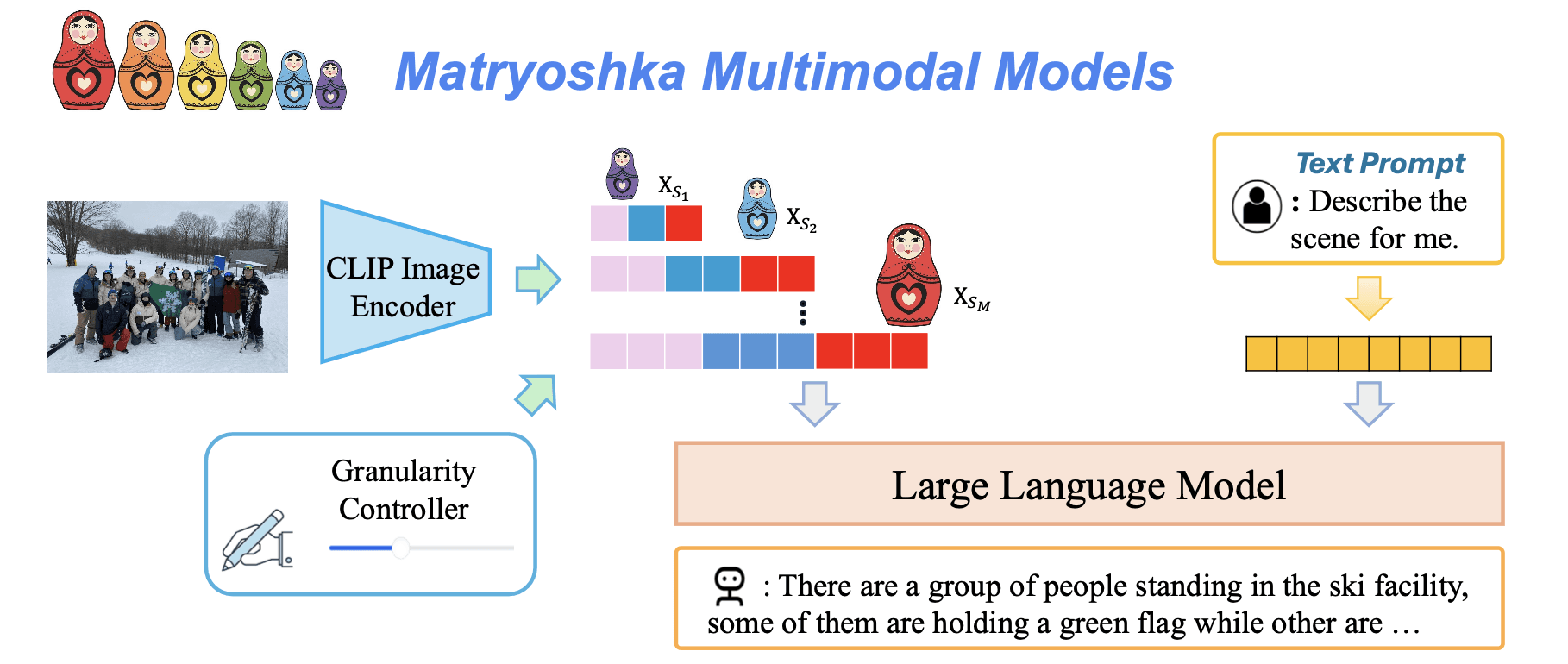
Technical Architecture
M3's training methodology is remarkably straightforward, taking the average of language generation loss across various visual token scales. The approach uses average pooling to obtain multi-granularity visual tokens, enabling a nested representation that proceeds from coarse to fine details.
Implementation Benefits
The implementation offers several significant advantages:
First, it maintains a simple design that uses vanilla LMM architecture and training data, requiring minimal code modifications and eliminating the need for a visual scale encoding module. Second, it provides unprecedented controllability, allowing researchers to explicitly adjust visual granularity during inference on a per-instance basis. Third, it serves as an analytical tool for understanding dataset requirements, revealing that many COCO-style benchmarks need only around 9 visual tokens to achieve performance comparable to using all 576 tokens.
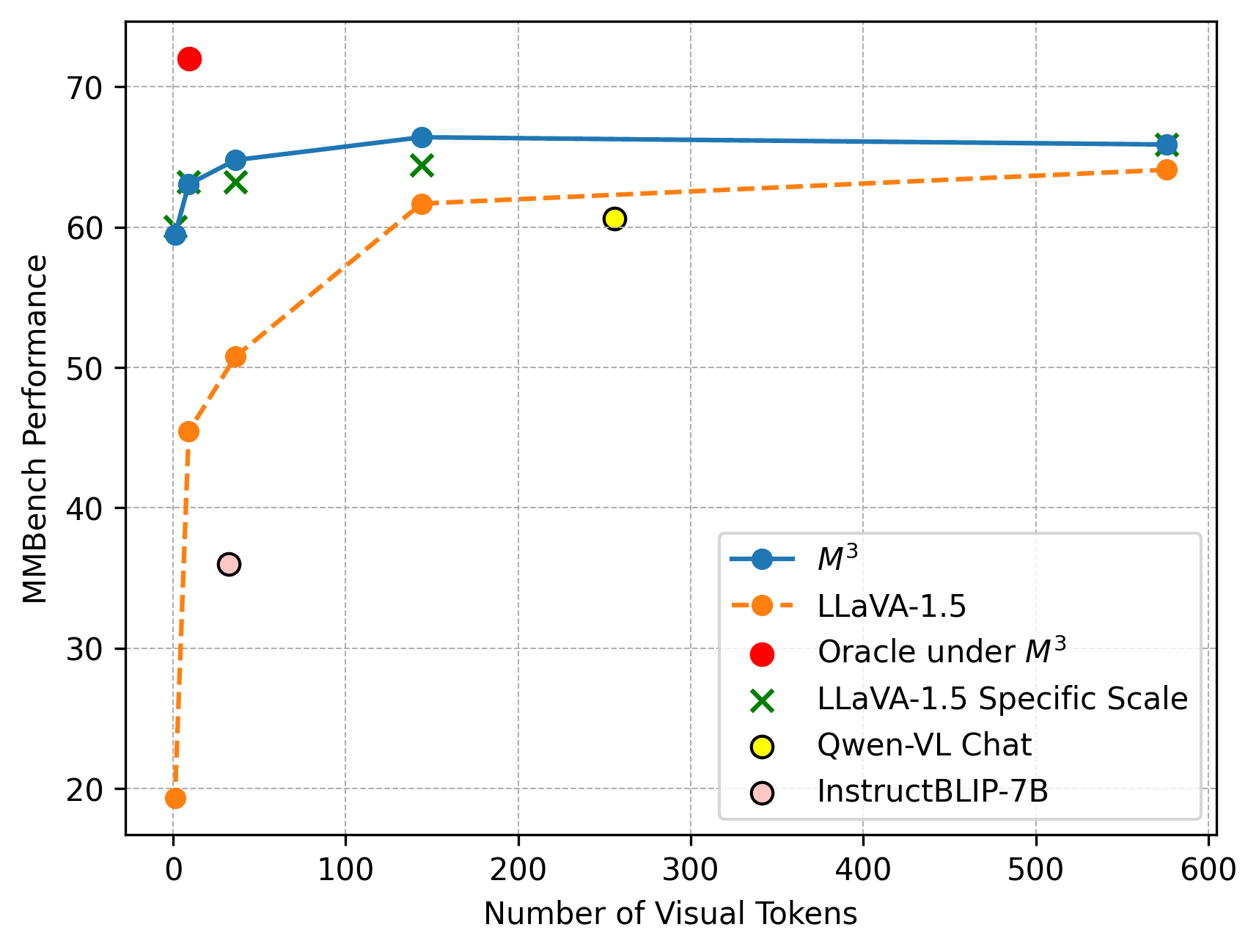
Empirical Results
Extensive testing on the MMBench revealed several crucial findings. The M3 variant of LLaVA-1.5-7B maintains or exceeds the performance of models trained on specific scales, while using significantly fewer resources. Particularly noteworthy is that M3 with just 9 visual tokens outperforms established baselines like InstructBLIP and Qwen-VL.
In video understanding tasks, full visual tokens often proved counterproductive. On four out of six benchmarks, using 720 or 180 tokens showed better performance than using the full token set, suggesting that excessive visual context might actually distract from accurate predictions. Most remarkably, for tasks like ActivityNet, IntentQA, and EgoSchema, using just 9 tokens per image grid (45 tokens total) performed within 1% accuracy of using the full 2880 tokens.
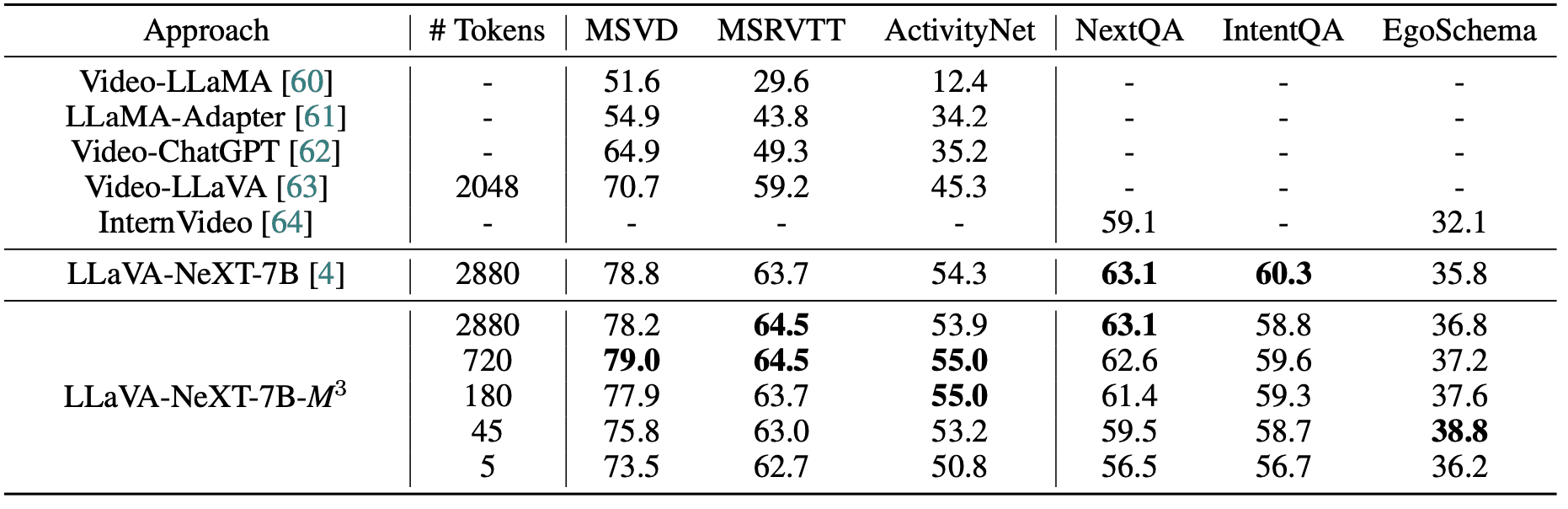
Broader Implications and Future Directions
The research presents several critical insights about the current state of video understanding models. While they show impressive capabilities in certain areas, they still fall significantly short of human-level intelligence. The Vinoground results particularly highlight how even short counterfactual temporal understanding remains challenging for current state-of-the-art models. Meanwhile, the M3 findings suggest that efficient processing of visual information might be more important than processing more visual tokens, pointing toward a promising direction for future model development.
4 - MovieGen: Foundation Model for Video Generation - Ishan Misra
Ishan Misra's presentation detailed Meta's MovieGen project, a groundbreaking initiative in text-to-video generation. The project addresses the fundamental challenge of generating videos from text input, with applications spanning creative content creation, video editing, image animation, simulation, and tools for both social media creators and Hollywood productions.
Evolution of Video Generation
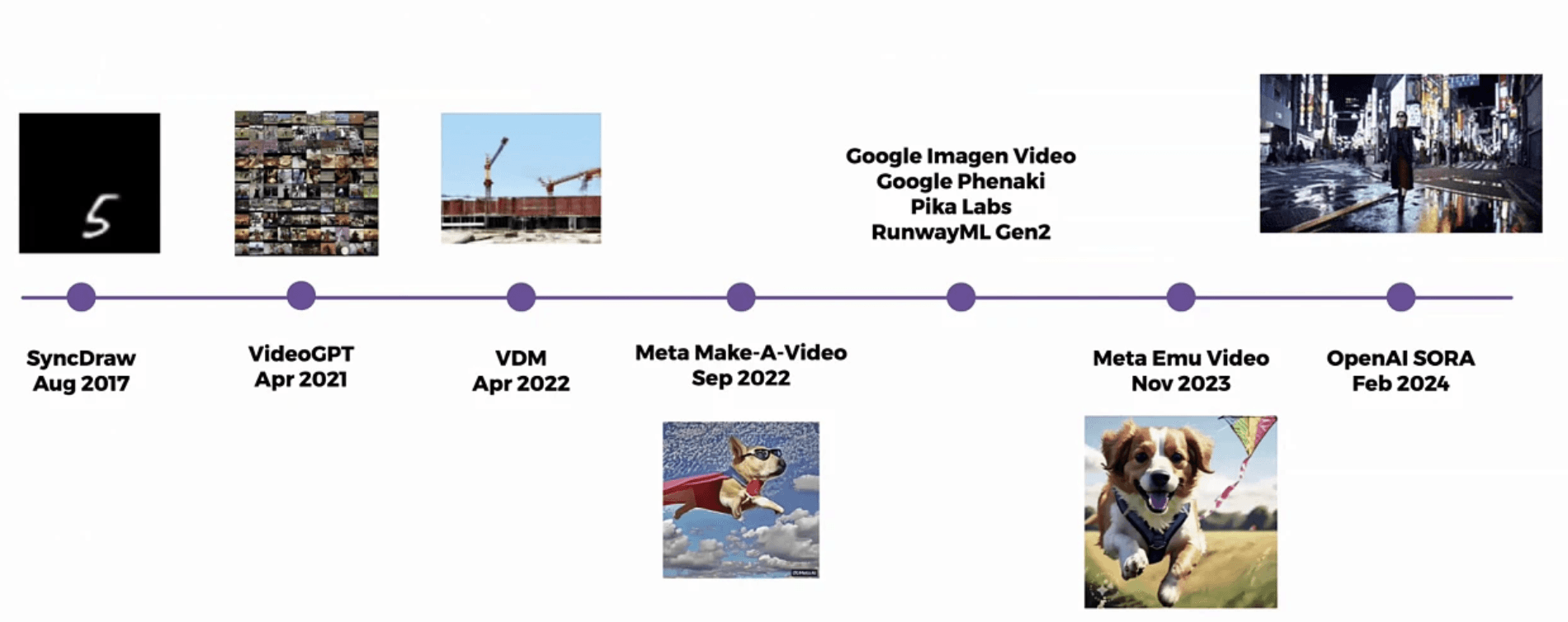
The presentation began with a comprehensive timeline of video generation models, highlighting the rapid progress in the field and setting the stage for MovieGen's contributions. This historical context emphasizes how the field has evolved from basic video synthesis to increasingly sophisticated generation capabilities.
Core Challenges in Video Generation
The field of video generation faces several fundamental challenges that MovieGen seeks to address. The first is the complex transformation from low-dimensional text input to high-dimensional video output, requiring sophisticated architectural solutions. Second, maintaining visual consistency and quality throughout generated videos demands robust temporal modeling. Third, the computational complexity of video generation necessitates efficient processing strategies and optimized architectures.
Technical Architecture and Innovations
1. Architectural Framework
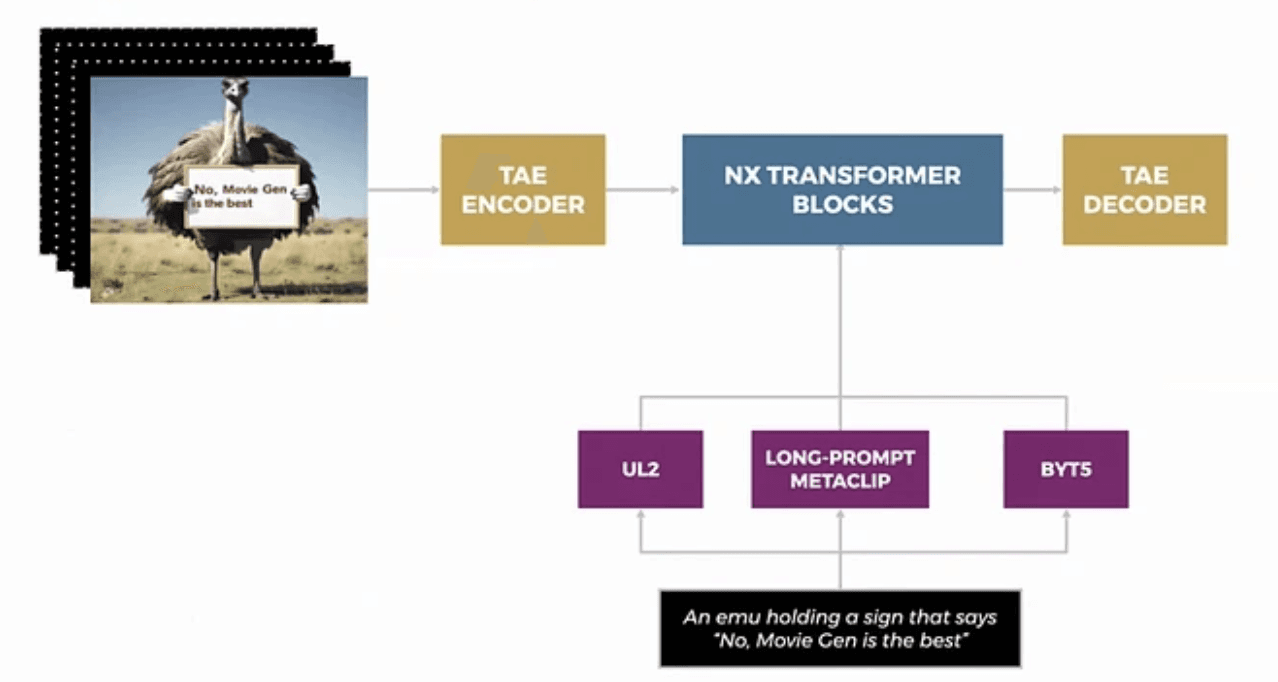
MovieGen's architecture represents a significant advancement in video generation, incorporating several innovative elements. The model employs enhanced text encodings that capture multiple aspects of input text prompts, enabling more nuanced understanding of generation requirements. The architecture is built on LLaMA-style Transformer blocks, which have been specifically adapted for video generation tasks, providing improved scalability and stability during training.
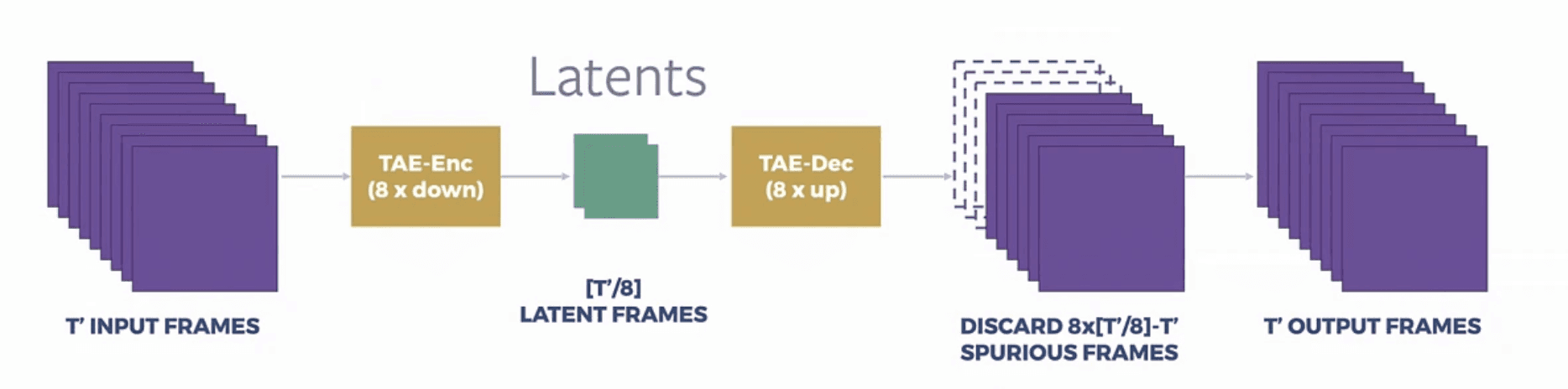
A key innovation is the model's approach to latent compression. MovieGen achieves an 8x reduction in video dimensions across height, width, and time dimensions, resulting in a remarkable 512x reduction in sequence length. This compression capability extends to both videos and images, allowing for variable-length sequence processing while maintaining generation quality.
2. Training Methodology
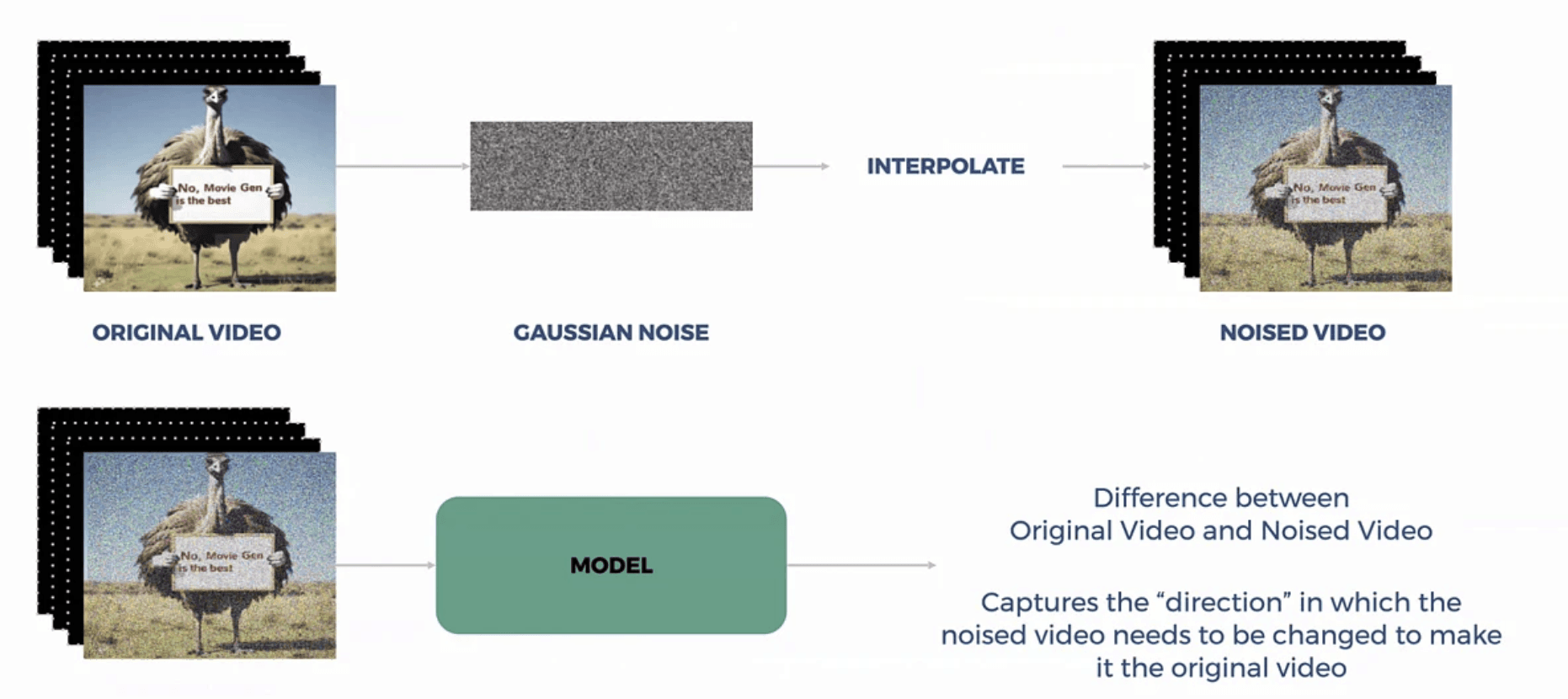
The training approach incorporates LLaMA-style blocks enhanced with diffusion Transformer (DiT) scale/shift modifications. This combination enables stable training at scale while allowing all parameters to be trained simultaneously on both images and videos, eliminating the need for frozen components. This end-to-end training approach contributes to the model's coherent understanding of both static and dynamic visual content.
3. Flow Matching Innovation
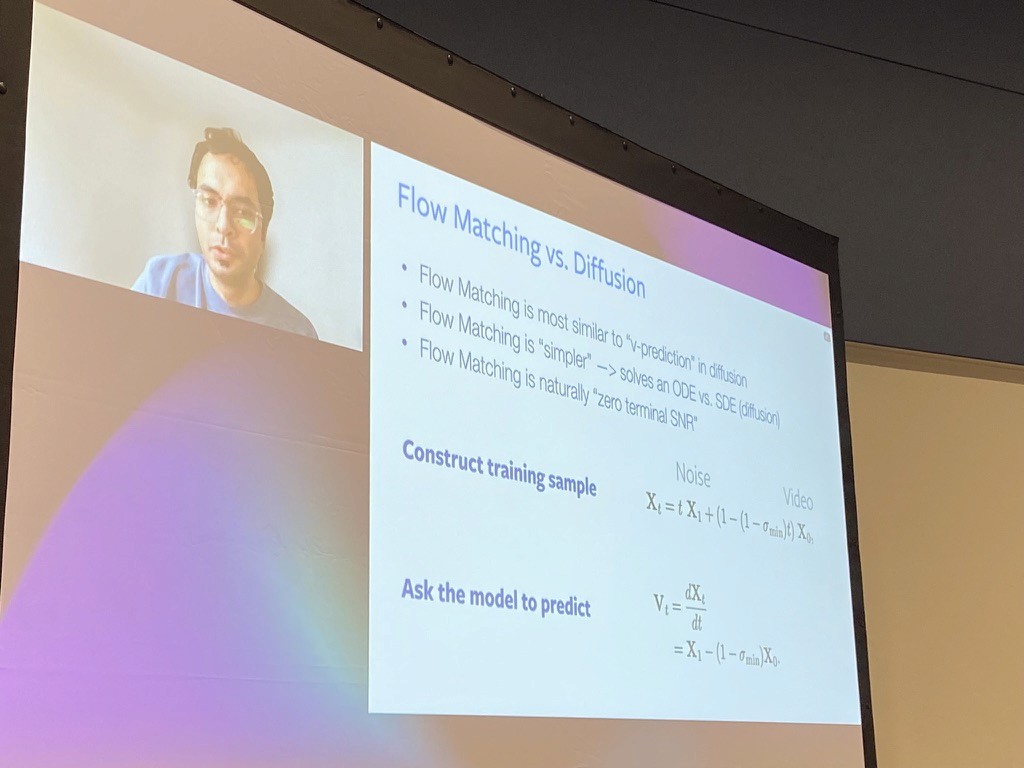
One of MovieGen's most significant technical innovations is its implementation of flow matching as an alternative to traditional diffusion approaches. This method is mathematically similar to "v-prediction" in diffusion but offers several crucial advantages. Instead of solving a stochastic differential equation (SDE) as in diffusion models, flow matching solves an ordinary differential equation (ODE), resulting in a simpler and more efficient process. The approach naturally achieves "zero terminal Signal-to-Noise ratio" characteristics, leading to both improved generation quality and faster inference speeds compared to diffusion-based methods.
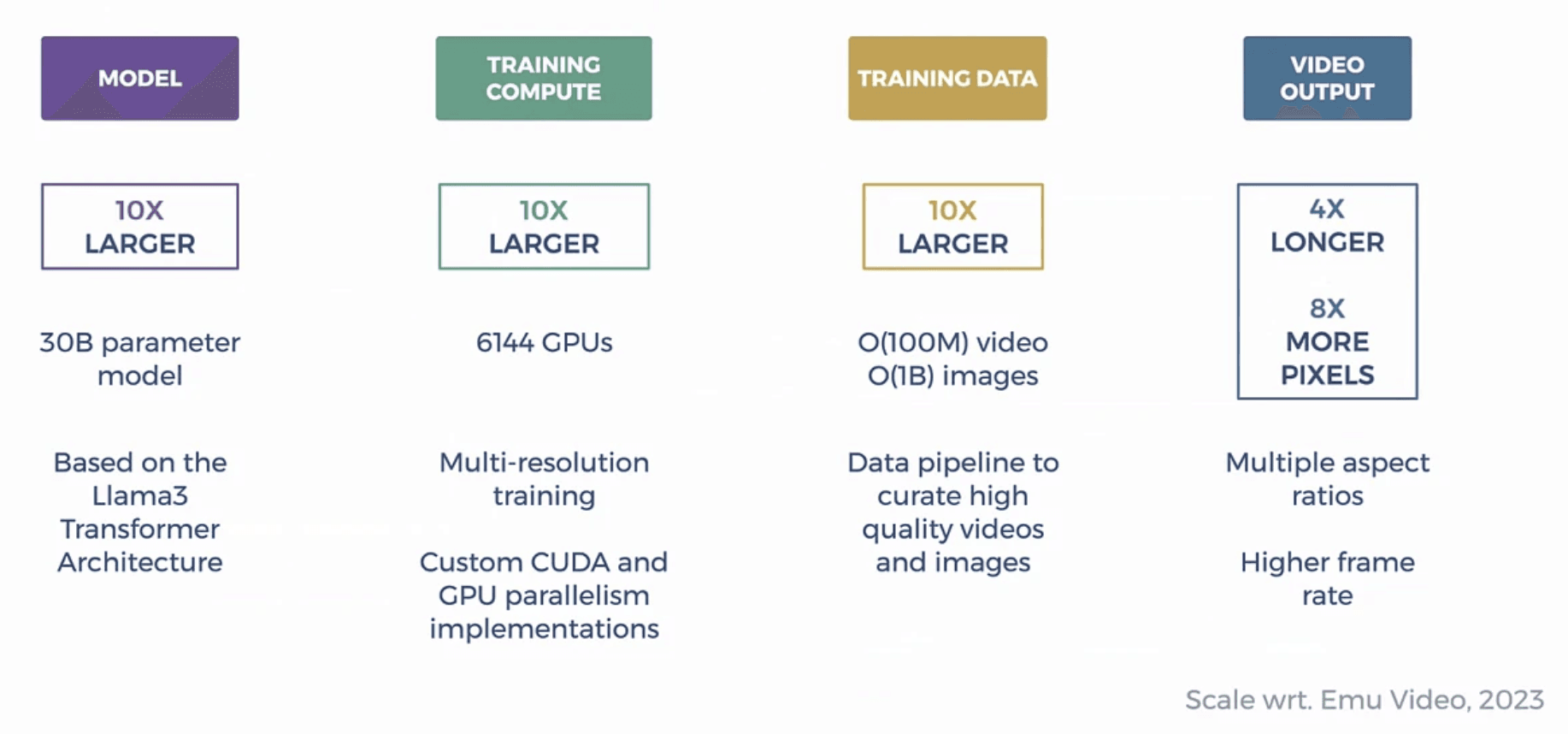
Training Data and Scaling
MovieGen's training methodology represents a significant scaling effort in both data and computation. The training dataset encompasses approximately 100 million videos and 1 billion images, with videos varying in length and frame rate to ensure robust generalization. A notable innovation is the use of LLaMA-3 for video caption generation, which substantially improves the model's ability to follow text prompts accurately.
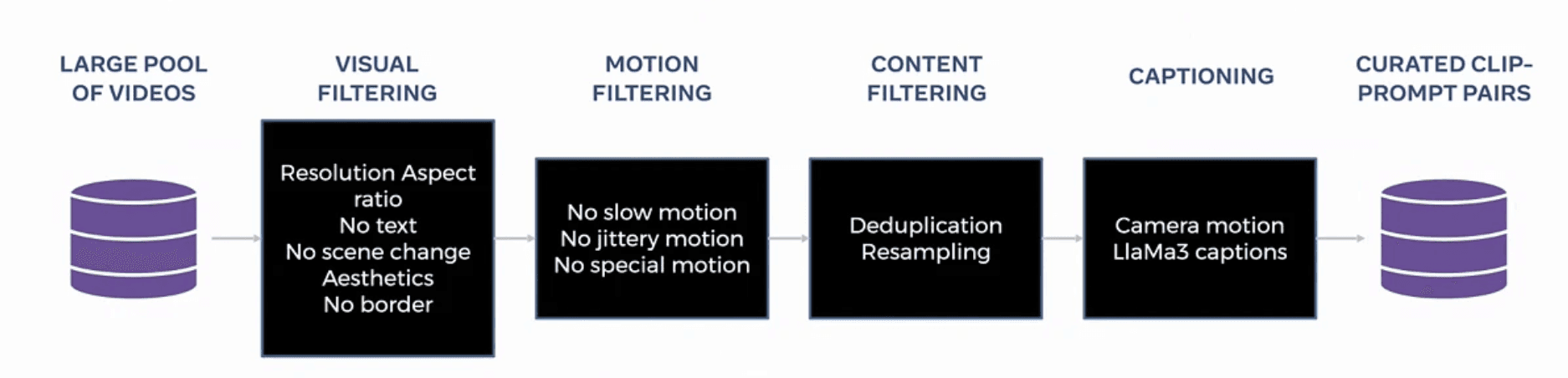
Comprehensive Evaluation Framework
The Meta team developed MovieGen Bench, a rigorous evaluation framework that surpasses previous benchmarks in both scale and comprehensiveness. This benchmark includes 1,000 non-cherry-picked videos, available publicly on GitHub, and offers three times more evaluation data than previous benchmarks. The evaluation methodology demonstrated a strong correlation between validation loss and human judgment, providing a reliable metric for model performance.
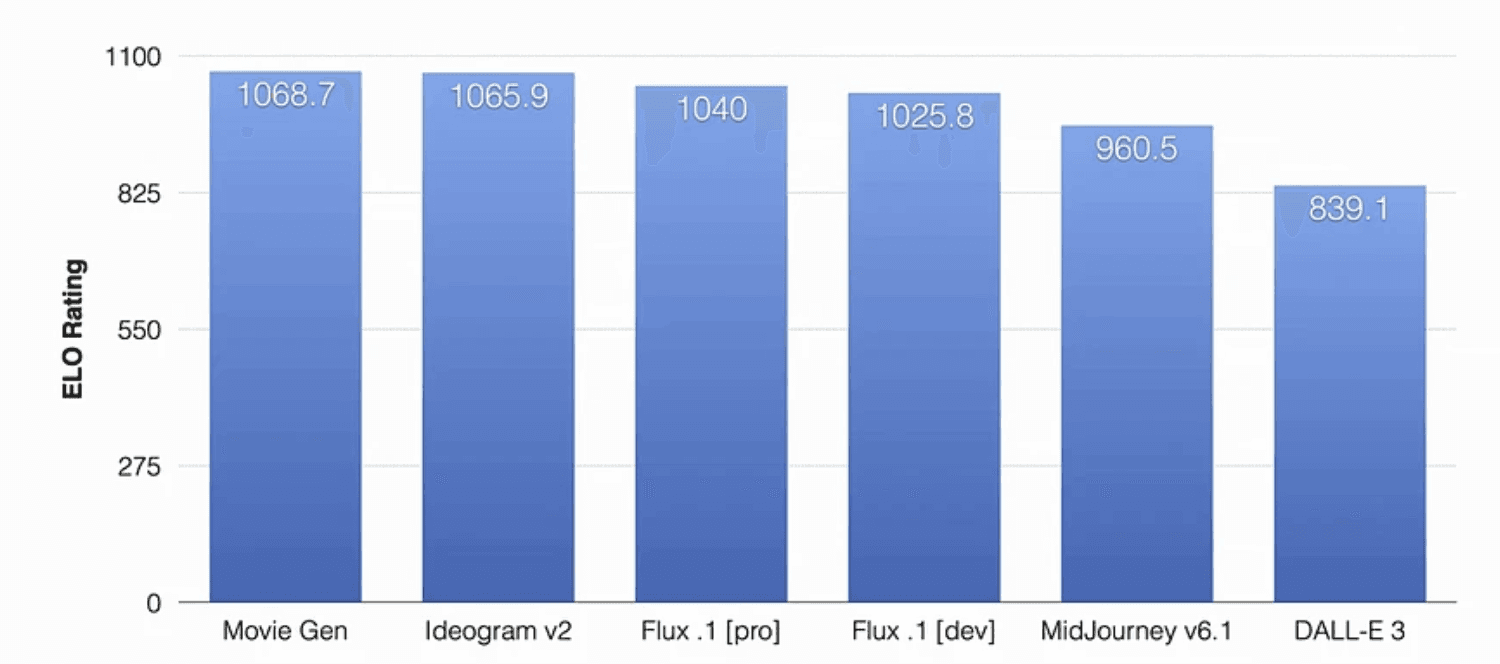
Performance and Future Directions
The evaluation results revealed impressive capabilities in several key areas. MovieGen showed competitive performance in both overall quality and realness metrics, with particularly strong results in text-to-image generation capabilities. The research uncovered important insights about scaling laws, notably that model performance has not yet reached saturation and that video generation scaling laws closely mirror those observed in LLaMA-3.
While MovieGen represents a significant advance in video generation capabilities, Ishan acknowledges important limitations and future challenges. Particularly, the extent of the model's ability to combine and generalize its understanding of the world remains an active area of investigation. This honest assessment of current limitations helps guide future research directions in the field of video generation.
5 - Towards Multimodal Agentic Models - Jianwei Yang
Jianwei Yang's presentation challenged the traditional focus on pure understanding in AI systems, arguing that interaction, not understanding alone, should be the ultimate goal. His comprehensive talk outlined a roadmap for developing multimodal AI agents capable of both understanding the past and acting for the future, with applications spanning web agents, robotics, and autonomous driving.
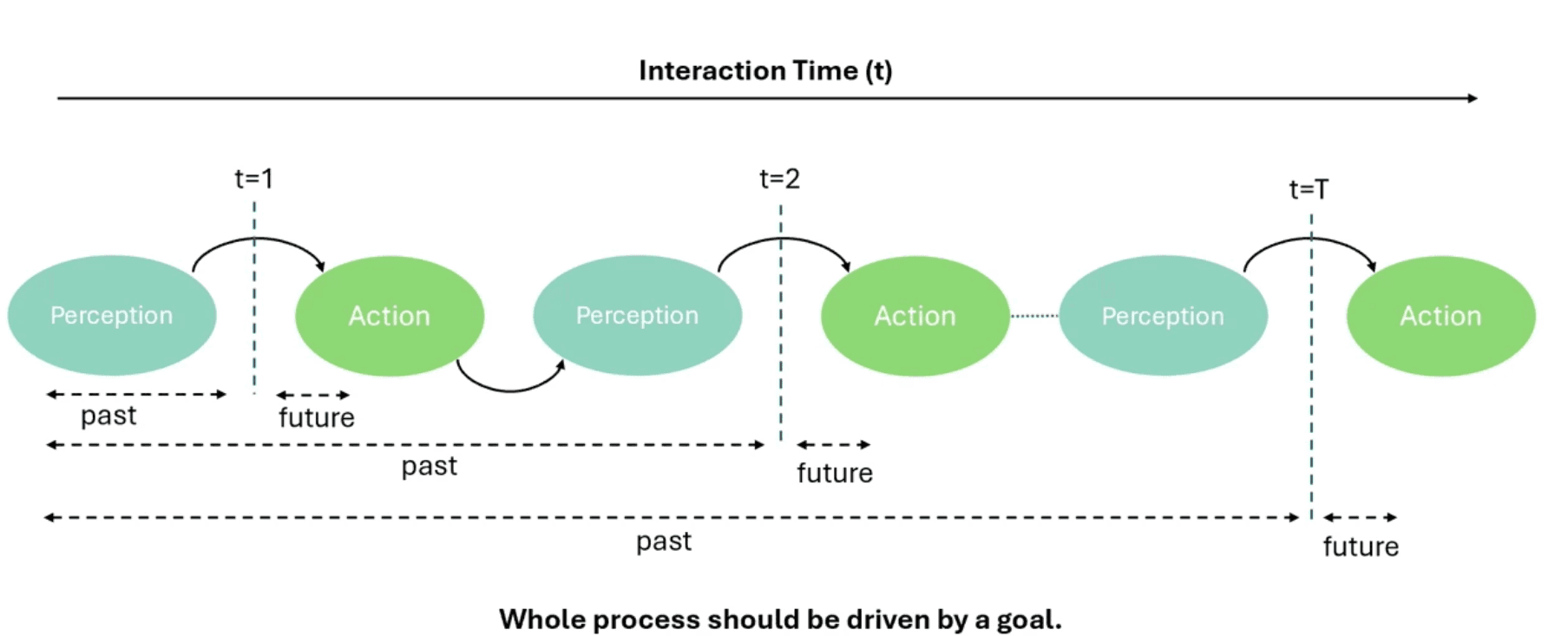
Understanding the Past: Enhanced Visual Perception
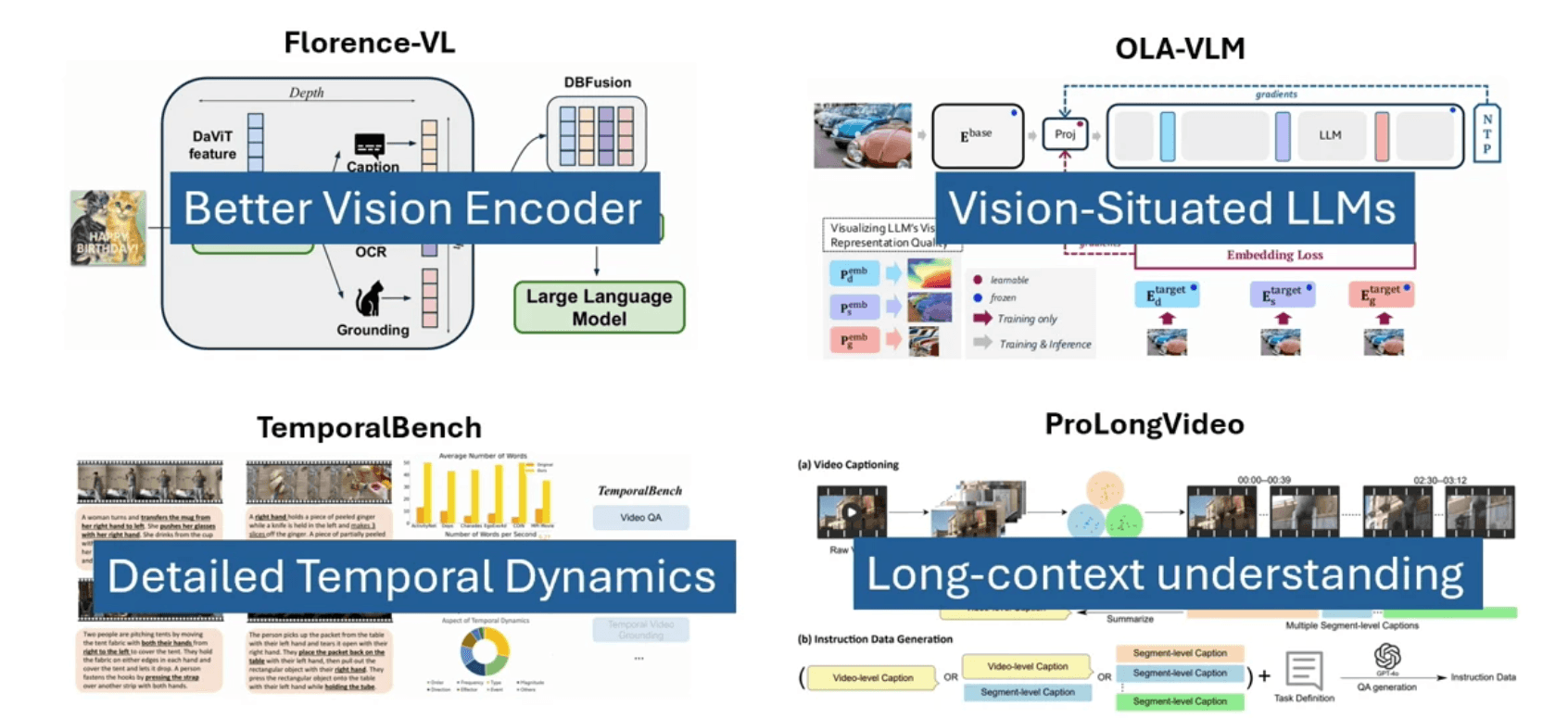
Florence-VL: Enhanced Vision-Language Models
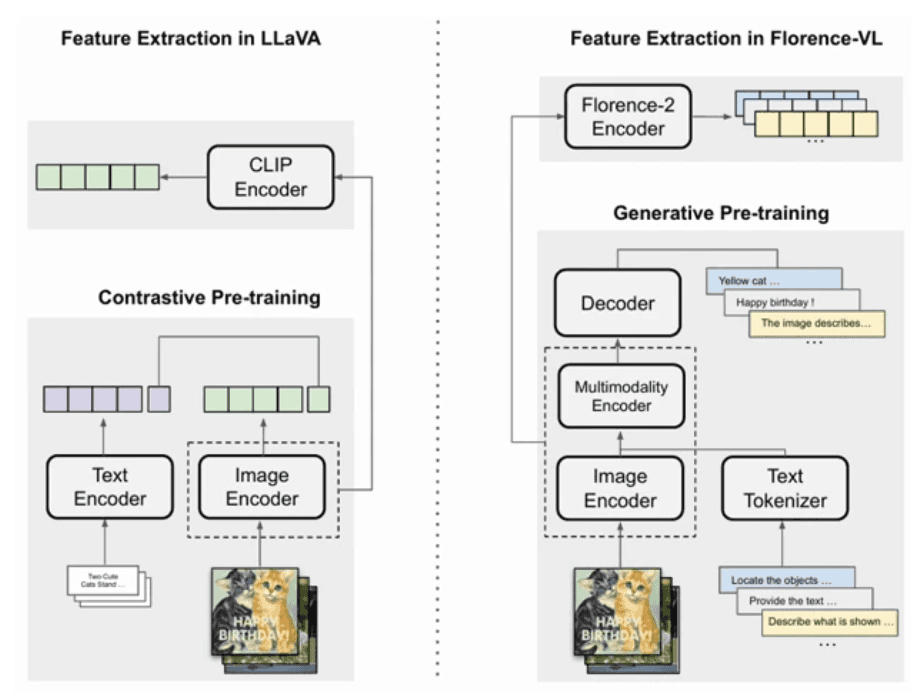
Florence-v2 represents a significant advancement in text-conditioned generative vision-language models. The model's architecture introduces a novel approach to visual perception through different prompts designed for various types of visual features. These features work complementarily, creating a richer overall representation of visual information. The model's effectiveness is measured through alignment cost between output features from the vision encoder and LLM encoder, providing a quantitative metric for evaluation.
OLA-VLM: Deepening Visual Understanding
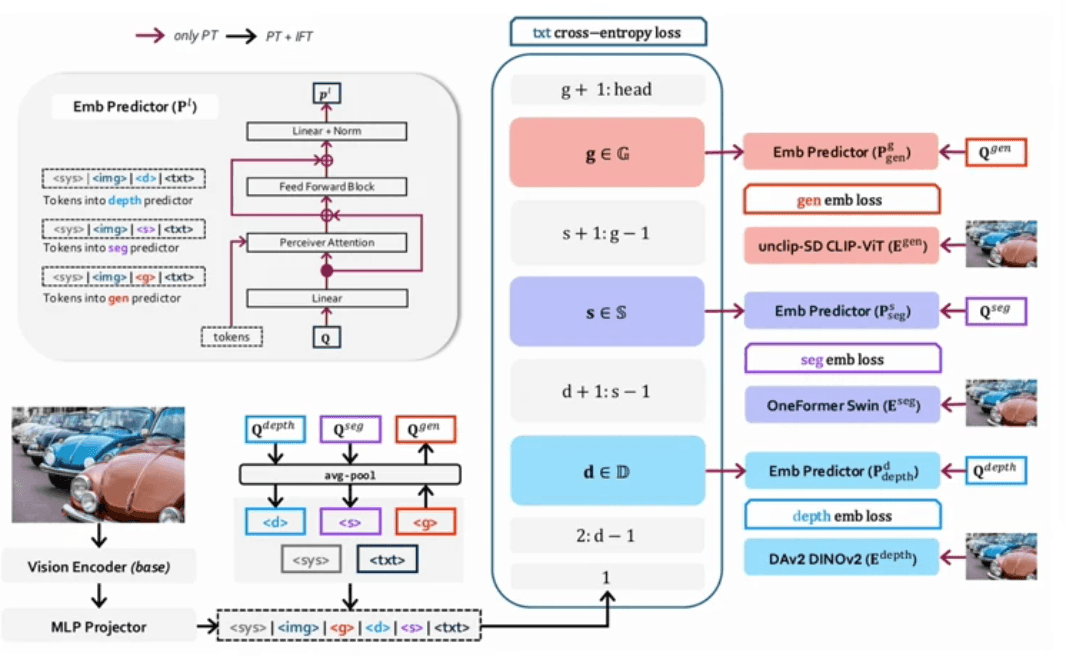
OLA-VLM pushes the boundaries of visual perception in multimodal LLMs by incorporating three distinct visual features for each image:
Depth features that capture spatial relationships
Segmentation features for object delineation
Unclip-SD features for enhanced visual representation
The research demonstrates that increasing text supervision leads to improved intermediate features for vision perception. A key innovation is the direct optimization of LLM features using "teacher" models, resulting in more robust visual understanding capabilities.
Fine-grained Temporal Dynamics
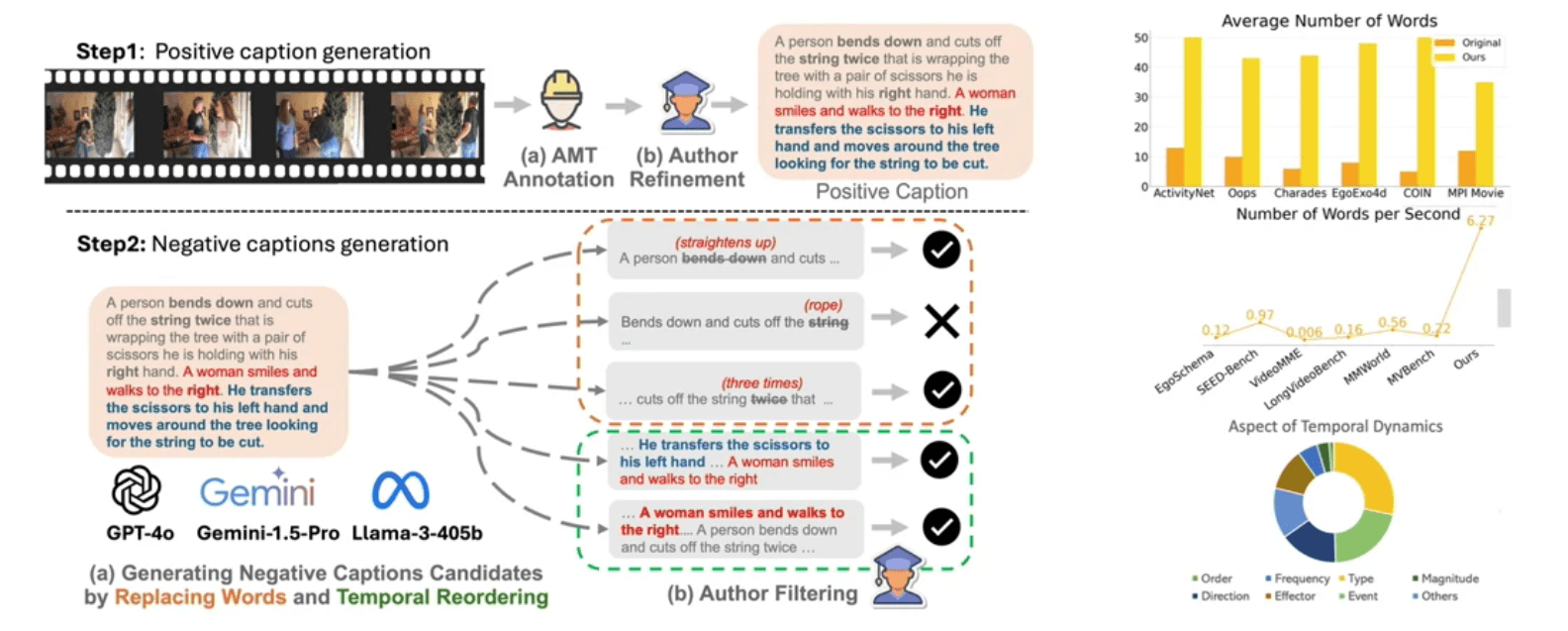
The TemporalBench project addresses a fundamental aspect of video understanding: videos contain not just static contents in individual frames but crucial fine-grained dynamics. This benchmark provides a comprehensive evaluation framework for assessing temporal understanding capabilities in multimodal video models.
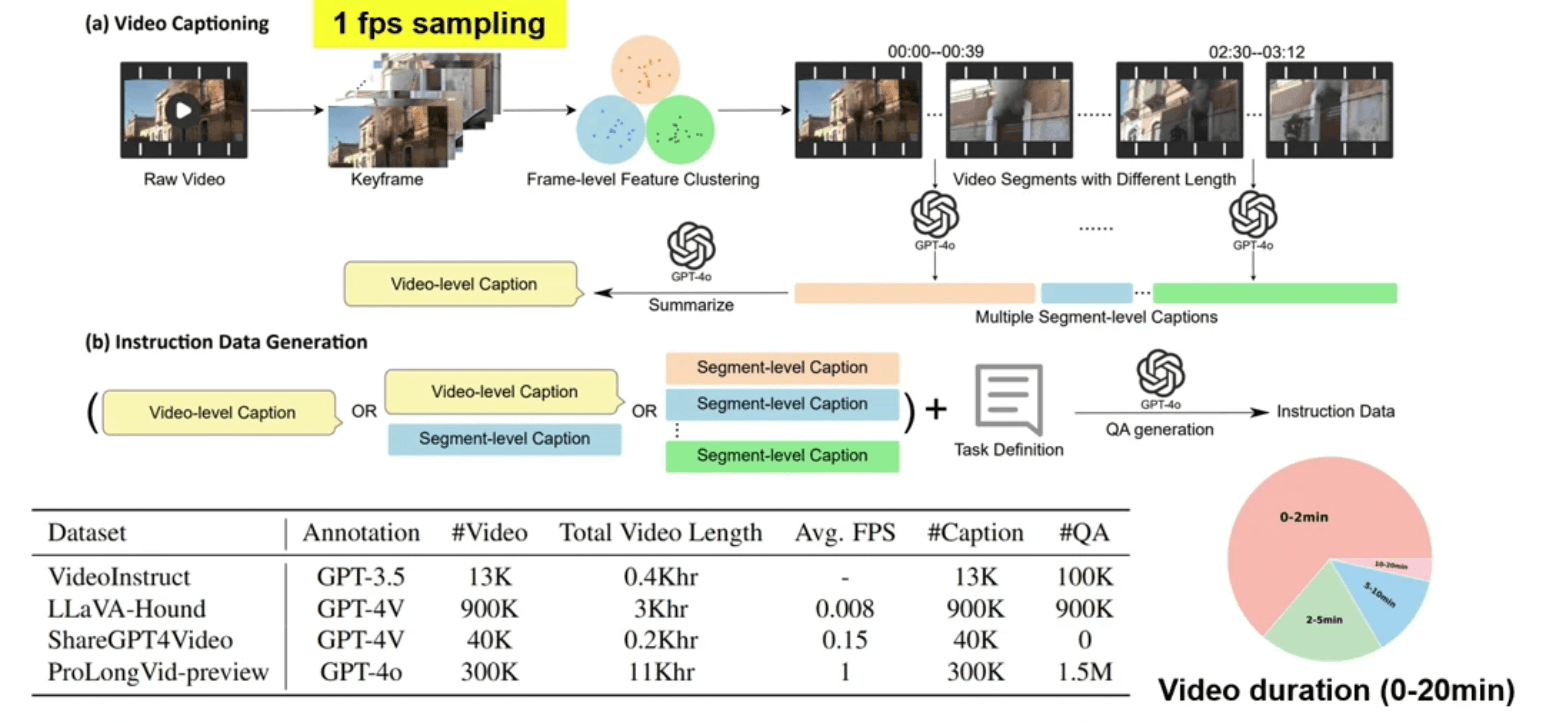
ProLongVid complements this work by focusing on two critical scaling dimensions:
Scaling video length during instruction data synthesis, enabling models to handle longer temporal sequences
Scaling context length during both training and inference, improving the model's ability to maintain coherent understanding across extended temporal spans
Acting for the Future: Multimodal Agentic Models
TraceVLA: Visual Trace Prompting
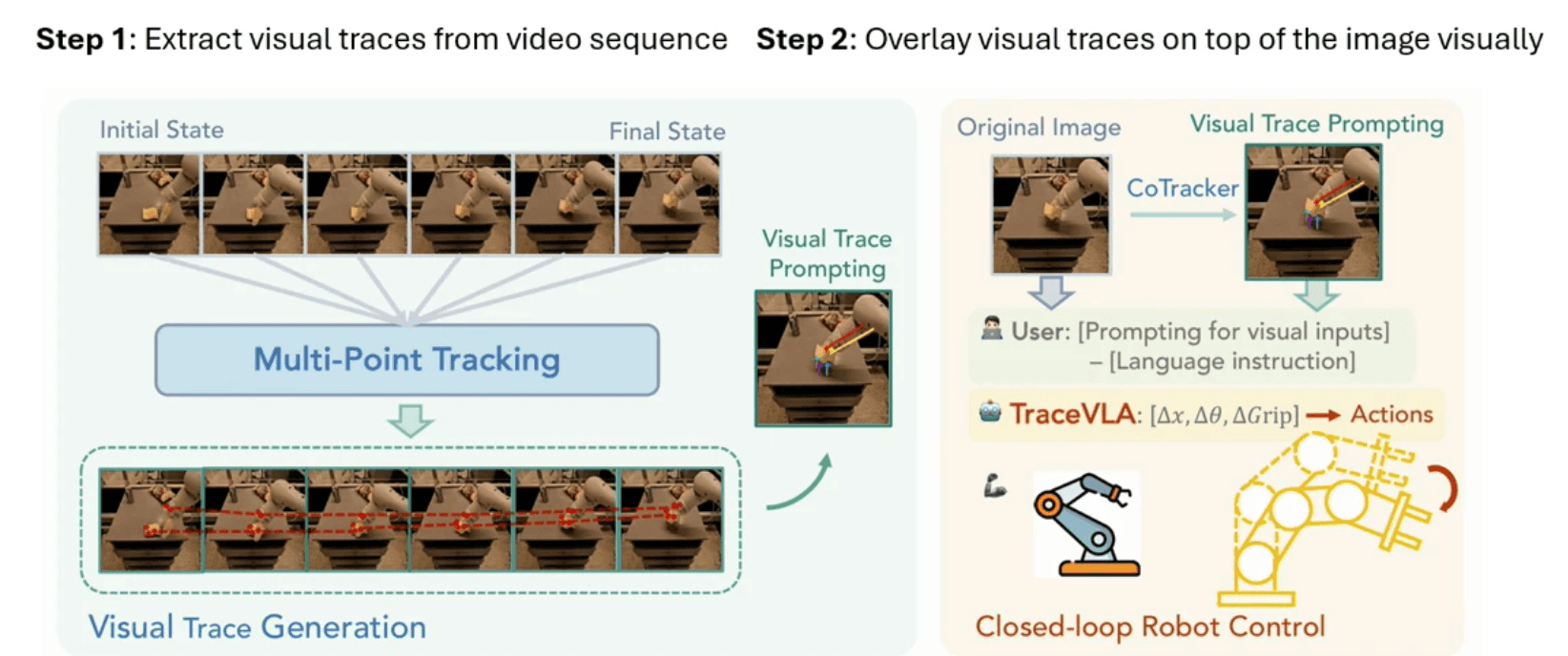
TraceVLA introduces a novel approach to enhancing spatial-temporal awareness for robotic policies. The core intuition behind this work is that better understanding of history facilitates better prediction of future actions. The system addresses several key challenges in multimodal processing:
Multi-image processing presents a significant challenge by increasing the number of visual tokens, while vision encoders struggle to capture subtle changes or motions across adjacent frames.
Text prompts, while easy to implement, fall short in capturing fine-grained movement information and spatial locations.
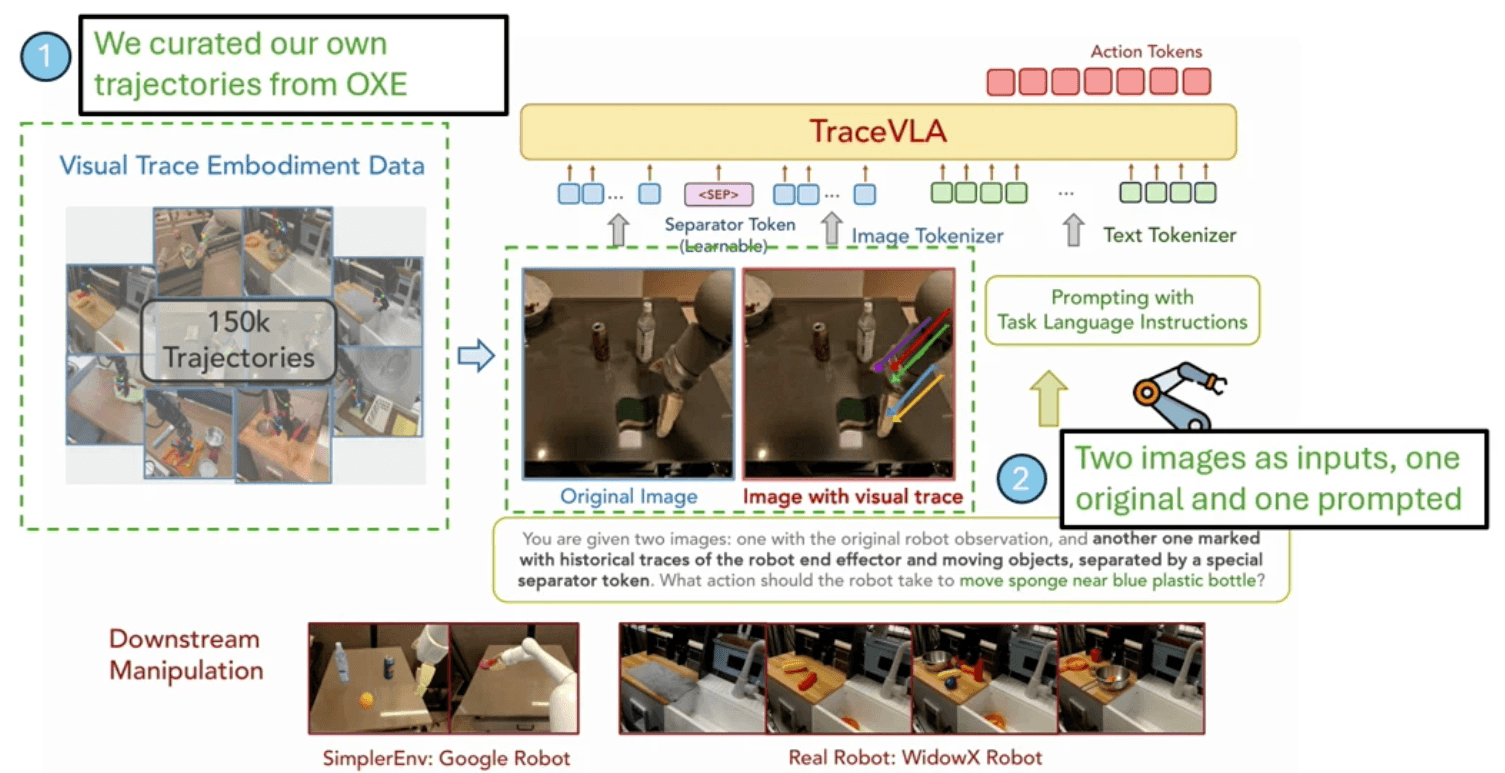
The innovation comes through visual prompts, which prove particularly effective through Set-of-Mark prompting, naturally building associations between current observations and historical context.
LAPA: Latent Action Pretraining
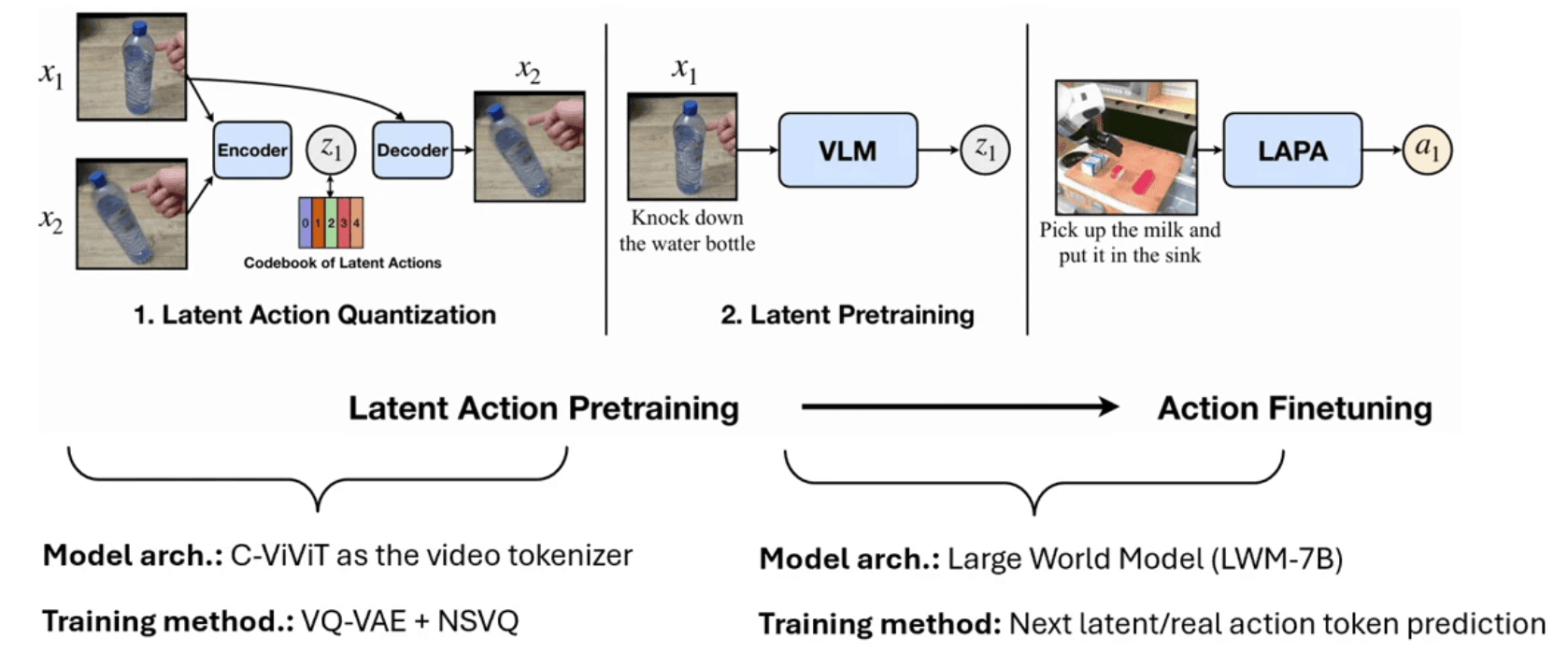
LAPA represents a breakthrough in leveraging unlabeled video data for robotics policy training. The system demonstrates impressive capabilities in learning from both robotics data without actions and human instructional videos. Using sthv2 as the human instructional video dataset, LAPA achieves significant improvements over models trained from scratch, though some gap remains compared to models pre-trained on robotics-specific data.
MAGMA: Foundation Model for Multimodal AI Agents
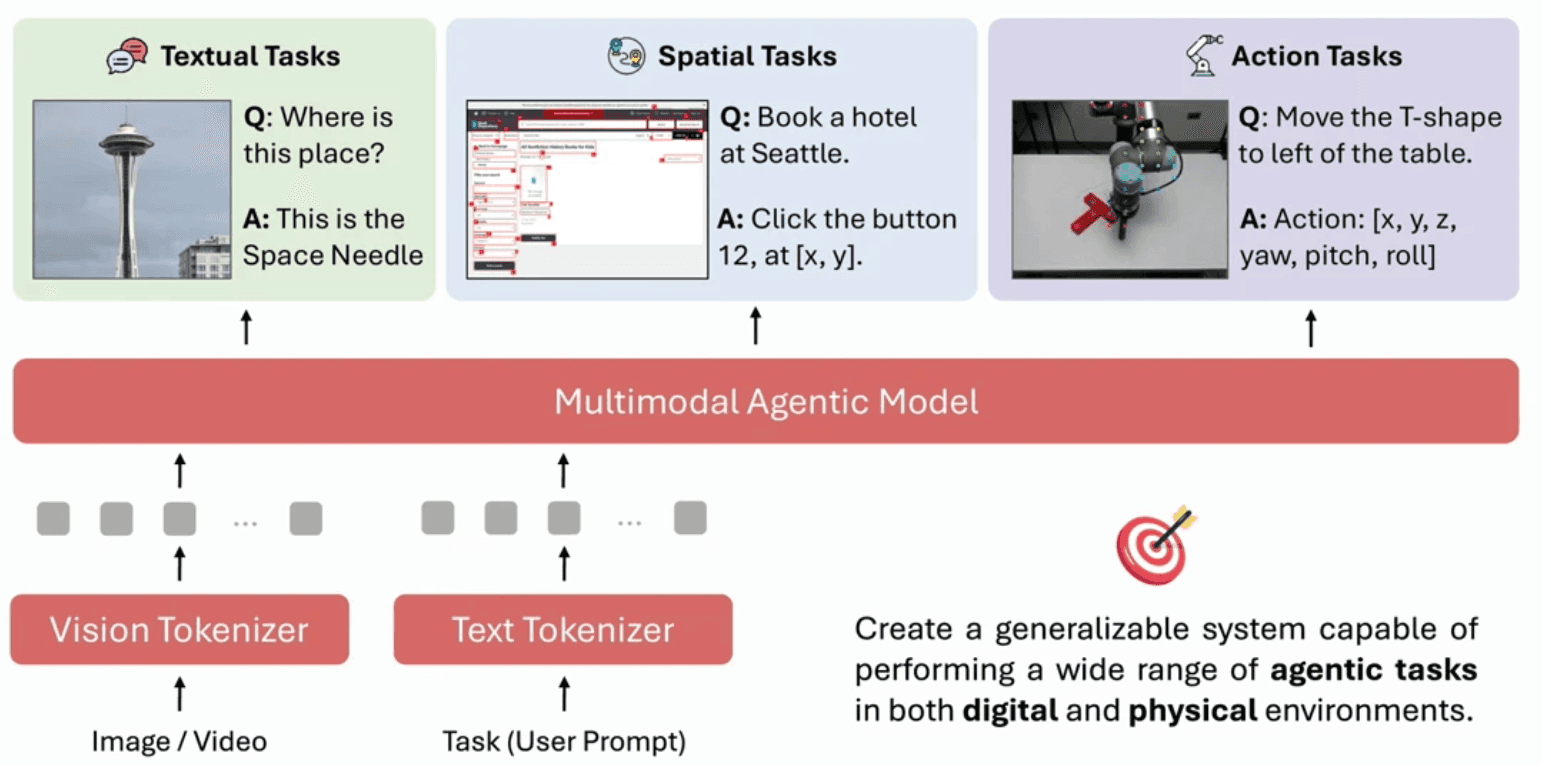
MAGMA introduces a comprehensive foundation model for multimodal AI agents, incorporating pre-training across a diverse range of images, videos, and robotics data. The model architecture is designed to facilitate both understanding and action, with particular emphasis on temporal motion supervision and action conversion. The pre-training tasks are carefully designed to develop emergent capabilities in robot trajectory planning, demonstrating effects of data scaling and task generalization.
Redefining Multimodal AI Agents
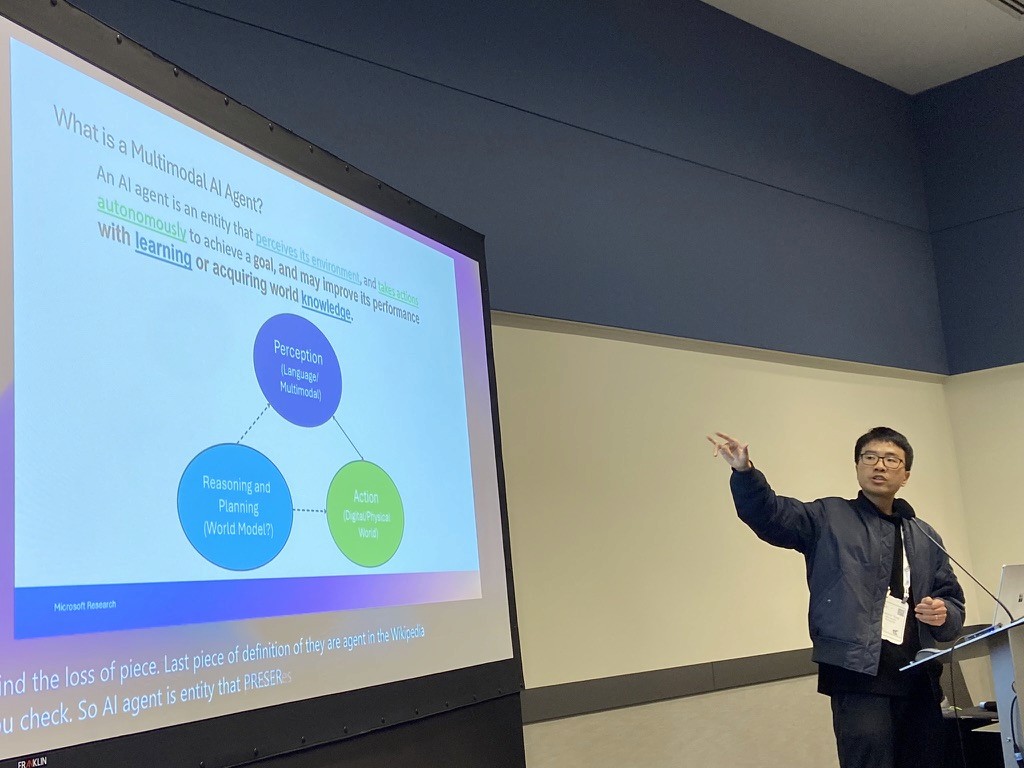
Yang concluded his talk by proposing an expanded definition of multimodal AI agents: "An AI agent is an entity that perceives its environment and takes actions autonomously to achieve a goal, and may improve its performance with learning or acquiring world knowledge." This definition emphasizes three critical aspects:
The transition from 2D to 3D perception for multimodal agents, acknowledging the importance of spatial understanding
The balance between model-free and model-based approaches in multimodal agent development
The crucial role of reasoning and planning in agent behavior
Future Directions and Challenges
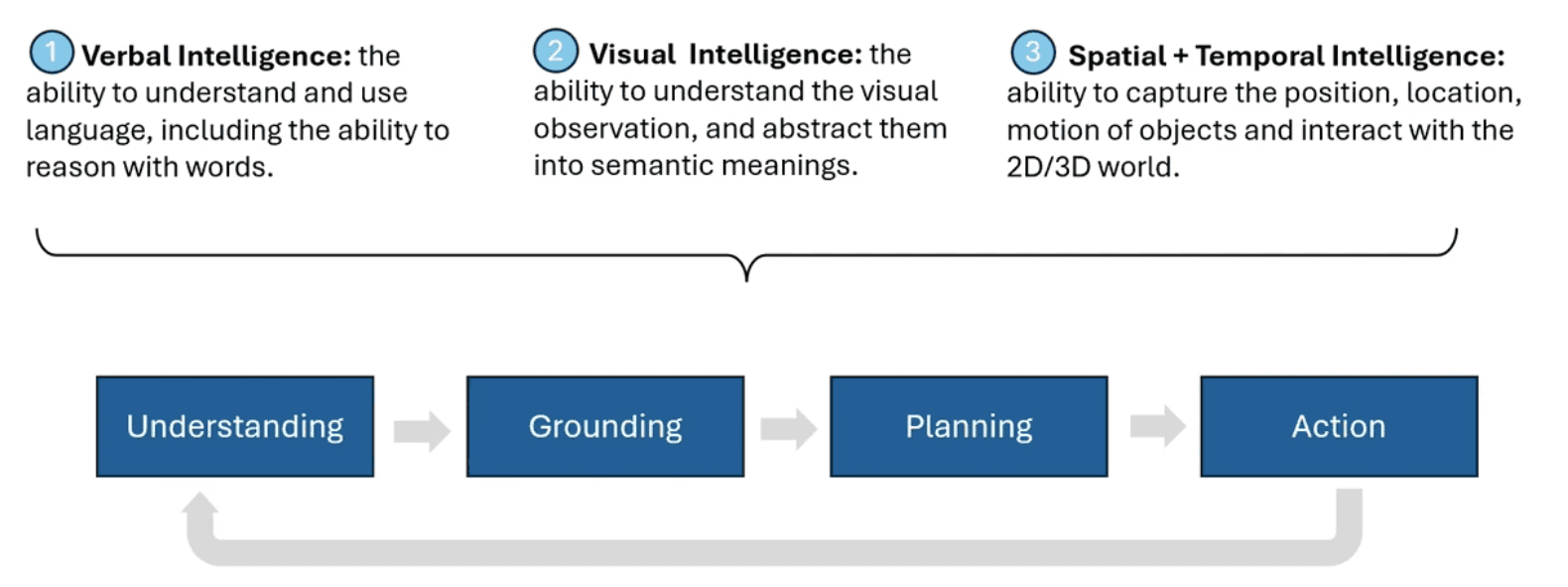
Yang emphasized that scaling remains a vital factor in advancing the field, particularly given the vast potential of video data and real-world interactions. He outlined several key directions for future research:
Multimodal Agentic Capabilities: Developing more sophisticated interaction abilities
Multimodal Reasoning: Enhancing the ability to make logical connections across modalities
Multimodal Understanding: Deepening comprehension of complex multimodal inputs
Multimodal Self-Awareness: Building systems with greater metacognitive capabilities
The presentation concluded with an important observation about current limitations: while visual understanding has improved significantly, it still falls short of human intelligence. Long-context video understanding, detail comprehension, and temporal dynamics remain challenging areas, particularly given the scarcity of high-quality video-text paired data despite the abundance of raw video content.
6 - From Video Generative Models to General World Models - Doyup Lee
Doyup Lee concluded our workshop exploring Runway’s journey from video generative models to general world models, centered around a fundamental question: "How can an intelligent machine understand the visual world?" His presentation delved deep into the nature of visual understanding and the role of imagination in AI systems, drawing from Runway's extensive experience in developing cutting-edge video generation models.
Understanding Visual Intelligence
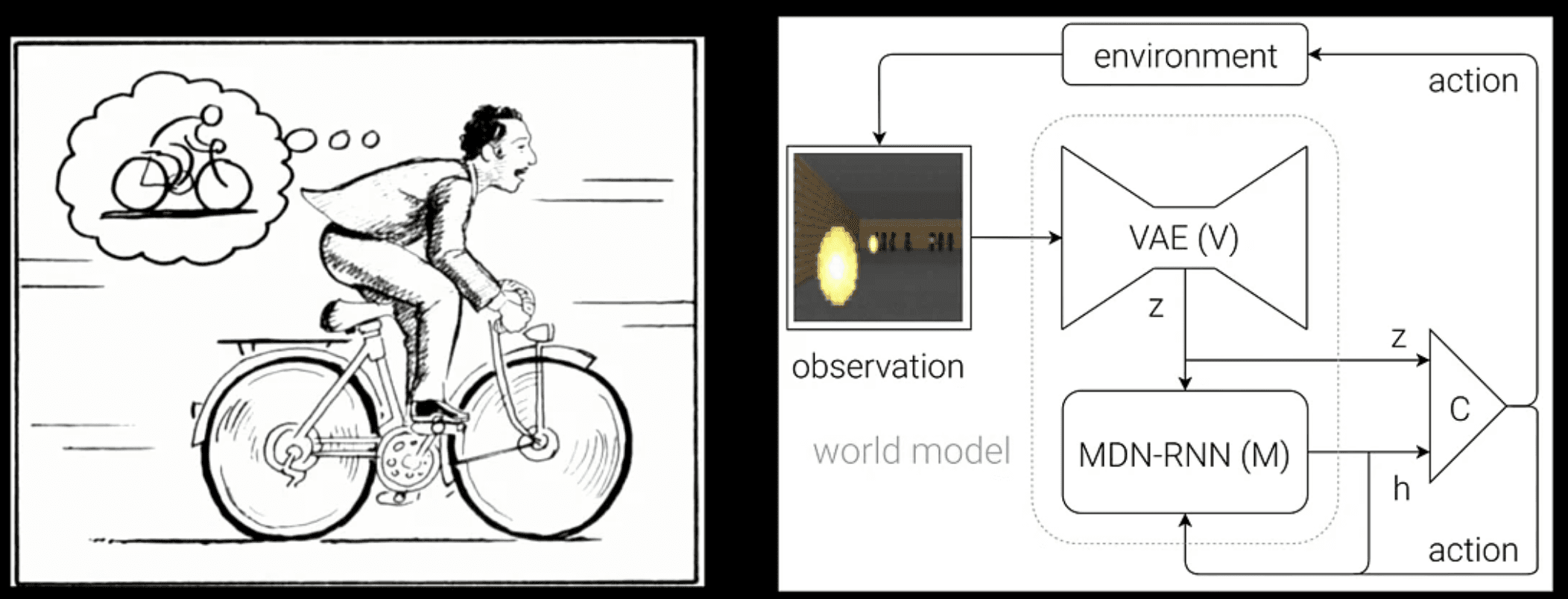
Lee began by examining what constitutes "visual understanding" through the lens of imagination and world modeling. He referenced the seminal work by Ha and Schmidhuber (2018) on World Models, which achieved impressive results in future predictions but remained confined to specific simulators or environments. Traditional visual recognition tasks - including image captioning, visual question answering, and semantic segmentation - have primarily focused on aligning visual information with human knowledge through text, but these approaches are inherently limited by the scope of visual data and world knowledge they can process.
The Platonic Representation Hypothesis
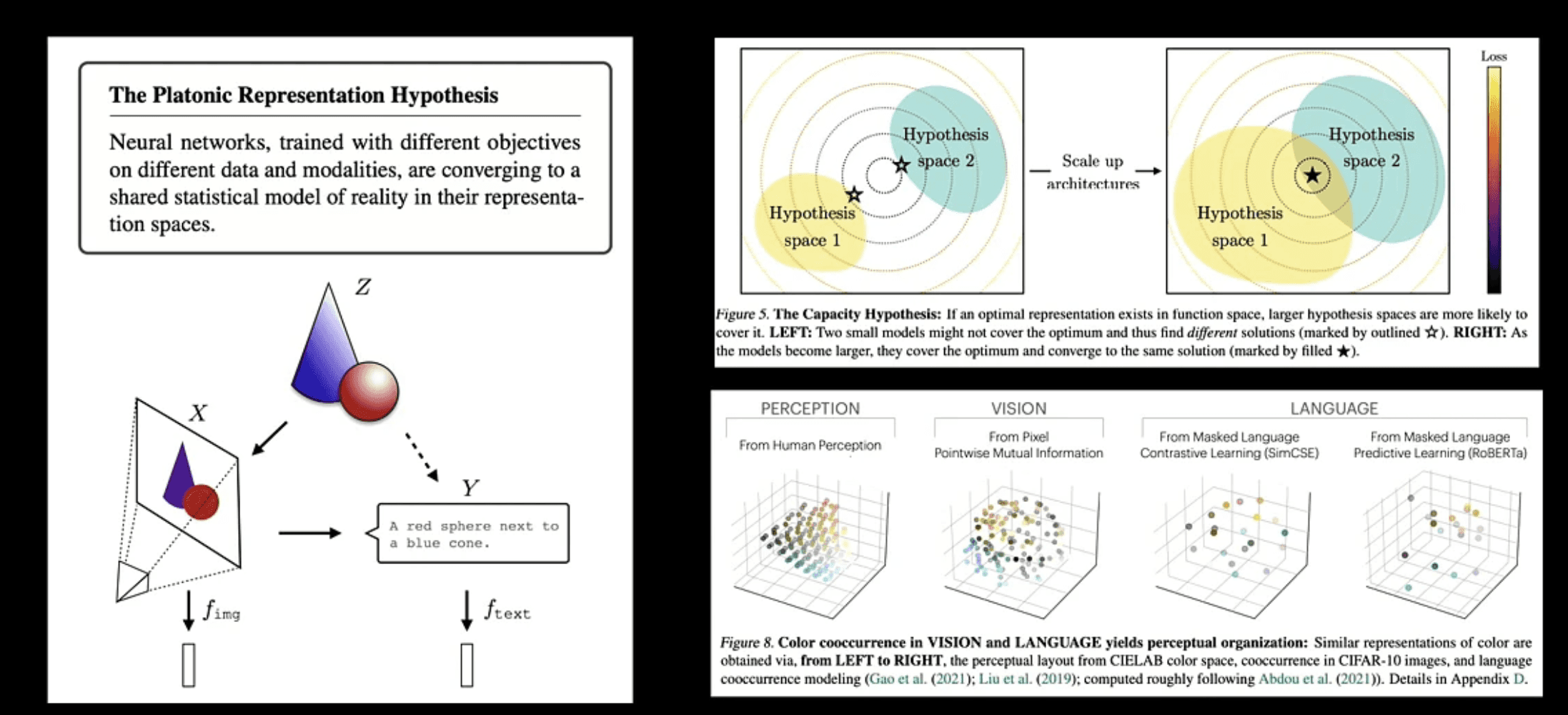
A cornerstone of Lee's theoretical framework is the Platonic Representation Hypothesis (Huh et al., ICML 2024), which identifies three essential components for visual understanding:
The ability to achieve high performance in future prediction tasks, demonstrating true comprehension of visual dynamics
Access to and effective utilization of large and diverse video datasets that capture the complexity of the visual world
Strong alignment with human knowledge, ensuring that the model's understanding corresponds to human concepts and expectations
The Five Essential Recipes for General World Models
1. The Bitter Lesson
Lee emphasized the importance of embracing the "bitter lesson" in AI development: that general methods leveraging computation and learning ultimately triumph over hand-crafted, specialized approaches. This principle guides the development of more flexible and scalable architectures.
2. High-quality and Large-scale Data
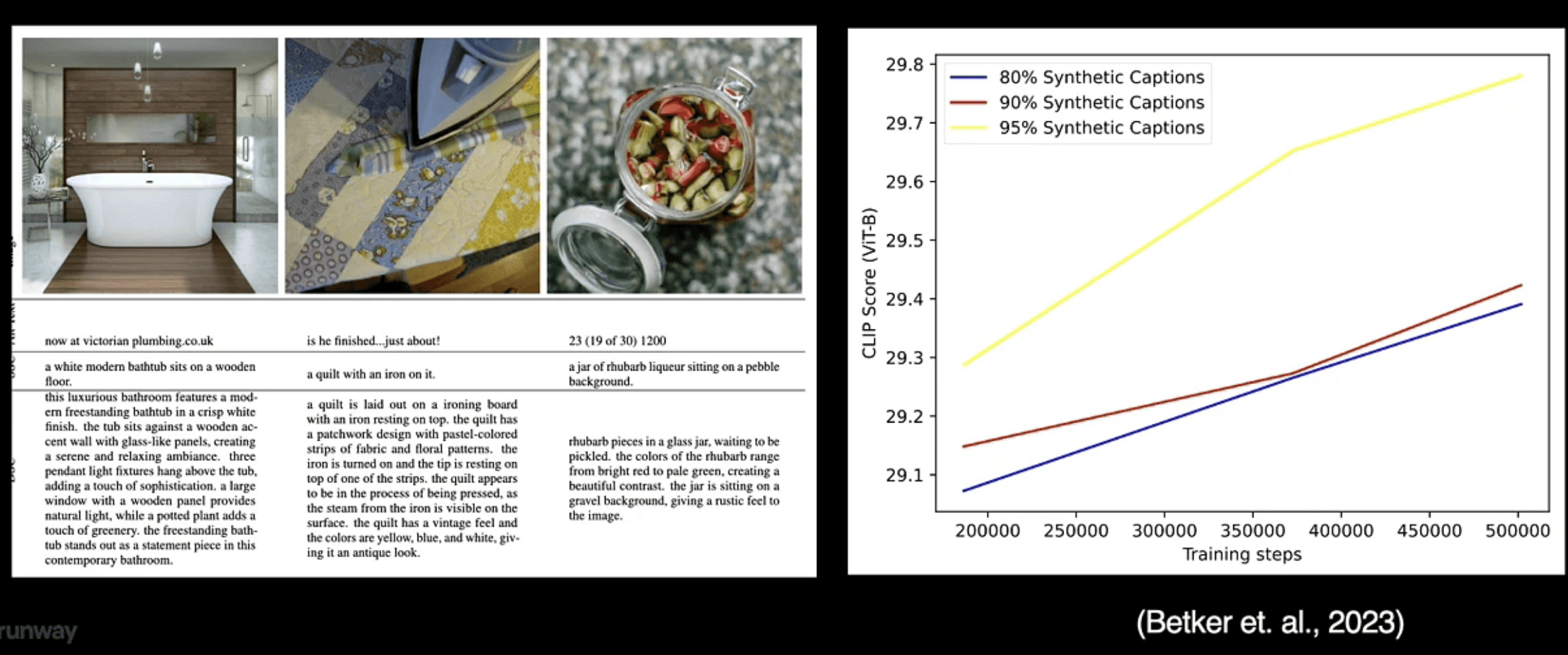
The presentation highlighted the critical role of data quality in model performance. Lee demonstrated how high-quality captions significantly improve both image and video models' capabilities. However, he noted that obtaining high-quality, large-scale video captions remains a significant challenge in the field, requiring innovative approaches to data collection and curation.
3. Scalable Neural Architecture
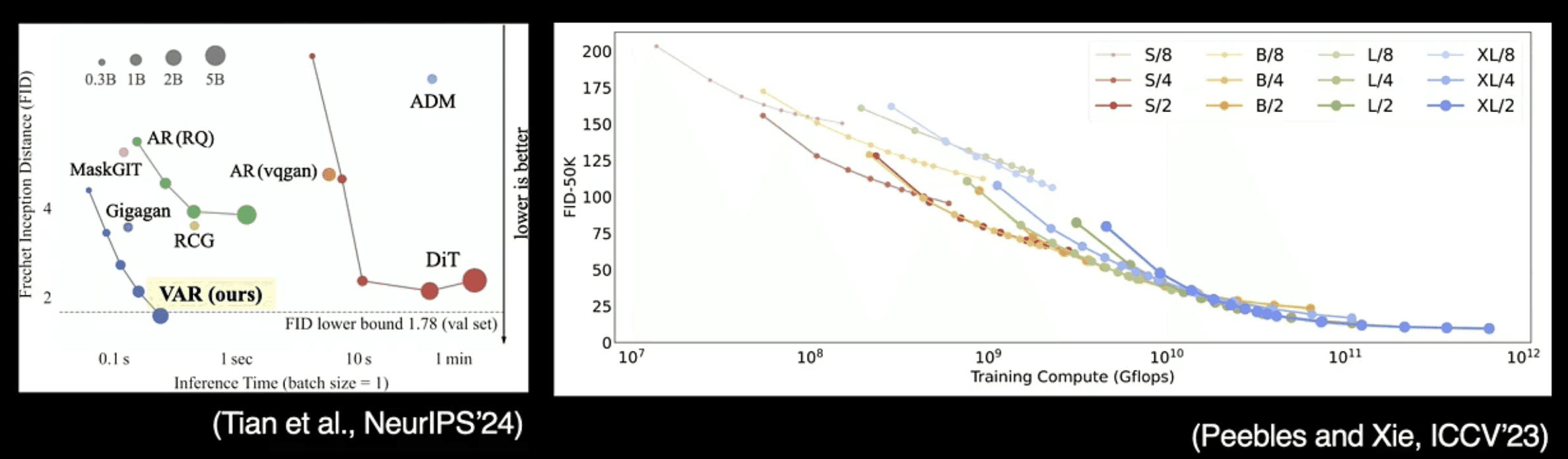
Lee detailed the importance of developing architectures that avoid relational inductive bias between data entities. The presentation included technical discussions of neural scaling laws, which provide an empirical framework for economic training with large compute resources. This approach enables more efficient utilization of computational resources while maintaining model performance.
4. Large-scale Compute Optimization
A critical technical component involves maximizing model FLOPS units (MFUs) during large-scale distributed training. Lee explained that MFU, defined as the ratio of observed throughput to theoretical maximum throughput, serves as a key metric for optimization. The approach encompasses various technical optimizations:
Implementation of low-precision computing techniques
Development of custom CUDA kernels for improved performance
Advanced parallelism strategies
Sophisticated cluster management systems
5. Cross-functional Team Integration
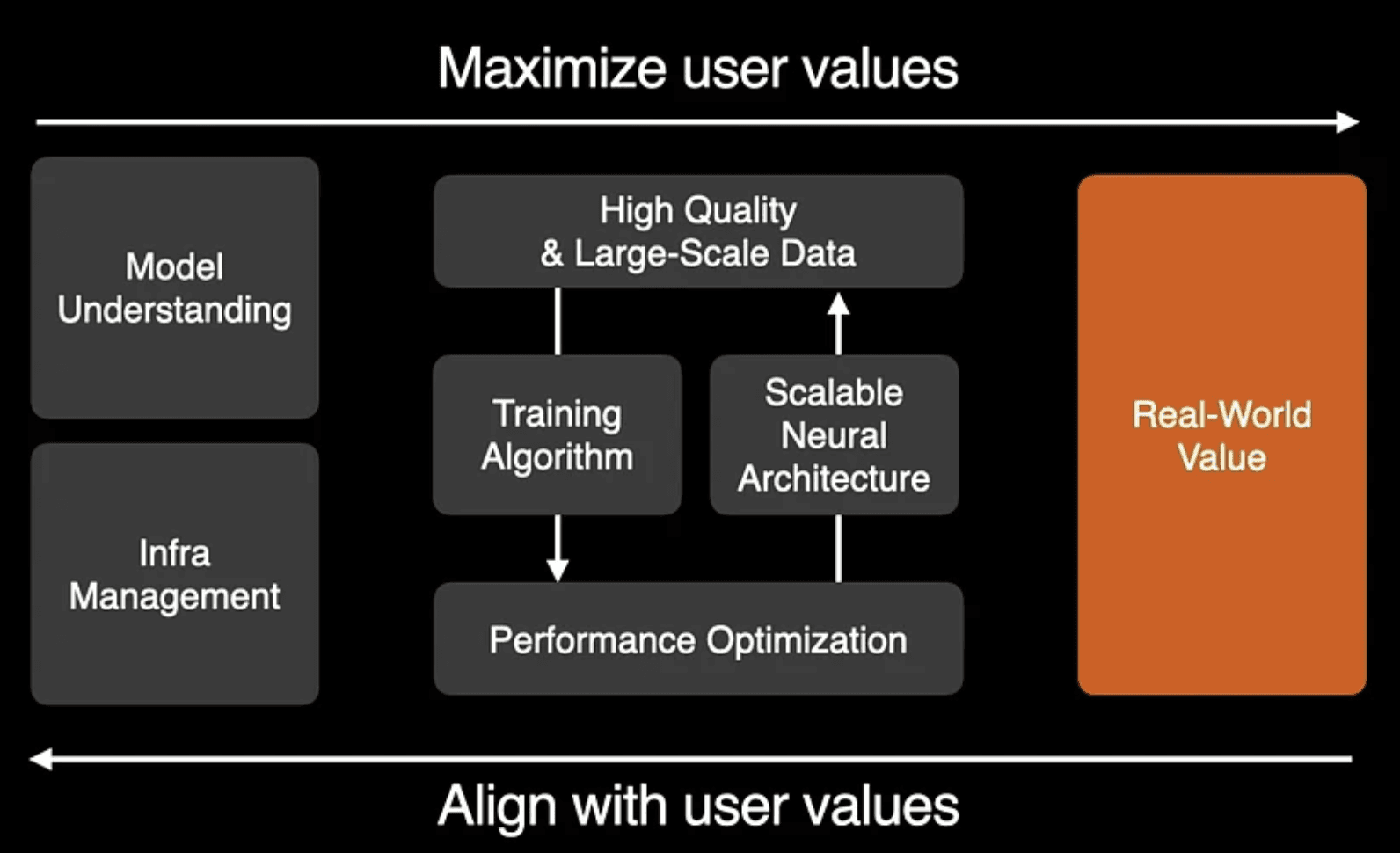
Lee emphasized that training large-scale models requires a systematic approach across functional teams. He presented a comprehensive framework for team collaboration, highlighting the importance of continuous feedback loops and cross-disciplinary expertise.
Runway's Advanced Video Generation Models
Gen-3 Alpha
Lee provided an in-depth look at Runway's Gen-3 Alpha model, which was released in June 2024. The model demonstrates remarkable capabilities in generating high-quality and diverse videos from natural language prompts. However, he acknowledged the ongoing challenge of fully understanding the model's ability to combine and generalize its world knowledge.
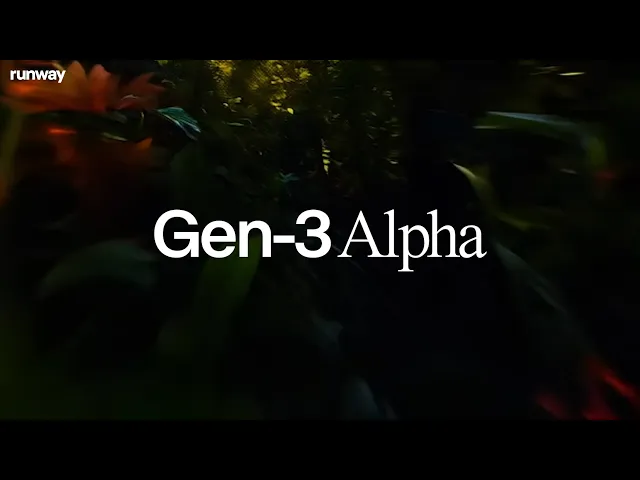
The Runway research team has conducted extensive research to understand the model's capabilities in several key areas:
Understanding and application of physical laws
Text rendering capabilities
Combinatorial generalization across visual concepts
Intuitive Control Mechanisms
Lee introduced several innovative approaches to controlling video generation:
Keyframe conditioning for precise stylistic control
Seamless integration with existing workflows through Generative VFX
Advanced camera control systems with 3D understanding
Fine-grained motion control through specialized brushes
Structural conditioning for video composition
Video out-painting capabilities for expanded creative control
Act-One: The Next Generation
The presentation included a demonstration of Act-One, representing the next evolution in video generation technology. This system builds upon the foundations of Gen-3 Alpha while incorporating more sophisticated control mechanisms and improved understanding of temporal dynamics.
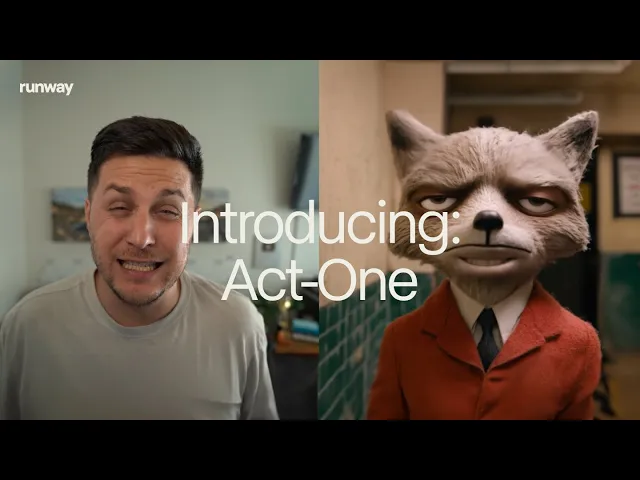
Future Directions and Challenges
Lee concluded by emphasizing that language and video serve as fundamental world descriptors: language providing the most abstract knowledge representation, and video offering the most scalable source of visual world representation. He stressed that scaling remains a crucial factor, with access to gigantic video datasets and the entire visual world for interaction.
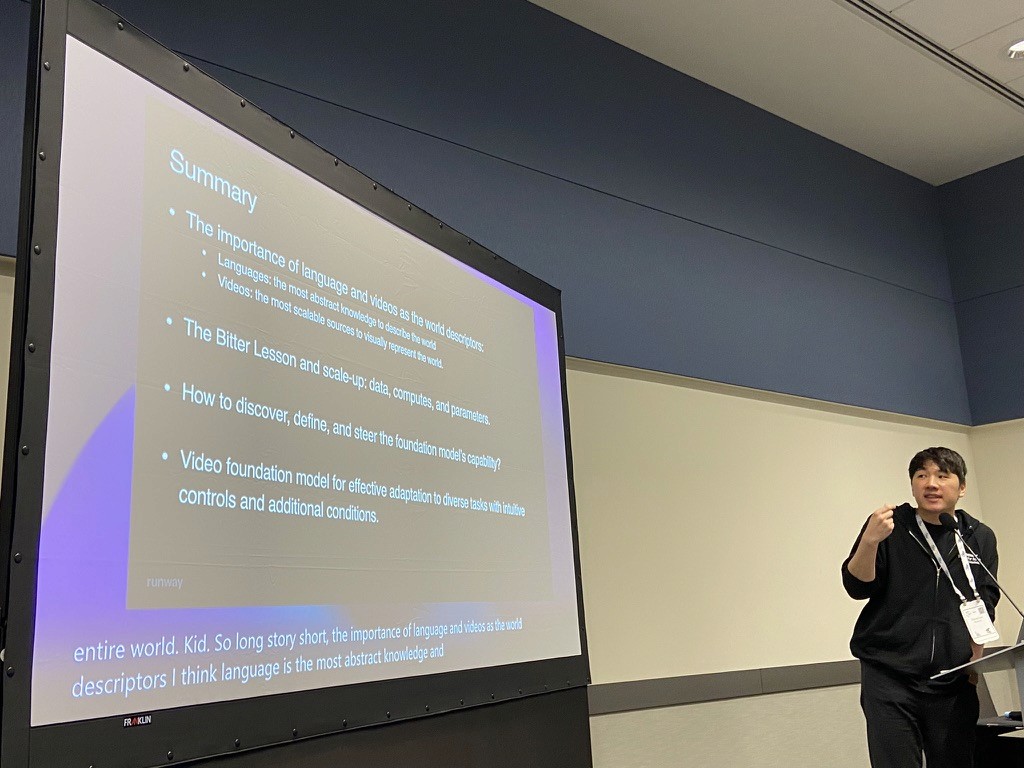
The presentation ended with a clear vision: the development of General World Models that understand the world at an unprecedented level. This goal requires continued innovation in:
Model architecture and scaling
Data collection and curation
Computational efficiency
Intuitive control mechanisms
Integration with existing creative workflows
The Future of Video-Language Models: A Vision Forward
The inaugural NeurIPS 2024 Video-Language Model Workshop has illuminated both the remarkable progress and compelling challenges in the field of video understanding. As an industry practitioner and organizer of this workshop, Twelve Labs recognizes the critical themes that emerged from our distinguished speakers' presentations.
Key Insights and Emerging Themes
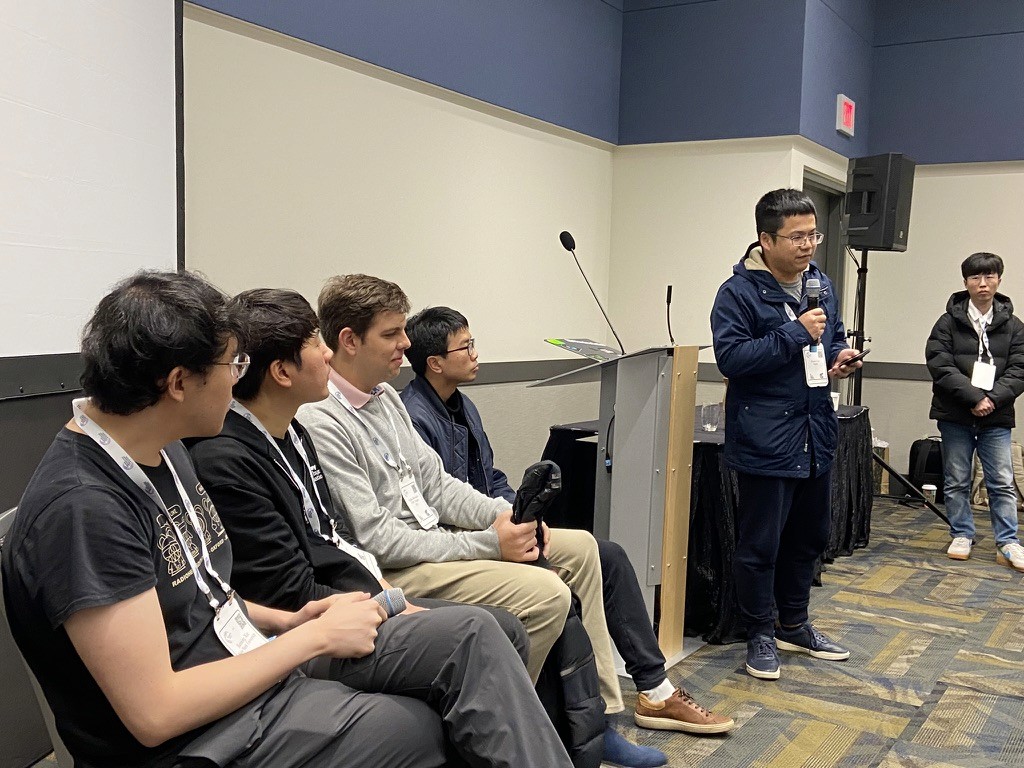
Several crucial themes resonated throughout the workshop. Professor Dima Damen highlighted the importance of understanding fine-grained temporal dynamics in egocentric videos, while Professor Gedas Bertasius emphasized the need to move beyond pure language-centric approaches in video understanding. Professor Yong Jae Lee's presentation revealed that even short-form video understanding remains challenging, particularly when dealing with counterfactual temporal information.
Jianwei Yang's vision of multimodal agentic models and Ishan Misra's insights into video generation demonstrated how the field is evolving beyond simple understanding tasks toward more sophisticated applications. Doyup Lee's exploration of general world models highlighted the potential for video-language models to develop comprehensive world understanding.
Industry Perspective and Path Forward
At Twelve Labs, these challenges resonate deeply with our experience developing video understanding technologies. Our journey with Marengo, our video embedding model, and Pegasus, our video-to-text model, has taught us firsthand about the complexities of multimodal integration, temporal understanding, and the critical importance of high-quality training data.
The workshop discussions validate our approach to tackling these challenges through:
Multi-vector embeddings that capture the multifaceted nature of video content
Sophisticated temporal modeling that addresses both short-term and long-term dependencies
Integration of multiple modalities including visual, audio, and textual information
Development of robust evaluation frameworks that go beyond traditional metrics
Vision for the Future
The future of video-language models is incredibly promising. As we move forward, we envision:
More sophisticated temporal understanding capabilities that can handle complex narratives and counterfactual reasoning
Better integration of world knowledge with visual understanding
More efficient architectures that can process longer videos while maintaining temporal coherence
Stronger bridges between academic research and industrial applications
This workshop marks an important milestone in bringing together researchers and practitioners to address these challenges collectively. As we continue to push the boundaries of what's possible in video understanding, we remain committed to fostering open collaboration and responsible development in this rapidly evolving field.
The path ahead is both challenging and exciting. By continuing to build on the insights shared at this workshop and maintaining strong collaboration between academia and industry, we can accelerate the development of more capable, efficient, and reliable video-language models that will transform how we perceive and interact with the world.
Related articles
© 2021
-
2025
TwelveLabs, Inc. All Rights Reserved
© 2021
-
2025
TwelveLabs, Inc. All Rights Reserved
© 2021
-
2025
TwelveLabs, Inc. All Rights Reserved